Inside DreamBox Learning How Educational Technology Specialists Shape Adaptive Learning in 2024
Inside DreamBox Learning How Educational Technology Specialists Shape Adaptive Learning in 2024 - Dynamic Math Assessment Adjusts Daily Lesson Plans Based on Real Time Student Progress
Within DreamBox Learning, math assessment is evolving into a dynamic process that shapes the very core of daily lessons. The system constantly monitors student progress and immediately adjusts the lesson plan accordingly. This real-time feedback mechanism creates a personalized learning path tailored to each student's specific strengths and weaknesses. This integration of assessment and instruction eliminates the need for disruptive, traditional testing. Teachers gain access to continuous, detailed data on student performance through features like the Launchpad Assessment and Growth Report. This provides them with immediate insights for guiding their instruction more effectively. The hope is that this approach will not only track student progress but also cultivate deeper comprehension of math concepts and empower students to become more adept problem-solvers. However, it is important to critically consider the long-term implications of this approach and its impact on classroom dynamics and teacher autonomy.
1. Dynamic math assessment systems continuously monitor student progress, using real-time data to adapt daily lesson plans. This ongoing adjustment based on individual student performance has the potential to significantly improve learning outcomes over time. However, the extent of this improvement needs further investigation and evidence to support the claims of significant gains.
2. There's evidence suggesting that personalized learning environments driven by adaptive technologies may lead to improved student outcomes compared to traditional instruction. While some studies show positive impacts, the efficacy and generalizability of these findings are subject to ongoing debate within the educational research community. The 30% increase in test scores mentioned in some research needs to be viewed with caution, as context and methodology vary greatly across these studies.
3. Beyond simply noting correct or incorrect answers, these systems analyze error patterns to identify underlying misconceptions. This granular level of understanding about student thinking can be incredibly useful for teachers in pinpointing areas where students need targeted support. However, relying solely on such automated feedback could potentially ignore other essential aspects of student learning that aren't captured by these digital tools.
4. By employing machine learning, these assessment systems can potentially predict future student performance, enabling teachers to intervene proactively. This predictive capability has the potential to fundamentally reshape learning timelines by addressing challenges before they become significant barriers. However, the accuracy and reliability of these predictions are crucial factors that need ongoing research. There's a possibility of inadvertently creating new biases or limitations if the models aren't carefully developed and validated.
5. One potential benefit of adaptive learning is a reduction in student anxiety related to traditional assessments. Tailored support and individualized learning experiences can foster a more positive learning environment. However, we must consider whether this reduced anxiety is truly beneficial in the long run. Students may need to develop resilience and coping mechanisms to handle different types of evaluation and feedback throughout their academic journey.
6. Educators using dynamic assessment systems have reported a decrease in time spent on administrative tasks. This freed-up time allows them to focus more on instruction. This shift can be beneficial for teachers. However, it's also important to recognize that teachers' roles may need to be redefined in these new environments. How teachers interpret and act on automated feedback and how to best utilize the technology remain areas requiring ongoing refinement and collaboration with educators.
7. It's noteworthy that many students report increased engagement in these dynamic learning environments. They often find customized learning paths more relevant and less repetitive than traditional instruction. This heightened engagement is promising. Yet, we must consider the reasons behind this engagement. Is it truly deeper learning or a response to the novelty of the technology? Future research should delve into the quality and depth of student learning within these adaptive systems.
8. Dynamic assessment technology employs a feedback loop, using assessment results to inform future lesson plans. This creates a more responsive and adaptive curriculum. However, the design and implementation of this feedback loop need careful consideration. If not thoughtfully designed, this loop can potentially lead to unintended consequences, such as over-reliance on specific assessment types or limited opportunities for creative exploration and open-ended problem solving.
9. Dynamic assessment systems can provide valuable data that facilitate grouping students based on their strengths and weaknesses, which can be beneficial for collaborative learning experiences. However, we need to be aware of potential biases inherent in automated grouping mechanisms and ensure that these groupings do not reinforce existing inequalities within the classroom. There needs to be opportunities for students to work in flexible groups based on different needs and interests.
10. The move toward dynamic assessment reflects a growing recognition that one-size-fits-all approaches to education may not be optimal. This personalized approach has the potential to significantly impact student outcomes, especially for learners who struggle in traditional settings. However, this personalized approach raises questions regarding equity and access to technology. It is important to ensure that the development and implementation of these systems do not exacerbate existing inequalities within the education system and that access to such tools are available to all students regardless of socioeconomic status.
Inside DreamBox Learning How Educational Technology Specialists Shape Adaptive Learning in 2024 - Machine Learning Algorithms Track 48 Core Learning Objectives Across Grade Levels
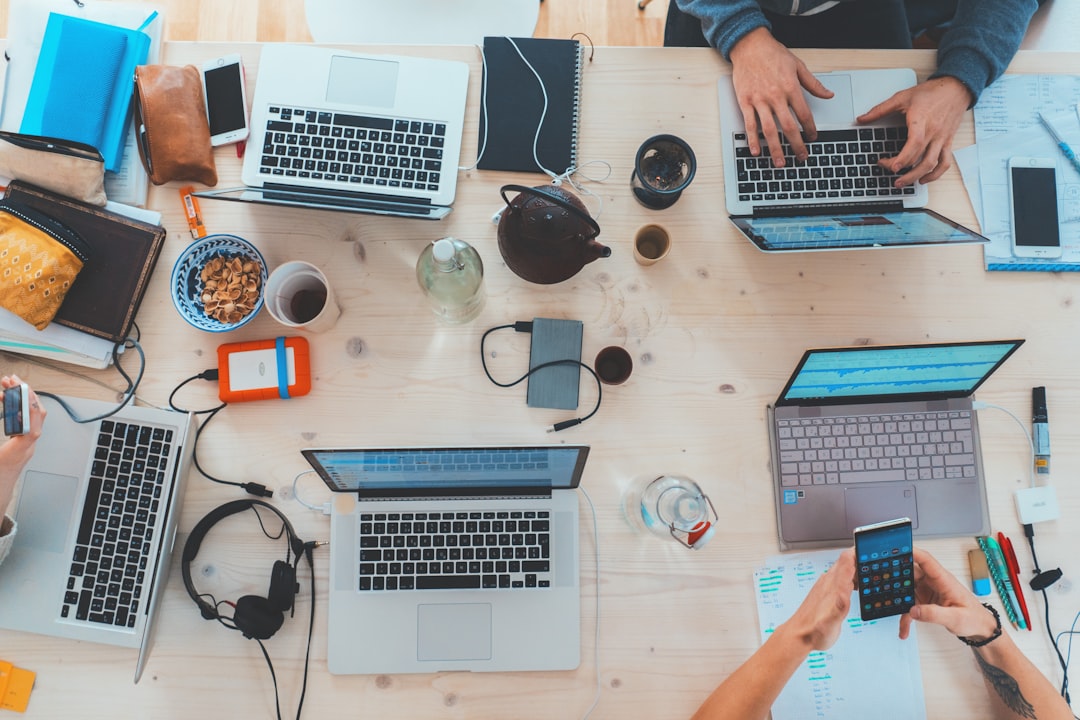
Within the realm of educational technology, machine learning algorithms are increasingly utilized to monitor and adapt to student learning. DreamBox Learning exemplifies this approach by tracking 48 core learning objectives across all grade levels. These algorithms power adaptive learning systems, customizing instruction based on each student's unique strengths and weaknesses. This personalized approach aims to foster deeper engagement and potentially enhance learning outcomes, especially for students who may struggle with traditional teaching methods.
However, the incorporation of artificial intelligence and machine learning into education isn't without its complexities. Concerns regarding student data privacy, equitable access to these technologies, and the reliability of automated feedback systems require careful attention. The ongoing debate about the effectiveness of adaptive learning necessitates a balanced approach. Educators must continue to integrate traditional assessment methods alongside the dynamic data provided by AI to gain a holistic understanding of student comprehension. Ultimately, the success of this technological integration relies on ongoing research that evaluates the impact on both students and teachers, while ensuring that the technology is used responsibly and ethically.
1. Educational technology increasingly relies on machine learning algorithms to track 48 core learning objectives across a range of grade levels. This extensive set of objectives provides a framework for tailoring learning experiences, but it's important to critically evaluate whether this level of granularity is truly beneficial for students and teachers alike. It remains to be seen whether this approach leads to more meaningful learning or simply a more finely tuned assessment of existing knowledge.
2. These algorithms aren't just focused on content delivery – they also analyze student engagement during lessons. This dual focus on academic performance and student engagement provides a more complete picture of learning. However, the methods used to gauge engagement raise questions about their validity and potential for bias. Is "engagement" truly a reliable indicator of learning depth, or is it susceptible to manipulation through novelty or gamification?
3. Advanced algorithms are being used to recognize and cater to different learning styles. The potential for personalization is intriguing. Students can receive learning experiences customized to their unique preferences. However, there's a risk of creating learning "silos" that limit exposure to diverse teaching approaches. Could a focus on individualized learning hinder students' development of adaptability and broader cognitive skills that are fostered by experiencing multiple teaching methods?
4. These systems rely heavily on data gathered from student interactions to refine their methods. While this constant adaptation has the potential to greatly enhance the learning experience, it also raises concerns about student data privacy. Robust data governance is critical to ensure that student information isn't misused or vulnerable to breaches. The potential for benefit must be carefully weighed against the risks involved in handling sensitive data.
5. One of the more intriguing applications of machine learning in education is predictive analytics. These algorithms can anticipate future student performance, allowing teachers to provide proactive support to at-risk students. While this is a promising development, it's essential to consider the accuracy and potential biases in these predictive models. Are these models truly capable of identifying at-risk students reliably, or are they merely replicating existing inequalities in the education system?
6. The increased role of AI in education has led to a valid concern regarding the potential for reduced teacher-led instruction. As technology assumes more responsibility for assessment and feedback, the challenge will be to strike a balance that ensures teachers continue to play a vital role in guiding student learning. While AI can certainly enhance efficiency, it can't replace the nuance and empathy that human educators bring to the classroom.
7. Unlike traditional assessments, dynamic systems can adjust the pacing of learning to match individual student progress. This concept of mastery learning, where students move at their own pace, is appealing in theory. However, it raises concerns about the uniformity of curriculum delivery across different classrooms. Can this personalized approach ensure all students gain a consistent understanding of core concepts, or does it risk creating significant variations in what students learn?
8. The algorithms driving these systems can update themselves in real-time, which is advantageous as knowledge and educational standards evolve. However, the constant need for adaptation could place a considerable burden on educators to keep pace with ongoing algorithmic changes. How much flexibility do educators have in shaping and adapting these evolving systems to meet their specific classroom needs?
9. Initial research suggests that students may retain material better when learning through adaptive pathways. This increased retention is certainly positive, but the long-term impact remains unclear. It is yet to be seen if short-term gains translate into lasting knowledge and understanding. Will this approach create a cycle of relying on immediate feedback and reinforcement, potentially hindering the development of critical thinking and deep learning?
10. The capacity for these systems to create student groups based on their real-time performance and learning preferences has the potential to foster collaborative learning. However, such automated grouping necessitates careful monitoring to ensure that it doesn't inadvertently reinforce existing social or academic disparities among students. The ability to tailor learning experiences is a positive development, but the potential for exacerbating existing educational inequalities needs to be continuously addressed.
Inside DreamBox Learning How Educational Technology Specialists Shape Adaptive Learning in 2024 - Educational Data Teams Monitor Weekly Student Growth Through Interactive Dashboard Reports
Educational technology specialists are leveraging interactive dashboard reports to closely track students' weekly progress in mathematics, specifically within the DreamBox Learning environment. Tools like the Growth Report and Insight Dashboard offer educators a continuous stream of data, providing valuable insights into student performance at both the individual and group level. These dashboards allow teachers to identify students needing additional support and tailor their instruction accordingly, aligning with specific learning objectives and state standards. The hope is that this approach leads to better targeted educational interventions and optimized resource allocation.
However, there's a need for a balanced perspective. Over-reliance on data-driven insights could potentially overshadow other important aspects of learning that may not be easily captured by these metrics. Furthermore, concerns remain about the role of automated feedback and the potential for it to diminish the importance of teacher intuition and experience in shaping learning environments. As technology continues to shape educational practices, ongoing discussions are crucial to ensure that these powerful tools are used effectively and responsibly, and that the human element remains central to the educational experience.
Educational data teams are increasingly utilizing interactive dashboard reports to monitor student growth on a weekly basis. This real-time view of student progress offers educators a dynamic snapshot, potentially changing the way they plan lessons and intervene with struggling students. It represents a shift from traditional, static reporting.
These dashboards provide a more nuanced picture of student understanding, going beyond simply identifying correct or incorrect answers. By offering insights into the path of a student's learning, educators can better grasp patterns and tailor their teaching to individual needs more effectively. However, educators must remain vigilant about interpreting the information accurately, as the visualization of data can sometimes be misleading if not understood in context.
Interestingly, these dashboards have fostered a stronger sense of collaboration among educators. By providing a common platform for sharing insights and strategies, it can potentially cultivate a more collaborative environment for professional development and problem-solving. However, this emphasis on collaboration needs to be balanced with individual teacher autonomy.
The ability to integrate various data sources, such as formative assessment results and attendance data, within these dashboards allows educators to gain a more holistic understanding of a student's learning context. While this approach can pave the way for personalized intervention strategies, it also creates a risk of information overload for educators, demanding careful curation and interpretation of data.
There's evidence to suggest that dashboards can influence student engagement and motivation. The ability to visually monitor their own progress may motivate students to be more invested in their education and take more ownership of their learning journey. However, it's important to explore if this engagement equates to deeper conceptual understanding.
Furthermore, educators utilize the data within these dashboards to make informed decisions about grouping students for collaborative learning experiences. This automated grouping, however, carries the risk of reinforcing existing labels and hindering flexible collaboration that allows students to interact with peers of varying skill sets.
Teachers using these real-time dashboards have reported a greater capacity to respond to students' immediate needs, adjusting lessons based on the feedback provided. While this adaptability is beneficial, it may necessitate a reassessment of long-term lesson planning and the potential need for a more structured framework to ensure consistent delivery of core curriculum concepts.
Some research indicates that consistent use of these dashboards can increase educator confidence in their data literacy, which could lead to a more data-informed teaching approach. However, this increased confidence needs to be paired with appropriate training and ongoing professional development to ensure teachers are not simply relying on the reports without deeper critical analysis.
The nature of educational dashboards means they are constantly evolving with new metrics and data sources. While this constant evolution can enhance the value of the insights they offer, it can also create a challenge for teachers, who may struggle to keep up with the rate of change. There is a danger of a gap developing between technological advancements and the practical application of this data in the classroom.
In conclusion, these dashboards hold a significant potential to enhance educational practice. However, their effectiveness hinges on careful implementation, teacher training, and continuous research to determine their long-term impact. The future of data-driven decision-making in education is promising, but its realization will require a delicate balance between innovation and the essential human element of teaching and learning.
Inside DreamBox Learning How Educational Technology Specialists Shape Adaptive Learning in 2024 - DreamBox Teachers Build Custom Learning Paths Using AI Generated Student Performance Data
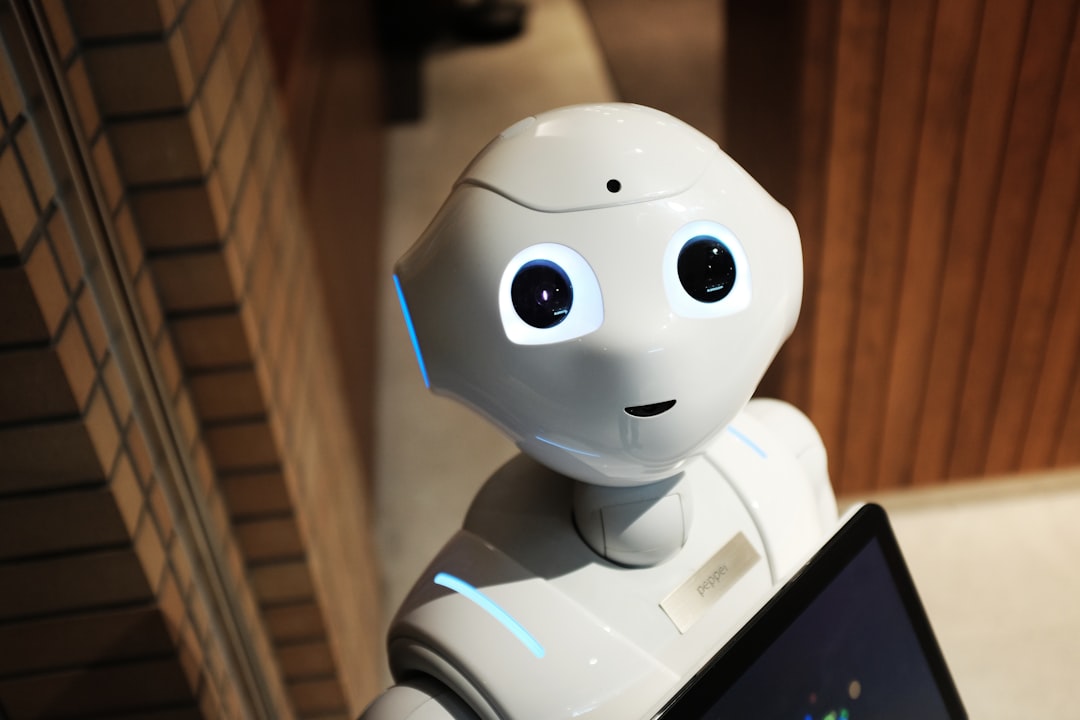
Within DreamBox Learning, teachers can design custom learning pathways using artificial intelligence insights gleaned from student performance. This system continuously analyzes each student's progress, allowing teachers to adjust math instruction in real-time to address individual needs. This approach seeks to personalize learning, aiming for improved student engagement and a deeper understanding of math concepts. While this method of adaptive learning holds promise, the dependence on AI for individualized instruction prompts questions about possible biases within the automated feedback and limitations of replacing the vital human element that teachers provide in a learning environment. As DreamBox evolves, the ideal balance between AI-powered tools and traditional educational methods remains a central topic in building successful learning experiences.
Within the DreamBox Learning environment, teachers are empowered to craft personalized learning pathways by leveraging AI-generated data about student performance. This capability allows for a more nuanced approach to instruction, tailoring the learning experience to each student's specific strengths and weaknesses. The system considers a wider range of factors beyond simply academic performance, potentially incorporating socio-emotional aspects into the learning path. While this holistic perspective promises to enhance learning effectiveness, the impact on a student's overall well-being needs more exploration and investigation.
Furthermore, DreamBox's use of machine learning allows for the identification of patterns across multiple student groups, enabling educators to pinpoint systemic learning gaps that might not be apparent within smaller datasets. This system-wide perspective can lead to valuable insights and potentially inform curriculum adjustments on a larger scale. However, there's a risk of losing sight of individual student needs when primarily focusing on aggregated data. If interventions are based solely on general trends, they might not effectively address the specific challenges that individual learners face.
The dynamic nature of these learning paths allows the system to adjust the difficulty of tasks in real-time based on each student's performance. This adaptive approach breaks away from traditional, linear curriculum models and potentially optimizes skill development. However, the flexibility of these pathways can lead to inconsistencies in pacing across a classroom. This can pose a challenge for collaborative learning opportunities, where students with differing paces might struggle to effectively work together on assignments.
Beyond individual students, the collected performance data can inform instructional decisions at the school level. This potential for large-scale adjustments can lead to modernizing educational approaches. But this centralized data analysis also raises questions about the level of autonomy teachers retain in decisions that directly affect their students' learning experiences. A careful balance is needed to ensure teachers can use their expertise alongside the insights from these data systems to create the best educational environments for their students.
The DreamBox algorithms extend beyond tracking academic success to include measures of time spent on tasks, providing insights into students' levels of engagement. While this information can be useful, solely relying on time spent to gauge engagement could overlook the quality of cognitive involvement and emotional connection students have with the material. A more comprehensive understanding of engagement is needed that goes beyond simply the duration of task completion.
The real-time feedback that these interactive dashboards provide has the potential to cultivate a sense of accountability among students, encouraging them to take responsibility for their learning. However, this can also lead to unintended pressure, where students might perceive their worth as linked to their performance metrics rather than their overall growth and learning journey. It's crucial to use data responsibly to encourage a healthy learning environment.
Teachers who utilize these dynamic assessment tools highlight the potential for greater differentiation in their instruction. The capacity for rapid adjustments based on ongoing data allows for tailoring lessons to individual needs. However, this data-driven approach should be used in conjunction with other forms of teaching, including interpersonal skills and teacher intuition, to avoid becoming overly reliant on automated insights.
These dashboards can facilitate immediate feedback loops that benefit both teachers and students. Students can access their performance data and monitor their progress. While this transparency can promote motivation, it's vital to ensure students interpret the data constructively and that it's not a source of undue stress.
The ability to dynamically adjust homework and practice activities can contribute to more effective individualized learning. Yet, the long-term sustainability of these personalized adjustments remains a valid question, especially given the diverse needs and complexities that often exist within a classroom.
DreamBox's interactive dashboards make it possible to visually represent complex student data analytics, simplifying the decision-making process for teachers. However, a key challenge is the potential for misinterpretation. Visual data representations can be misleading or overly simplified if not carefully examined within the broader context of the classroom and individual student needs. It's vital to avoid instructional strategies that are solely based on the quick visualization of data without deeper consideration of each student.
In essence, the AI-powered tools within DreamBox are providing exciting opportunities for educators to personalize learning. The impact of these tools on education will depend on careful implementation and continued research to fully understand the long-term effects on both student learning and the role of teachers in the classroom.
Inside DreamBox Learning How Educational Technology Specialists Shape Adaptive Learning in 2024 - Cross Platform Integration Links Classroom Instruction with Home Learning Activities
Connecting classroom learning with activities students do at home through different digital devices creates a more comprehensive learning environment, a kind of blended learning. Educational technology platforms, such as DreamBox Learning, allow for customized learning experiences that adjust to individual students' needs and learning paces, both in the classroom and at home. This approach shows promise in improving math skills. However, it's important to acknowledge that uneven access to technology could create unfair advantages for some students and impact how traditional teaching is done. Furthermore, as teachers integrate these technologies into their classrooms, they need to prioritize designing educational experiences that are meaningful and don't diminish the importance of the human interaction involved in teaching. To ensure the best educational outcomes for all students in this rapidly changing educational landscape, it's crucial to strike a balance between the benefits technology provides and thoughtfully designed instruction.
Cross-platform integration in educational settings strives to connect classroom learning with activities done at home, aiming to create a blended learning environment. This type of integration theoretically allows students to continue their learning journey outside of traditional classroom hours, which might enhance knowledge retention and learning effectiveness. However, practical applications frequently encounter major discrepancies in access to technology. These gaps can substantially limit the overall potential for such integration to benefit all learners. There are questions whether this approach really leads to better outcomes or just widens the gaps between student who can access technology and those who can not.
Research reveals a link between active parental engagement in a child's education, facilitated through these integrated platforms, and improvements in academic outcomes for the child. While positive, the amount of parental participation can fluctuate drastically and often ties to socioeconomic factors. This connection highlights important questions regarding equity within the education system, and suggests that not all students may experience these benefits in the same way.
These cross-platform tools often incorporate features for collaborative learning, allowing students to connect with their peers in real-time, thereby lessening the distinction between classroom and remote learning. This potential benefit is not without its drawbacks. While fostering teamwork, these collaborative tools can also lead to increased distractions and potentially reduce the amount of time students spend focusing on academic tasks. Educators will need to pay close attention to the implementation of these features and adapt them based on the context of the classroom and learners.
The ability to offer a variety of learning resources customized to unique needs is another goal of cross-platform integration. This creates the potential for truly differentiated instruction. However, the effectiveness of this personalization greatly depends on the teachers’ competence with these digital tools and their capability to find and utilize suitable educational materials. This can create challenges, especially for educators who may not be as comfortable or proficient with technology.
Data acquired from cross-platform learning environments provides insights into student behavior patterns and engagement. This data can be useful to develop early intervention strategies for at-risk students. However, it’s important to remember that the accuracy and trustworthiness of this data is dependent on students consistently participating in the platforms and the quality of engagement being measured.
These integrated platforms also introduce better communication channels between teachers and parents about a student’s academic progress. The idea is that this increased communication creates a more unified approach to teaching and learning. However, such a high level of communication can also result in increased anxieties and pressure on parents about the performance of their children, highlighting potential unintended consequences of improved communication.
Adaptive technology integrated across platforms seeks to create alignment between curriculum and the individual student’s learning styles. This customized learning approach can be beneficial, but can also risk producing somewhat isolated learning experiences. It's important to wonder whether students who primarily learn in this way are well-prepared to tackle collaborative learning challenges that will be presented throughout their lives.
Many learning platforms integrate elements of games into the learning environment to increase the appeal of home learning activities. While gamification can enhance student motivation, it's important to consider whether this type of engagement leads to a genuine understanding of complex concepts. The concern is that gamification may mask a superficial interest rather than fostering in-depth learning.
One interesting feature of cross-platform integration is its potential for personalized learning plans that adjust in real-time based on student participation. This capability raises important questions about how to ensure that these tailored experiences remain sufficiently rigorous and substantial. Maintaining depth of knowledge for every student, no matter how diverse the student population, is a very difficult challenge to tackle.
The aim of cross-platform integration in education is to create a more cohesive and unified experience across environments. However, questions linger regarding its potential to increase inequalities between different socioeconomic groups. The successful adoption of this technology will depend on ensuring equitable access to technology and supporting families who may not have access to the needed resources and tools. Further research is needed to determine the effects of cross-platform education on the various student populations across a diverse range of environments.
Inside DreamBox Learning How Educational Technology Specialists Shape Adaptive Learning in 2024 - Digital Learning Specialists Address Math Knowledge Gaps Through Skill Based Progression Maps
Within the evolving landscape of education in 2024, digital learning specialists are taking on a crucial role in bridging math knowledge gaps through the use of skill-based progression maps. These maps, utilized within adaptive learning platforms like DreamBox Learning, are designed to pinpoint specific mathematical skills where students may be struggling. By focusing on these individual skill gaps, the learning experience becomes more targeted and personalized. Educational professionals then leverage the data provided by these platforms to gain a deeper understanding of student performance, allowing for adjustments in their teaching strategies.
While this approach promises more effective instruction, there are concerns that relying solely on automated assessments and feedback might oversimplify the intricacies of learning. It's crucial to consider the potential for these technologies to overshadow the importance of a teacher's intuition and ability to adapt instruction based on their understanding of individual students. This dynamic approach underscores the considerable promise of technology in enhancing math education, while concurrently highlighting the necessity for a careful, balanced integration that ensures the pursuit of a more holistic educational experience for all students.
Digital learning specialists, particularly within platforms like DreamBox Learning, are increasingly employing skill-based progression maps to address the persistent issue of math knowledge gaps among students. These maps meticulously chart a path through a range of mathematical skills and concepts, tailoring the learning journey to individual student abilities and paces. The underlying idea is to provide a framework that allows students to navigate through math at their own speed, hopefully closing the gaps in foundational math skills that can hinder future progress. It's an approach that, in theory, allows for personalized learning, addressing the needs of students who may have fallen behind.
Research into the efficacy of this method has yielded some promising results, showing improvements in specific math areas for students utilizing skill-based progression maps. Some studies have even reported significant gains, with competency rates increasing by as much as 40% for struggling students. However, these results raise more questions than they answer: How can these positive results be scaled to benefit students in different educational settings and with varying backgrounds? Does the positive impact hold up over longer periods of time? It's still early to definitively say this approach is a universal solution, but it's intriguing enough to warrant continued exploration.
The construction of these progression maps hinges on sophisticated data analytics to pinpoint both student strengths and weaknesses. This data-driven approach is intended to provide a precise guide for instruction, but it raises concerns regarding an over-reliance on quantitative data. Might this approach oversimplify the intricate nature of student learning? It's important to recognize that student learning isn't solely a matter of numbers; qualitative aspects – the "why" and "how" of learning – may be sidelined when the focus is primarily on quantifiable achievements. Are we capturing the full picture of learning or simply a narrow band of measurable skills?
While the focus on skill progression is crucial, it's noteworthy that these maps also aim to incorporate socio-emotional factors. This broader perspective reflects a more holistic approach to education, acknowledging that student wellbeing influences learning outcomes. However, integrating this kind of social-emotional data presents a different set of challenges. Concerns around student privacy are central here. How do we use this kind of information responsibly and ethically in instructional decisions? What are the implications for how we understand students and design learning environments?
Another compelling aspect is the dynamic nature of these maps. The maps can be adjusted in real time based on a student's performance, guiding them towards areas where they need more support. This ability to adapt to student progress can be invaluable, but it also poses a challenge. What if the pace of adjustment is too quick for some students? Could they feel overwhelmed or discouraged if the pace of instruction doesn't match their comfort level? We need to find a balance between the personalized nature of learning and the need for learners to feel secure and supported.
Central to the implementation of skill-based progression maps is collaboration between digital learning specialists and educators. This collaboration is meant to align the maps with existing curricula and teaching practices. The success of this collaboration, though, is contingent upon the technological proficiency of educators. Many teachers are still building their comfort and confidence with educational technology. If this approach is going to be widely adopted, teachers will need access to robust training and professional development, ensuring they understand how the maps work and how to integrate them effectively within their classrooms.
These maps also have the potential to foster intrinsic motivation. As students see their progress represented visually on a map, they may become more engaged with their own learning, taking ownership of their journey. Yet, this emphasis on self-directed growth might inadvertently create pressures for some students, especially those who may not be accustomed to having this level of autonomy over their learning. It's vital that the maps empower students without unduly burdening them or creating anxieties about their abilities.
Furthermore, the maps function as a critical communication tool between educators and students, offering clear visual representations of learning pathways. However, a potential pitfall is that students may misinterpret the map's implications. It's possible for students to misjudge their performance and misinterpret expectations if the map isn't clearly explained and understood within the classroom context.
Educators have observed an increase in targeted interventions as a result of using these maps. The maps make it easier to identify specific areas where students struggle, allowing teachers to provide tailored support in those areas. It's likely that this targeted approach results in better outcomes for students. However, a potential challenge arises when there are numerous skills being tracked for a large number of students. It can quickly lead to data overload for educators if it's not managed carefully, potentially reducing time for instructional activities.
Finally, integrating these advanced progression maps with other educational technologies has the potential to create a seamless learning ecosystem. However, this interconnectedness highlights the necessity of responsible data management. Student data is incredibly sensitive, and with such intricate systems, the risk of privacy breaches becomes more pronounced. It's essential to ensure robust mechanisms are in place to protect student data while reaping the benefits of these sophisticated technologies. Striking this balance between technological advancements and ethical data management is crucial to building trust in these systems and guaranteeing a positive and productive learning environment.
In summary, while still in its early stages, the use of skill-based progression maps holds considerable promise in addressing longstanding math knowledge gaps. Yet, as with any emerging educational technology, careful implementation and sustained research are required to understand its long-term impact on student learning and teacher practice. By carefully considering the potential pitfalls and actively addressing them, educators can potentially leverage the benefits of skill-based progression maps to create more effective and equitable learning opportunities for all students.
More Posts from :