Marketo's Engagement Program A Deep Dive into Personalized Content Delivery Strategies in 2024
Marketo's Engagement Program A Deep Dive into Personalized Content Delivery Strategies in 2024 - Marketo's Engagement Program Structure and Functionality
Marketo's Engagement Programs utilize a framework built on "streams" to organize content. These streams are essentially pathways designed to deliver specific content to carefully defined audience groups. Rules govern which segments are funneled into particular streams. The delivery method, called "casts," ensures the right content reaches the right audience at the right time, all powered by pre-set cadences within each stream. Engagement Programs also leverage a Content Engagement Level, a score that helps gauge how well content is performing by tracking metrics such as opens, clicks, and unsubscribes. This score provides insights into how effective a program's content is and potentially indicates areas for improvement. These programs also boast automation features, triggering actions like emails or social media posts based on individual interactions. While the ability to segment and personalize is undoubtedly valuable, the true power of these programs hinges on the creation of truly engaging content. Understanding the preferences and characteristics of diverse audience segments is vital to effectively utilizing Marketo's Engagement features. In 2024, the success of using Marketo for lead nurturing will depend on how well organizations navigate the delicate balance between automation and fostering genuine connections with prospects through engaging content.
Marketo's Engagement Programs organize content into "streams," essentially creating categories that help focus content delivery. These streams aren't just about broad audience segments like demographics, but also leverage user behaviors to refine targeting. This means the program can tailor content based on how a person has interacted with past communications, not just their basic characteristics.
Rules are set up to decide which audience segments get assigned to which streams. This automated assignment is a key feature but potentially complex to configure properly. It would be interesting to investigate the finer details of how Marketo interprets and uses these rules.
Once the streams are populated with targeted audiences, content delivery happens via "casts." This concept is an interesting choice, raising questions about how robust and flexible the casting process actually is, especially in cases with rapid changes in audience behavior.
Each stream within a program operates on a schedule or "cadence" that defines the timing of content deliveries. Setting a cadence for each stream can be tricky, especially when dealing with diverse audience behaviors. It remains to be seen whether a cadence-based approach can truly adapt to modern audience expectations.
The program also has a Content Engagement Level metric, which scores content between 0 and 100 based on user interaction. The metric factors in things like opens, clicks, unsubscribes, and overall program success. This is a valuable way to gauge performance, though the exact way it combines various data points can likely be improved. Further research is needed to better understand how this metric truly reflects program health and value.
The success of an Engagement Program is very dependent on designing and delivering content that truly resonates with the audience. Understanding the unique traits and preferences of each segment is crucial, yet in practice, this might not always be straightforward. This aspect could benefit from further study, particularly in cases of highly diverse segments.
Engagement Programs rely on automation to execute actions like sending emails or posting on social media. These automated actions are triggered by user behavior and preferences. The automation capabilities are quite powerful but can potentially lead to unintended consequences if not carefully configured. Evaluating how well Marketo's automated actions can handle unexpected or edge cases would be helpful.
Marketo's Engagement and Nurture Programs are often used interchangeably because both focus on nurturing leads through a series of interactions. This overlaps functionality can be slightly confusing, and it may be useful to have a clearer conceptual separation of these terms, as the nuances may be important depending on the specific goals and metrics.
Within a Marketo instance, there are four program types, and Engagement Programs are considered a strong option for complex lead nurturing workflows. The variety of program types available can be beneficial in structuring complex campaigns, but might also introduce complexity for users when they try to determine the best structure for their needs.
Finally, Marketo has built-in mechanisms to assign 'credit' or 'attribution' of success based on lead interactions. This ability to track and credit engagements is valuable, but it is crucial to understand how success is defined and measured, as this can significantly impact campaign optimization. It’s also worth noting that depending on the complexity of the campaign, success attribution can become intricate and challenging to analyze and optimize.
Marketo's Engagement Program A Deep Dive into Personalized Content Delivery Strategies in 2024 - AI-Driven Dynamic Content Personalization in 2024
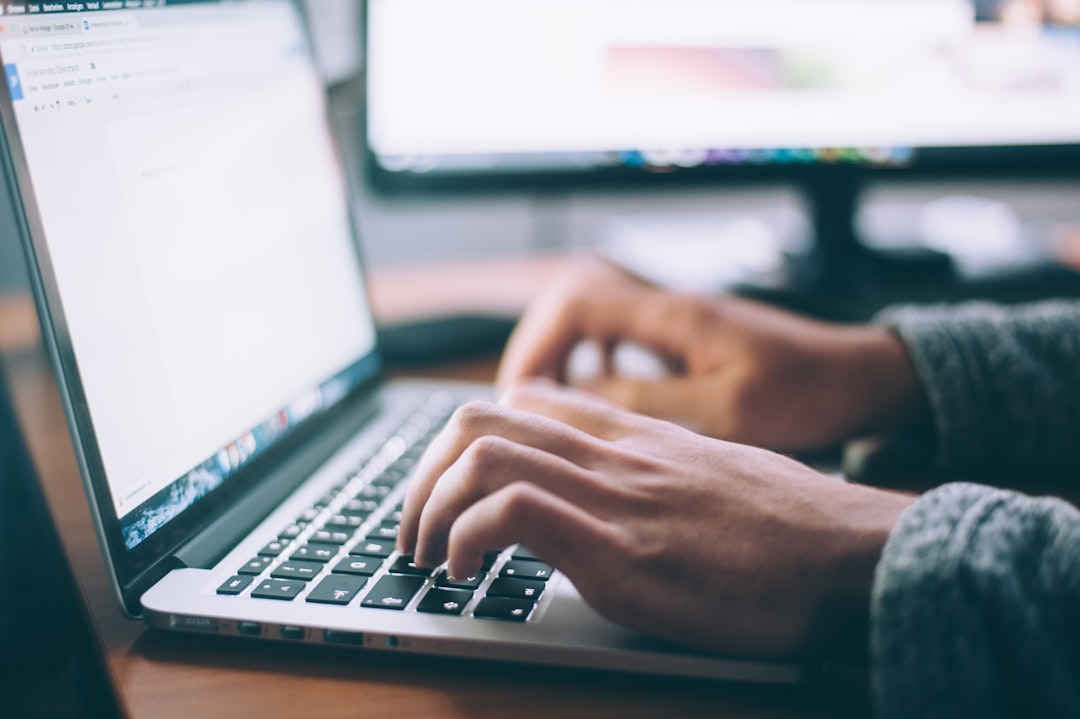
In the evolving landscape of marketing in 2024, AI-driven dynamic content personalization has emerged as a crucial strategy for businesses aiming to deepen customer engagement and cultivate lasting loyalty. This approach necessitates a robust data foundation that allows for comprehensive tracking and analysis of audience interactions, going beyond simple demographics to capture intricate behavioral patterns. AI systems, through advanced analytics and machine learning, are capable of delivering hyper-personalized experiences, moving beyond basic segmentation to cater to individual preferences and needs. This shift towards highly tailored content experiences, while powerful, also presents new challenges. Many organizations may find themselves lacking the necessary expertise in AI to manage and leverage these advanced personalization techniques effectively. This gap in knowledge may hinder the ability to truly harness the full potential of AI in delivering meaningful, customized content. The future of successful engagement will be tightly linked to the capacity to tailor content experiences and build a truly personalized user journey, a skill set that will become increasingly important as marketing trends continue to evolve.
The ability of AI to personalize content dynamically is becoming increasingly crucial in 2024, especially as it can now predict user intent with a remarkable degree of accuracy by examining past behavior. This capability allows marketers to deliver extremely relevant content, even before users themselves realize they need it. This is a fascinating development, though it's important to question how much truly novel insight these systems are providing, especially given the ever-present concerns about potential biases in the training data.
We're also seeing significant improvements in AI's ability to understand context through natural language processing. Now, AI-powered systems can craft content that is sensitive to things like time of day or local weather. Such details enhance user experience by creating a sense of immediacy and relevance that generic content can never achieve. However, there's a fine line here between relevant personalization and unwanted intrusiveness, something that researchers will need to keep a close eye on.
The effectiveness of this personalized approach is backed up by various studies, some of which show conversion rates rising by a substantial 200% when compared to traditional, non-personalized strategies. This emphasizes the growing importance of targeted messaging in digital marketing, but a healthy dose of skepticism is warranted. There's often a tendency to inflate the value of such claims, and it would be prudent to explore these claims more rigorously.
Historically, audience segmentation relied largely on demographic information. However, AI models are now incorporating psychographic factors, offering a much deeper understanding of user motivations. This shift is quite significant, offering valuable insights for marketing efforts, but the validity of inferred 'psychographics' needs careful consideration. The line between legitimate inference and potentially problematic stereotyping is still somewhat blurred.
Content delivery is increasingly automated and can now react in real-time to changes in user behavior. This adaptability allows for truly dynamic interactions, yet also introduces significant challenges for marketers. Keeping up with quickly changing audience preferences will require substantial investment in personnel and infrastructure. It remains to be seen whether organizations will be able to adapt quickly enough.
While this shift to AI-driven personalization has the potential to significantly reduce the time spent creating content, potentially freeing resources for more strategic projects, we're still in the early stages. The benefits seen in reducing content creation time by 50% may not be universally experienced and it's crucial to understand the contexts where such efficiencies are achieved.
Predictive analytics is playing a crucial role in identifying areas where content is lacking. By analyzing user engagement with existing content, AI can predict future needs and proactively create the necessary content. This shift towards proactive content development is exciting and offers a potential solution to long-standing challenges, but as with any predictions, the accuracy will depend heavily on the quality of the underlying data and the AI models.
New privacy regulations are adding another layer of complexity to personalized content delivery. Marketers are now exploring solutions based on anonymized data, trying to reconcile innovation with the growing concerns over user tracking and data protection. This highlights a crucial tension, but there is little evidence that anonymized data truly addresses concerns about data security and privacy. It will be critical to see how this space evolves and whether viable solutions can be implemented.
Machine learning algorithms are not only becoming more sophisticated at suggesting content based on past behaviors but are also learning from failed interactions. This capability allows for continuous improvement, driving the evolution of personalized content strategies. This is definitely a positive step, though there is the risk that continuous optimization may lead to unintended homogenization of content, potentially limiting diversity and creativity.
The challenge of maintaining a balance between automation and genuine human connection remains paramount. Over-reliance on automated systems runs the risk of alienating users. It's crucial to maintain a sense of human touch, even in an increasingly automated landscape. While it is understandable that many marketers see automation as a path to significant efficiency gains, it is important to consider the ethical dimensions of designing and implementing these systems in the future.
Marketo's Engagement Program A Deep Dive into Personalized Content Delivery Strategies in 2024 - Real-Time Testing and Optimization Strategies
Within Marketo's Engagement Programs, and indeed within the broader marketing landscape of 2024, real-time testing and optimization are no longer optional but rather fundamental to success. The ability to swiftly adjust content and messaging based on how individuals are actually interacting with the materials is critical to maintaining high levels of audience engagement. This necessitates a methodical approach to testing, where marketers isolate single variables to see their specific effects. The goal is to gain clear insights that can then be used to refine the program's overall effectiveness. This process is greatly enhanced by AI-powered analytics, which allow for even more granular personalization based on real-time behavioral data.
However, while these strategies are incredibly powerful, they do present a new set of challenges. Rapid adaptation requires constant attention and can introduce complexity that some organizations might struggle to manage. Marketers need to be prepared for this level of dynamism and be willing to dedicate the resources necessary to make it work effectively. Ultimately, the ability to rapidly adjust and optimize is vital for achieving the desired results in today's fast-paced marketing environment. The question then becomes: how do organizations navigate the complexity of real-time adaptation while retaining the ability to create genuinely engaging and meaningful connections with their audience?
Real-time testing and optimization are becoming increasingly important in today's marketing landscape, especially as we see AI and machine learning play a larger role in delivering personalized experiences. It's fascinating to see how these technologies can learn and adapt based not only on user interactions but also on external factors like market trends and seasonal patterns. This broader context helps enhance the relevance of the content delivered, moving beyond simple behavioral signals.
Companies that implement real-time testing have reported seeing changes in engagement within just a few hours of optimizing their content or strategies. This rapid feedback loop is incredibly valuable because it allows marketers to quickly adjust their approaches based on real-time user reactions, making adjustments almost immediately. This differs from the slower, more traditional A/B testing where you might only test a couple of variables at a time. Now we're seeing multi-variate testing, where multiple elements are tested concurrently. While it adds complexity, it can lead to richer insights about audience preferences and create more tailored optimization strategies.
It's interesting to note the rising influence of neural networks in this realm. These networks aren't just a trend; they're actually enhancing the predictive modeling capabilities of these systems. It allows the systems to anticipate user needs with greater accuracy than with older, conventional methods. Some research suggests that this can lead to engagement improvements of over 30%.
The increased precision of segmentation through real-time optimization is also notable. Instead of relying on broad segments like demographics, marketers can now focus on 'micro-segmentation,' where content is tailored to much smaller, niche groups based on very specific behavior. It's likely this refined level of targeting leads to significantly higher conversion rates compared to more generalized segmentation approaches.
The beauty of real-time testing is the constant feedback loop it creates. Every interaction with a piece of content directly informs how that content evolves over time. This organic learning process can lead to extremely refined strategies that resonate much more deeply with the target audience. It's not surprising that organizations embracing this approach often choose to reallocate resources previously dedicated to manual outreach efforts towards more strategic planning and creative content development. This can lead to greater campaign efficiency while maintaining or even reducing operating costs.
However, as we push towards more personalized experiences using these technologies, we need to be mindful of the ethical implications, particularly regarding privacy and consent. With the granular data we can collect and analyze with these tools, there's a heightened risk of crossing privacy lines. It's a balancing act that will require continuous monitoring and evaluation.
Beyond simply tracking clicks and opens, the field of performance metrics is expanding to consider user sentiment and interaction depth. This creates a more comprehensive view of how effectively content is actually performing. Even with this improved understanding, there's still significant resistance to embracing these real-time optimization strategies in some organizations. This resistance often stems from organizational inertia and a reluctance to change established processes, even when presented with data that clearly shows the potential for improvement. It is a fascinating dynamic, and it will be interesting to see how it plays out in the coming years.
Marketo's Engagement Program A Deep Dive into Personalized Content Delivery Strategies in 2024 - Content Engagement Level Scoring Explained
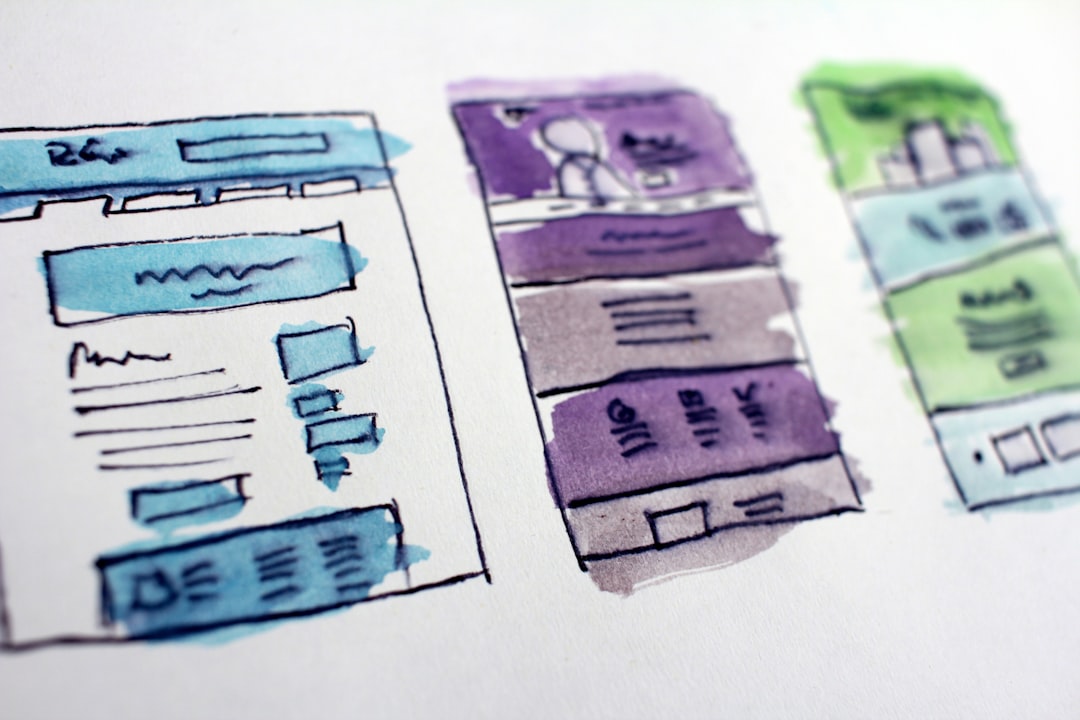
Within Marketo's Engagement Programs, understanding how the Content Engagement Level Scoring system works is crucial for improving your personalized content strategy in 2024. This score, which falls between 0 and 100, offers a snapshot of how well your content is performing. It does this by examining both positive interactions like clicks and email opens, as well as negative signals, such as unsubscribes. The score, which is calculated using a proprietary algorithm, attempts to gauge the effectiveness of content within a given stream, but it's worth considering the limits of this automated approach in capturing the true essence of engagement. The challenge remains to balance automated scoring with the nuances of human interaction. As we continue to utilize these programs, it becomes more evident that we need to shift our focus from just counting interactions to truly understanding their quality and meaning. By skillfully employing the insights gleaned from this scoring system, marketers have the potential to refine their strategies and foster a much deeper connection with their audience.
The Content Engagement Level Score, ranging from 0 to 100 within Marketo's Engagement Programs, isn't just a simple tally of interactions. It's a dynamic score that blends various interaction data like email opens, clicks, and form submissions, along with disengaged actions like unsubscribes. How these factors are weighted can significantly alter the final score, making a deeper analysis crucial for practical insights. It's intriguing how the score could be tweaked to emphasize specific behaviors, but understanding the potential impact of these changes is important.
Research suggests a strong link between engagement and faster sales cycles. One study showed a 60% acceleration in the sales funnel for highly engaged leads, raising questions about how well organizations are utilizing this scoring system to optimize their processes. If there is such a strong relationship, there may be overlooked benefits to be gained by fine-tuning engagement scores.
We can potentially use the Content Engagement Level score for predictive analysis, attempting to forecast which content might resonate with specific audience segments in the future. However, the accuracy of these predictions depends heavily on the quality and depth of past engagement data. It makes one wonder if our data is sufficient to reliably predict engagement, especially given the rapidly evolving nature of user behaviors.
Some research suggests that engagement-focused content might increase brand loyalty by up to 50%. While the connection is captivating, it's worth being cautious about whether this type of content can truly foster deep trust. There's a potential that while metrics go up, true human-centric relationships remain important for enduring loyalty.
Focusing too heavily on engagement scores can incentivize organizations to produce clickbait-style content rather than content with inherent value. While this might temporarily inflate engagement metrics, it could ultimately harm brand reputation and erode user trust. It raises the interesting point of whether short-term gains in engagement metrics are worth potential long-term damage to a brand's reputation.
Many tools that measure the Content Engagement Level use machine learning to adapt in real-time. These algorithms can effectively synthesize user interaction data across diverse demographics, creating a kind of "collective intelligence." But, if not thoughtfully managed, biases present in the data can skew the output of these algorithms. There is a risk of creating a 'filter bubble' and promoting a specific view, which should be considered when evaluating these systems.
The accuracy of engagement scoring tends to drop when trying to assess a very diverse audience. With significantly different interests and behaviors across a user base, a single score might struggle to accurately reflect the concept of engagement across different segments. This indicates a potential need for more customized or nuanced scoring models to capture this inherent variety of user engagement behaviors.
There's a trend where organizations emphasizing engagement scores see conversion rates rising between 20% and 50%. However, it's worth asking if this increase is sustainable in the long term or just a temporary effect driven by novelty. It would be interesting to track these conversion rates over time to better understand the actual impact on long-term customer engagement.
One striking pattern is the strong correlation between engagement and content relevance. Content that directly addresses the specific needs of an audience segment frequently outperforms generic content. Creating this type of targeted content requires a nuanced understanding of the audience, a challenge many organizations are still grappling with. The quality of the data available to guide content creation is a limiting factor here.
Companies with robust content engagement metrics often see lower churn rates, with some reporting a 30% reduction. This raises important questions about how traditional retention strategies compare to a focused engagement framework. Are retention programs effectively addressing the same needs that engagement programs are, or are there fundamentally different approaches at play? This is worth further investigation.
Marketo's Engagement Program A Deep Dive into Personalized Content Delivery Strategies in 2024 - Integration of Sales Insights for Lead Prioritization
Within the evolving world of personalized content strategies, incorporating sales insights into lead prioritization is becoming increasingly important. Sales teams can now access buyer engagement data directly within their CRM systems, which enables them to make better choices about which leads to pursue. This allows them to initiate conversations at the optimal moment based on recent interactions with marketing materials. Predictive analytics tools are transforming how leads are ranked, giving businesses a much better chance of finding leads with a high probability of becoming customers. Combining marketing and sales data creates a complete picture of each prospect, improving the overall strategy for engaging leads and moving away from older, less refined methods. The foundation for this modern approach relies on high-quality data that reveals what potential customers are truly interested in. While these improvements offer valuable advantages, the challenge lies in skillfully integrating and applying this information to enhance the sales process while retaining a genuine human connection with potential customers.
In the realm of marketing and sales, lead prioritization has evolved from rudimentary methods to sophisticated systems that leverage a wealth of data. Integrating sales insights into this process offers a potent avenue to enhance lead nurturing and guide sales interactions. One of the more significant developments is the integration of sales engagement data directly into CRM systems. Sales teams, armed with this information, gain the capacity to prioritize leads based on recent customer interactions, enabling them to initiate timely and relevant conversations. This integration not only enhances sales productivity but also allows marketers to better tailor messages to individual needs and preferences.
The concept of "Sales Insight Actions" highlights the synergy between sales and marketing. By embedding marketing insights into sales workflows, companies can optimize both inbound and outbound sales processes. For instance, a sales representative can see the specific content a prospect interacted with, enabling them to tailor their initial contact to a prospect's known interests. This level of personalization can significantly increase the likelihood of engagement. Furthermore, having unified access to sales and marketing activity within both Marketo and the CRM system provides a consolidated view of the customer journey. This complete picture improves a company's understanding of their customers and can facilitate more effective personalization efforts across various teams, from sales and marketing to customer service.
Traditionally, lead prioritization has largely relied on basic metrics, like demographics or lead source. However, the landscape has shifted, with a growing focus on sophisticated intent data. This intent data includes things like website behavior, content downloads, and engagement with marketing campaigns. Predictive analytics has emerged as a powerful tool in this shift. Utilizing machine learning and AI, predictive lead scoring analyzes various data points—interactions, demographics, and other signals—to identify leads with the highest probability of conversion. This ability to identify high-potential leads can significantly optimize sales efforts.
The increasing need for a holistic view of leads drives the expectation that more data sources will be integrated in the future. This could include social media interactions, customer support logs, and even external market data. This multi-faceted approach has the potential to refine lead prioritization methods and create a richer understanding of customer behavior. However, it also brings significant challenges, particularly around data privacy and security. Organizations need to be mindful of how they collect and utilize this data to maintain customer trust.
While the prospect of leveraging AI and enriched data to build hyper-personalized marketing and sales strategies is appealing, it also raises some significant questions. For example, will relying too heavily on AI-driven models lead to a homogenization of marketing strategies? It's conceivable that overly personalized approaches, while initially engaging, might inadvertently lead to a reduction in creative and innovative marketing approaches. Furthermore, are these AI systems accurately interpreting user intent, or do inherent biases in the training data skew the results? Further investigation is needed to assess the reliability of the predictions derived from these systems and determine if these are simply replicating existing biases or generating truly novel insights.
Another potential concern with integrating various data sources is the possibility of data silos. If marketing and sales teams are not collaborating effectively and sharing data openly, the benefits of a holistic approach are lost. To truly benefit from integrating sales insights, it's crucial to break down these data silos and encourage cross-functional collaboration to achieve a unified understanding of customer behavior. This seamless integration across teams is essential to create a truly personalized and effective customer journey, a crucial goal in 2024.
While the prospect of AI-driven lead prioritization is promising, there are complexities and potential challenges that need careful consideration. As we continue to refine these methods, it's crucial to understand the inherent biases of these systems, the potential for over-reliance on automation, and the necessity of prioritizing human connection alongside data-driven insights. This nuanced approach will be essential in ensuring that AI-driven strategies truly empower marketers and sales teams to build genuine connections with their customers, ultimately maximizing marketing and sales ROI.
Marketo's Engagement Program A Deep Dive into Personalized Content Delivery Strategies in 2024 - Measuring ROI of Personalized Content Delivery
Determining the return on investment (ROI) of delivering personalized content is vital for optimizing marketing efforts and boosting customer engagement. Personalized content has the potential to substantially increase user interaction, which in turn, can elevate conversion rates and foster enduring brand loyalty. Metrics like direct sales and user satisfaction can help us understand how well content strategies are working, while engagement data, such as bounce rates and click-through rates, provides a more detailed picture of how users react to targeted messaging.
However, crafting clear, measurable goals that align with overarching marketing objectives can be tricky. It's important to remember that engagement isn't always easily translated to direct revenue. Defining those connections between specific content and quantifiable outcomes is often complex. As businesses continue to refine their approaches to content, understanding and using ROI measurement tools is critical for successfully navigating the ever-changing world of personalized marketing. The ability to connect content performance to business outcomes will likely become increasingly important in the coming years.
Observing the effectiveness of personalized content delivery requires a multifaceted approach, going beyond simple interaction counts. While overall content marketing ROI has been impressive historically, ranging from several hundred to over a thousand percent, achieving similar results with personalized strategies demands careful measurement.
For instance, focusing on improving content relevance, even by a small margin, can significantly impact conversion rates. Research suggests a mere 10% improvement in content relevance can lead to a 50% increase in conversions. This highlights the value of prioritizing quality personalized interactions over sheer volume.
Similarly, refining audience segmentation by moving from broad demographic categories to highly specific behavioral groups can boost engagement. Studies show that a more nuanced segmentation strategy can result in a 20% to 30% increase in engagement rates, making micro-targeting an important consideration.
The speed at which we can now adapt content is also noteworthy. Real-time testing enables organizations to gain insights almost instantaneously, with some organizations reporting a 40% increase in engagement following relatively minor content adjustments. This rapid feedback loop underscores the need for marketers to be highly responsive to evolving audience preferences in today's fast-paced environment.
AI is rapidly changing how we understand and predict user behavior. Advanced algorithms can now predict user interactions with impressive accuracy, often reaching an 85% success rate in anticipating content needs. This allows for preemptive content delivery, potentially engaging users before they even know what they're looking for. However, this capability also raises questions about potential biases within the training data that might skew predictions.
Marketo's Content Engagement Level scoring system is a useful tool for evaluating performance, but it can be tricky to interpret. This scoring system combines positive and negative user interactions—like opens, clicks, and unsubscribes—to generate a score that represents engagement. The way the algorithm weights these interactions can significantly impact the resulting score, so it's important to critically evaluate how the score is calculated and its true meaning within a specific marketing context.
Interestingly, while initial increases in engagement metrics are often observed, there can be a natural decline over time. This suggests that some engagement tactics, especially those that rely heavily on short-term, clickbait-style content, might lead to unsustainable growth. Evidence suggests that retention rates can decrease by as much as 30% when engagement efforts rely primarily on such strategies. This reinforces the need to focus on building longer-term engagement through valuable and relevant content.
The reliance on machine learning algorithms for engagement scoring brings its own set of challenges. While powerful, these algorithms are susceptible to biases present in the training data. If not carefully addressed, these biases can lead to misleading insights and potentially inaccurate predictions. This makes careful data management critical for avoiding skewed interpretations that could misguide a marketing strategy.
However, by expanding the range of engagement metrics beyond basic interactions to include elements like sentiment analysis, organizations can gain a more complete picture of user behavior. Studies indicate that companies focusing on a multi-dimensional understanding of engagement see a 25% improvement in customer satisfaction scores, highlighting the importance of truly grasping the depth and quality of user interactions.
Bridging the gap between sales and marketing by incorporating sales insights into marketing strategies can greatly enhance lead conversion rates. Organizations that successfully integrate these insights have seen conversion rates improve by up to 70%. This seamless flow of data provides a distinct advantage but requires careful attention to potential data silos within an organization.
Finally, the ethical dimensions of personalized content delivery are becoming increasingly relevant. Concerns about data privacy are rising, and overly aggressive personalization strategies can potentially erode user trust. It's vital that organizations balance the pursuit of innovative approaches with ethical considerations to ensure that personalized content delivery respects user privacy while maintaining strong engagement over the long-term.
More Posts from :