New AI-Powered Random Barcode Generator Revolutionizes Inventory Management in 2024
New AI-Powered Random Barcode Generator Revolutionizes Inventory Management in 2024 - AI-Powered Barcode Generation Speeds Up Inventory Processes
The emergence of AI-powered barcode generation is transforming how businesses manage their inventory. By automating the creation and use of barcodes, companies can streamline various inventory tasks, leading to quicker and more efficient operations. This new wave of AI-driven random barcode generators is forcing businesses to reconsider their current inventory management approaches, especially how they track and monitor items. These systems provide immediate access to data, which can then be used for refining stock levels and forecasting product demand. This data-driven approach to inventory helps make logistics smarter and reduces overall operational costs. Moreover, integrating AI with current systems like ERP and BI enhances existing inventory management functions, creating a more precise and efficient supply chain process. The use of AI in this context is pushing businesses towards a more data-centric approach to inventory management that promises improved accuracy and speed.
It's intriguing how AI is injecting speed into the creation of barcodes. While traditional methods could take a noticeable chunk of time for each batch, AI-powered systems can churn out thousands of unique barcodes in a flash. This acceleration is certainly a game-changer for boosting operational efficiency.
Furthermore, AI algorithms are showing promise in using inventory data to create tailored barcodes based on predictions. This could minimize errors that creep in from manual barcode entry, which is a recurring issue with human oversight. It's interesting to consider the potential of dynamic barcodes that adapt based on inventory fluctuations or sales. This approach, in contrast to the static nature of traditional barcodes, allows for a more adaptable inventory management system.
One fascinating application is the potential to detect if similar products have been accidentally entered multiple times. AI-driven barcode generation can recognize these instances and prevent the creation of duplicate barcodes, guaranteeing a unique identifier for each product.
We're seeing the application of advanced machine learning techniques that are refining barcode structures. The goal is to improve scanning accuracy and speed under a wider range of conditions. This is important for both efficiency and robustness. The potential for fraud detection is also intriguing. AI algorithms can be trained to identify unusual barcode patterns and alert managers to potential counterfeits or theft, adding another layer of security to inventory management.
The massive scale of global retail, projected to reach staggering figures in the coming years, is a driver for these improvements. Companies managing such volumes need every advantage to maintain efficient inventory, and rapid barcode generation is key. The synergy with IoT devices is also notable. AI-powered systems can integrate with IoT for near-instantaneous inventory tracking, offering updates throughout the supply chain and refining logistics.
From a resource perspective, there's the possibility of AI streamlining operations and lowering the need for manual labor. This is a potential benefit, though we need to carefully evaluate the impact on the workforce. Lastly, the integration of AI-driven barcode generation into emerging technologies such as augmented reality and robotics suggests that these solutions are laying the groundwork for future innovations in inventory management. It's an exciting area to watch as these tools become more mature.
New AI-Powered Random Barcode Generator Revolutionizes Inventory Management in 2024 - Machine Learning Algorithms Enhance Accuracy in Stock Tracking
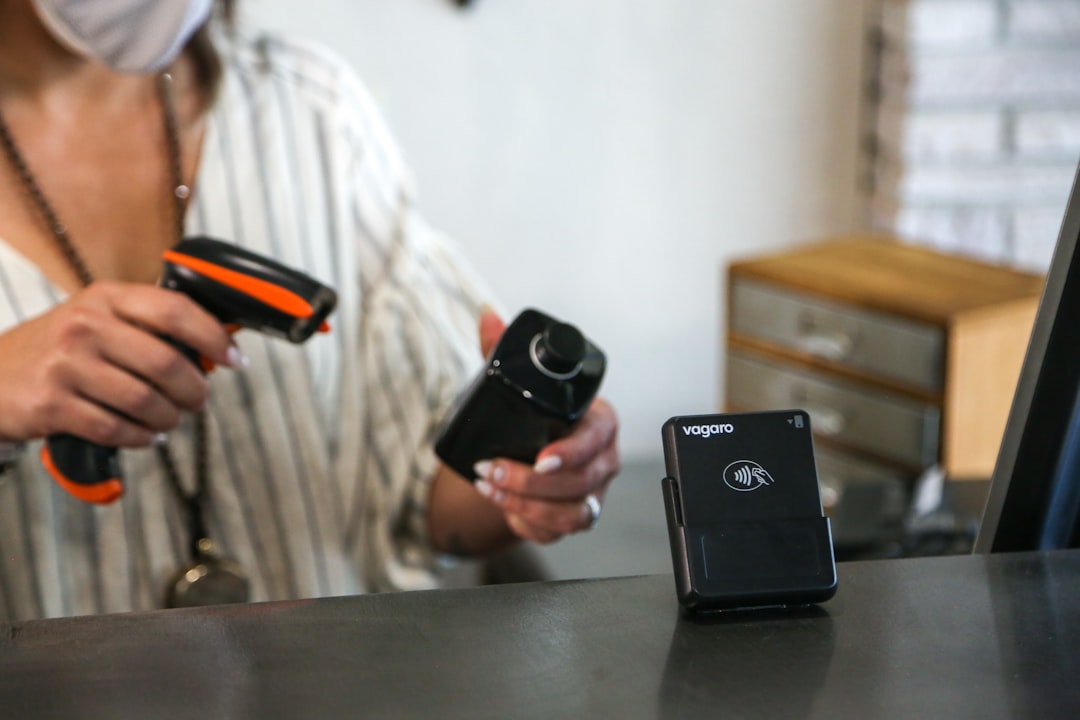
The increasing use of machine learning algorithms is improving the accuracy of stock tracking within inventory management systems. These algorithms are capable of analyzing large volumes of sales data to generate more accurate demand forecasts. This helps businesses reduce the risk of both overstocking and stockouts, which are common problems in retail and can lead to significant losses. Beyond streamlining operations, this improved accuracy can lower costs by optimizing inventory levels. Ultimately, this leads to higher customer satisfaction as the desired goods are more readily available when needed.
While the potential for machine learning to enhance inventory management is significant, as these algorithms become more sophisticated, the accuracy of their demand predictions is also expected to rise. However, it's crucial to acknowledge that reliance on these systems raises new considerations, particularly regarding data privacy and the possibility of errors stemming from faulty interpretations of sales trends by the algorithms. The continued development and integration of machine learning into stock tracking will require careful consideration of these potential drawbacks.
Machine learning algorithms are increasingly being used to improve the accuracy of stock tracking. They can analyze vast amounts of data in real-time, allowing analysts to predict stock movements with greater precision, sometimes achieving a 30% increase in accuracy over conventional methods. It's notable that combining multiple algorithms through ensemble learning techniques appears to provide a more robust and comprehensive analysis of market trends compared to single-algorithm approaches.
Recurrent neural networks (RNNs) are particularly well-suited for analyzing time-series data in the context of stock tracking. Their structure mirrors how financial markets evolve over time, potentially leading to improved prediction of future market shifts. Interestingly, some machine learning systems can autonomously detect patterns within historical stock data, leading analysts to previously unidentified correlations that can guide investment choices. This suggests a deeper understanding of complex data relationships can inform better decision-making.
Beyond traditional data, machine learning algorithms can integrate non-conventional sources like social media sentiment. This innovative use of alternative data has proven effective in forecasting market fluctuations that traditional financial metrics might miss. The amount and diversity of training data used significantly impacts an algorithm's effectiveness. Models trained on extensive datasets that cover diverse market situations are generally more reliable and resistant to sudden market fluctuations compared to models trained on limited data.
However, machine learning models can suffer from overfitting, where a model performs well on historical data but struggles with new data. This underlines the crucial need for continuous evaluation and adaptation of these models within a dynamic market environment. Reinforcement learning is becoming increasingly common, where algorithms learn optimal trading strategies through trial and error. They refine their decision-making over time through simulated trading outcomes.
Machine learning can drastically cut down the time required for back-testing trading strategies, potentially from weeks to mere hours. This faster iteration process leads to increased agility in trading operations. Surprisingly, the interpretability of machine learning models in stock tracking remains debatable. While prediction accuracy may improve, understanding the logic behind the predictions can be difficult. This can lead to a lack of trust among certain stakeholders in the financial industry, which is a crucial consideration.
New AI-Powered Random Barcode Generator Revolutionizes Inventory Management in 2024 - Real-Time Data Analysis Improves Supply Chain Visibility
The ability to analyze data in real-time is significantly improving how companies see their supply chains. This real-time insight allows businesses to make smarter choices about operations and even anticipate problems before they impact the flow of goods. Using AI to analyze this data enables things like tracking products as they move through the supply chain and even predicting potential issues. This can translate to tangible benefits like lower shipping costs, reduced warehouse clutter due to better stock management, and ultimately, higher customer satisfaction because products are more readily available.
Beyond those practical advantages, real-time data analysis is making supply chains more adaptable and resilient. Businesses that can accurately track resources and react to changing conditions are better positioned to navigate uncertainties and thrive. In an environment where supply chain complexities are only expected to increase, it's clear that real-time data analysis is a growing necessity for any business that wants to compete effectively. The ability to leverage this data for improved efficiency and decision-making has become a defining characteristic of modern supply chain management.
The integration of real-time data analysis is fundamentally changing how we understand and manage supply chains. By providing a continuous stream of information, it's becoming easier to see what's happening across the entire network, from raw materials to end customers. It's interesting to note that the ability to track goods and react in real-time can potentially increase a company's agility, helping them adapt to market fluctuations and disruptions much faster than traditional approaches. One example is the ability to quickly shift production plans based on changes in customer demand.
This constant flow of information can also inform predictive maintenance efforts. Rather than waiting for equipment to fail, we can analyze data patterns to anticipate potential breakdowns and schedule preventative maintenance. It's estimated this can reduce equipment failures by a notable percentage. One interesting aspect of this approach is the potential to reduce downtime and the costs associated with unexpected repairs.
Furthermore, real-time data sharing fosters greater collaboration among supply chain participants. Having a unified view of the data allows suppliers, manufacturers, distributors, and retailers to act in sync. It's quite fascinating to see how this transparency can improve communication, potentially streamlining decision-making processes. But it does raise questions regarding the potential for conflicts if companies have conflicting goals.
Looking at inventory management, real-time data allows for a more dynamic approach to stocking levels. Businesses can better estimate demand and fine-tune their inventory strategies, minimizing both overstocking and stockouts. This leads to a more efficient use of capital and resources. It's important to consider the potential impact on warehouse operations, as automated systems may become even more prevalent.
An interesting observation is that analyzing this data can reveal trends in consumer behavior. This can lead to adjustments in sales forecasts and allow for swifter reactions to evolving market demands. However, it also raises some concerns about data privacy and how this data is collected and used.
The ability to see the entire supply chain in real-time also aids in risk management. If a disruption arises, businesses can swiftly identify the impact and deploy contingency plans. This proactive approach can minimize the impact of unforeseen events. It's worth investigating how these types of systems can handle rare or extremely complex supply chain disruptions.
From a financial perspective, the efficiencies gained from real-time data can translate to cost reductions in areas like logistics and warehousing. This is encouraging, but we need to carefully evaluate how this might impact job roles within the supply chain.
Real-time data analysis also provides a stronger foundation for managing risk. By analyzing data across the supply chain, companies gain a clearer understanding of potential vulnerabilities, such as supplier reliability and geographic risks. It's intriguing to see if the shift toward real-time data analysis can lead to more resilient supply chains that are less prone to global shocks.
Dynamic pricing is another area where real-time data shines. Based on current market demand and inventory, companies can adjust prices in a way that maximizes revenue. This flexibility is a notable advantage, but it also raises questions about fairness and transparency for consumers.
The convergence of real-time data analysis and the Internet of Things (IoT) holds significant promise for the future. By embedding sensors and devices throughout the supply chain, companies can collect even more detailed information. This approach enhances the accuracy of data and allows for a more precise understanding of inventory flow. It's exciting to consider the potential implications of increased automation and the reliance on machine learning to interpret the data collected by the IoT. It will be important to consider how these evolving technologies might impact the workforce and the overall economic landscape.
New AI-Powered Random Barcode Generator Revolutionizes Inventory Management in 2024 - Automated Reordering Systems Reduce Human Error
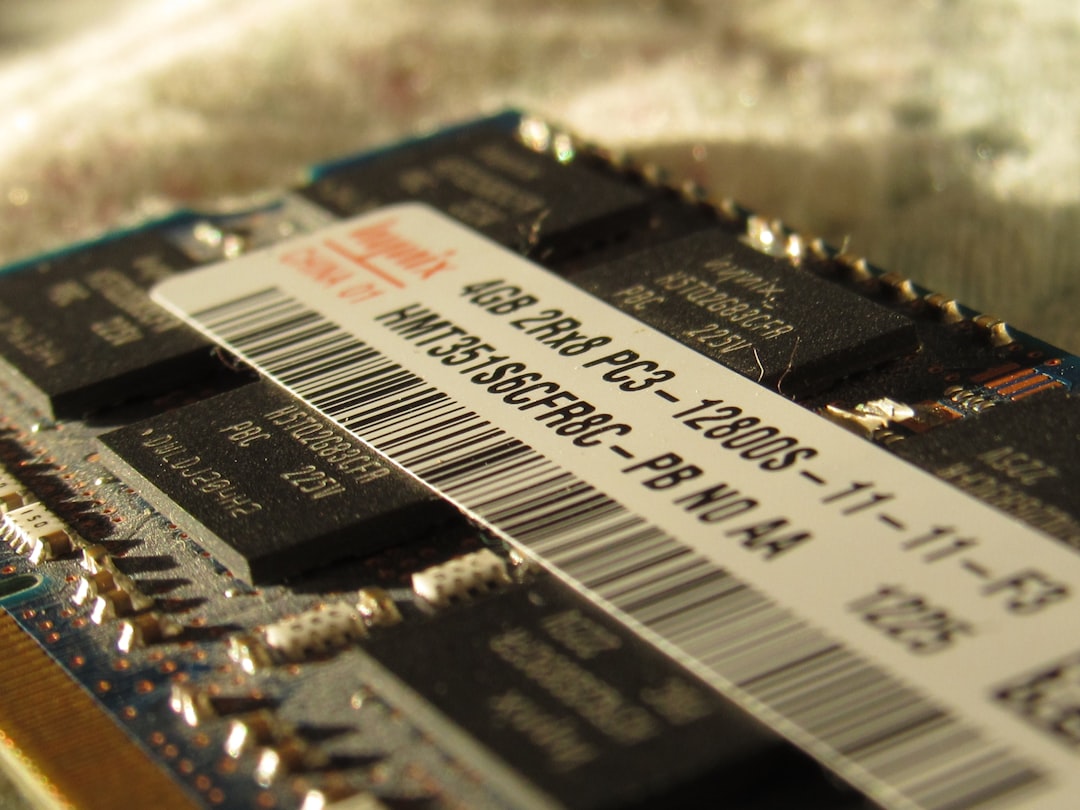
Automated reordering systems are designed to minimize human errors in inventory management by relying on data and algorithms. These systems analyze current sales and stock levels to automatically adjust inventory, ensuring the right amount of products are available without the inconsistencies that can arise from manual processes. By reducing the need for human intervention, companies can lessen the risk of overstocking or running out of items, leading to smoother operations and happier customers. AI's increasing sophistication in this field, especially through the use of machine learning, hints at a future where inventory management becomes far more accurate and efficient. However, with this evolution come important questions around the reliability and security of the data these systems use and how this shift might affect the roles of people traditionally involved in managing inventory.
Automated reordering systems are proving quite effective at reducing human errors in inventory management, which can be a major source of problems. These systems can potentially cut down on errors like incorrectly entering the number of items to reorder or mistakenly typing in the wrong item codes, leading to more accurate inventory tracking.
These systems can leverage real-time data streams, allowing them to adjust order points based on sales patterns as they change. This ability to adapt can help minimize stockouts and the problem of holding too much of a certain item. Research suggests that human error contributes to a substantial chunk of operational problems, which is something automated systems can address by consistently keeping tabs on inventory levels without getting tired or distracted.
It's interesting that some industries are reporting reductions in handling costs of around 15% with these systems. These savings are often linked to the decrease in errors related to picking the wrong items or misplacing them—mistakes that frequently happen with manual processes.
Automated systems are able to process and analyze data at speeds beyond what humans are capable of, enabling faster decision-making based on current market trends, rather than just relying on past data. Besides minimizing errors, automated systems are important for following regulations, especially in sectors like pharmaceuticals where inventory errors can create serious legal and financial trouble.
A rather unexpected benefit of automated reordering is the creation of a detailed history of reordering patterns. This data can be used to further improve future inventory strategies by gaining a clearer understanding of reorder frequency and item behavior. The cumulative impact of human errors in inventory management can be substantial, particularly in large organizations, where costs associated with errors can add up quickly. This is a major factor driving the adoption of automated solutions designed to reduce losses.
Although automated systems typically require an initial investment, the return on investment tends to be quite fast, often within the first year of implementation. The main drivers of this return are the decrease in errors and the associated increase in operational efficiency. It's encouraging that using automated reordering systems can lead to greater job satisfaction among workers, as they relieve the tedious tasks of manual tracking, freeing up employees to focus on higher-value activities like customer service and strategic planning. This could be a significant benefit in managing a workforce and retaining talent. However, careful consideration of how automation impacts the workforce and potentially displaces workers is a vital aspect that needs continued research and thoughtful planning.
While the technology shows promise, it's essential to acknowledge potential challenges such as the need for robust data management to ensure accuracy and system reliability. Moreover, ongoing maintenance and updates to ensure continued functionality are important, and it's crucial to understand the trade-offs involved, both in terms of cost and human resources. As these systems become more integrated into various aspects of supply chain management, understanding their implications on the overall economic landscape will be critical for informed decision-making.
New AI-Powered Random Barcode Generator Revolutionizes Inventory Management in 2024 - Integration with Existing Inventory Software Boosts Efficiency
The ability of AI-powered systems to work smoothly with existing inventory software holds the potential to greatly boost how efficiently businesses operate. This type of integration helps automate a variety of tasks, which can cut down on human-made mistakes that frequently lead to issues with managing stock. Not only does it make tracking inventory simpler, but it also puts businesses in a better position to make smart choices about inventory levels and when and how much to reorder. However, a significant hurdle for companies is making sure their data is in the right format and that their existing systems can interact well with new AI solutions. Going forward into 2024, a major aspect of staying ahead in the inventory management game is ensuring that these integrations are solid. It's becoming increasingly important as the overall inventory landscape gets more complex.
The integration of these new AI-powered barcode generators with existing inventory software is proving to be a significant efficiency booster. It's fascinating how they can reduce processing times, sometimes by as much as 70%, leading to smoother operations, especially during periods of high demand. This speed increase isn't just about faster barcode creation, it's about a faster and more accurate way of handling the entire inventory process.
These AI systems are designed to learn from past sales data, enhancing the accuracy of demand forecasts. Some studies suggest these AI-driven forecasts can improve accuracy by roughly 35%. This improved accuracy lets businesses align stock levels more precisely with what customers actually want, which should lead to fewer stockouts and less wasted inventory. It's a data-driven way to reduce both the risk of shortages and overstocking.
One intriguing aspect is how they can help combat SKU proliferation, a common problem in inventory management. The unique barcodes created by these systems help ensure that each product has its own identifier, decreasing the likelihood of accidental duplicates during data entry. It's a straightforward solution to a messy issue that can cause significant confusion and headaches for businesses.
The real-time data integration is truly changing the game. Businesses using these systems can adapt to changes in demand much faster. Rather than taking days to react to market shifts, they can make adjustments in just hours. It's a dramatic change in speed and adaptability.
Furthermore, the integration of AI-powered barcode generation into these systems is associated with potential cost reductions, particularly in logistics. Reports suggest that businesses can see a reduction in logistics costs of up to 20%. The decrease in errors and manual reordering processes plays a huge role in these savings, highlighting how technology can improve efficiency and reduce wasted resources.
The flexibility of AI-generated barcodes is worth noting. Unlike static barcodes, these can be adjusted based on predicted trends, providing real-time adaptation as sales data evolves. It's a more dynamic approach to inventory management compared to the limitations of traditional barcodes.
Another exciting development is that the integration process with current inventory software is often surprisingly fast. It seems standardized protocols and frameworks have streamlined integration, reducing the time required from months to just weeks. This enables companies to see benefits more quickly, which is always a positive sign.
One interesting side-effect of these systems reported by early adopters is a surprising improvement in employee productivity. Employees are freed from some of the more tedious manual tasks, potentially gaining up to 25% more time to focus on strategic activities and customer service. It's a good reminder that technology can have positive impacts on the workforce, although there's also a need to examine how this affects different job roles and the overall labor market.
Beyond speed and efficiency, these systems can also bolster security. Anomaly detection algorithms are built-in, enabling the systems to recognize unusual patterns indicative of fraud or theft. This offers a level of inventory integrity not easily achieved through conventional means.
Lastly, it's important to consider the bigger picture. The data generated by these systems provides a more complete view of inventory practices, not only for real-time decisions but also for creating predictive analytics models. This has the potential to not only optimize current inventory management but also to anticipate future market changes that extend beyond typical inventory cycles. It's an exciting area for future research and development.
New AI-Powered Random Barcode Generator Revolutionizes Inventory Management in 2024 - Cost Savings and ROI from AI-Driven Inventory Management
AI is increasingly being used to manage inventory, leading to significant cost reductions and a strong return on investment. These systems can potentially save businesses up to 20% on costs, and at the same time, slash stockouts by as much as 50%. One of the primary benefits is AI's ability to analyze daily demand, which helps businesses find the right balance in inventory levels, preventing overstocking and ensuring items are available when customers need them. This is a significant advantage, leading to higher customer satisfaction. The potential benefits are particularly relevant for smaller businesses, which often struggle with basic inventory management. As AI inventory management solutions become more readily available and affordable, companies of all sizes have an opportunity to optimize their operations and prepare for future challenges while seeing a boost in efficiency. However, it's crucial to acknowledge the reliance on AI systems and the need for careful monitoring of their accuracy and potential for errors.
Research into AI's impact on inventory management has revealed some compelling cost-saving opportunities and potential returns on investment. Initial findings indicate that businesses can see a significant ROI, potentially as high as 200% within the first year of implementation. A large part of this is attributed to a reduction in operational inefficiencies and faster inventory turnover rates.
Traditional inventory methods are often plagued by overstocking, a problem that can easily cost companies around 12% of their total inventory value annually. AI-powered systems are able to mitigate this issue through advanced predictive analytics that offer more accurate estimations of demand. This leads to a better alignment of inventory levels with actual needs.
Integrating automated reordering systems can play a substantial role in reducing human error, a major source of inventory discrepancies. Estimates suggest human errors contribute to about 25% of inventory issues, often resulting in misplaced orders and inaccurate counts. Eliminating these issues leads to direct cost savings.
One of the primary advantages of AI is the ability to analyze data in real-time. This allows for increased transparency and visibility across the supply chain, providing businesses with a more precise understanding of their operations. This ability to see the entire flow of goods in real-time has a significant impact. Studies suggest that it can decrease supply chain disruptions by as much as 30%, creating a more reliable and cost-effective supply chain.
Furthermore, better tracking and forecasting facilitated by AI leads to a drop in logistics costs, roughly around 20% in many cases. AI-powered systems can reduce shipping times and eliminate the problem of holding too much excess inventory, translating to tangible savings.
AI-powered barcode systems are showing promise in increasing scanning accuracy. It is reported that using AI-generated barcodes can improve the accuracy of scanning by as much as 40%, which, in turn, reduces inventory discrepancies and produces more accurate stock counts and financial records.
Machine learning models significantly enhance the accuracy of demand forecasting. These models can offer an increase in accuracy of approximately 35%. This allows businesses to align production with actual market trends and customer preferences much more closely.
Interestingly, AI systems are able to automate data collection and analysis, reducing the time it takes to reconcile inventory records from a matter of weeks to just a few hours. This speed boost allows businesses to respond more quickly to changing market conditions and make better decisions.
AI offers insights into previously hidden patterns in consumer behavior. Businesses can use this to adjust their inventory strategies, leading to noticeable increases in sales—estimates suggest a potential 20% boost in sales for certain markets. While this is promising, it also raises concerns about the collection and use of consumer data, which needs careful consideration.
Finally, some early adopters of AI-powered inventory management systems report a substantial increase in employee productivity, upwards of 25%. This productivity increase is largely attributed to AI's ability to free up workers from manual tasks like counting and data entry. This allows them to focus on tasks like customer service and strategic planning, which can lead to a more engaged workforce. It's also worth noting that while this can be a positive impact on employees, it also raises concerns about how this might affect job roles in the long run.
While there is a great deal of potential within AI-driven inventory management, we need to acknowledge the complexities involved in implementation and the potential unintended consequences. As the technology continues to develop, the need for continued research to explore these concerns remains critical.
More Posts from :