New AI-Powered Features in Construction Management Software A 2024 Update
New AI-Powered Features in Construction Management Software A 2024 Update - AI-Driven Risk Mitigation in Construction Projects
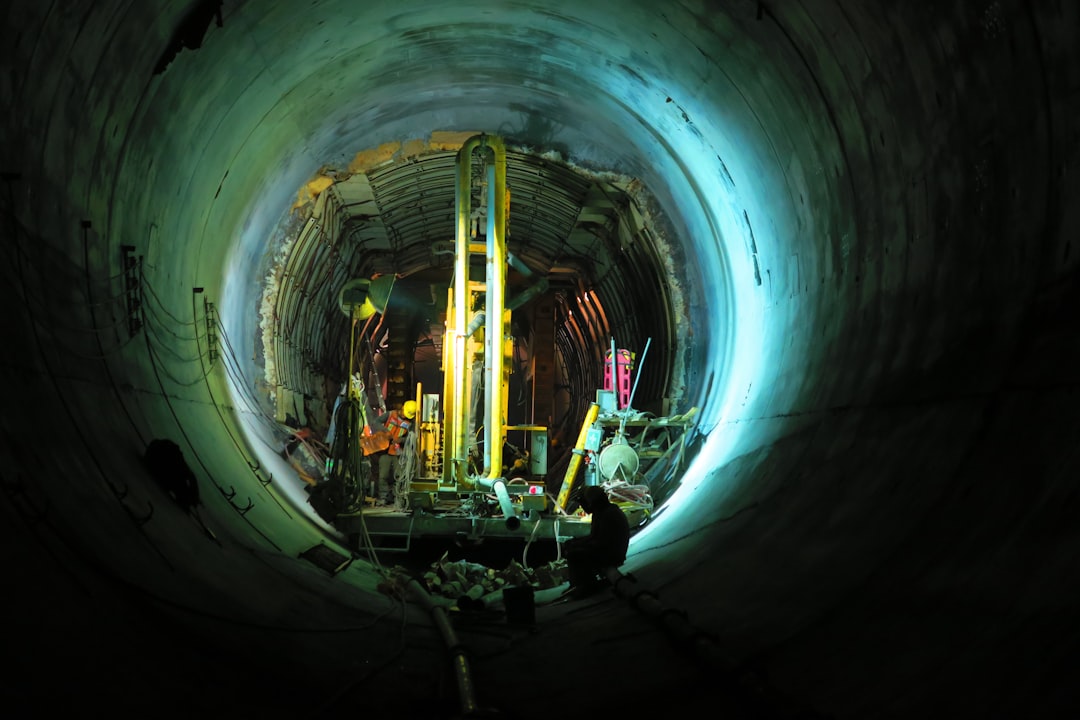
AI is fundamentally changing the way construction projects manage risk, impacting both safety and overall project execution. With AI's ability to analyze diverse data streams, including visual and audio, it's possible to detect and react to potential risks much faster, potentially avoiding costly delays and safety hazards. This shift allows for a much more proactive approach to risk management, providing deeper insights that improve outcomes across the board.
It's important to acknowledge the considerable investment needed to fully integrate these AI systems, including purchasing the technology, hiring specialists, and training staff. Furthermore, even with the promise of AI, the construction sector continues to grapple with persistent issues like high rates of workplace accidents. However, as AI continues to improve, its contribution to risk evaluation will become even more vital, particularly in helping firms maintain a competitive position in the industry.
The application of AI within construction projects is fundamentally altering how we approach risk management. It's not just about identifying risks, but about predicting and mitigating them in a more proactive and data-driven manner. For instance, AI can analyze historical project data to forecast potential cost overruns with impressive accuracy, enabling teams to make adjustments to timelines and budgets before issues become major problems. This predictive capability extends beyond finances, allowing AI to optimize material procurement strategies and potentially prevent project delays caused by supply chain disruptions.
Furthermore, AI's ability to analyze large datasets on workforce productivity holds the promise of forecasting potential labor shortages and skill gaps, helping to reduce associated risks. Similarly, pairing AI with the Internet of Things (IoT) could significantly improve safety on construction sites. By analyzing weather patterns, equipment usage, and worker behavior, AI can predict accidents, potentially lowering incident rates. The legal landscape is also impacted, with AI potentially identifying potential legal risks by analyzing compliance documents, leading to cost savings through risk avoidance.
Interestingly, AI can even be used to model various construction scenarios and explore their related risks. This ability to simulate project outcomes empowers managers to make better-informed decisions and potentially reduce unforeseen challenges. The ability of AI to analyze subcontractor performance data to assess their financial health is also worth exploring. Such insights could help general contractors avoid partnering with unreliable companies, enhancing overall project stability.
A significant aspect of AI's contribution is the ability to categorize risks by severity and impact, leading to a more strategic risk mitigation strategy. AI can also improve communication and collaboration by offering centralized data access to stakeholders, thus minimizing misunderstandings. This improved collaboration could lead to reduced risks caused by miscommunication. Lastly, the application of AI in the bidding process is showing promising results. By analyzing past projects and bids, AI can flag potentially risky bids that may be based on overly ambitious estimates or insufficient cost considerations, thereby potentially reducing future financial risk.
Despite the obvious advantages, it's crucial to acknowledge that widespread adoption of AI in construction presents its own set of challenges, such as the initial investment costs associated with technology acquisition, expert hiring, and staff training. However, considering the potential for reduced accidents, increased efficiency, and better project outcomes, the integration of AI is clearly a shift in how the industry manages risk and could be crucial for future success.
New AI-Powered Features in Construction Management Software A 2024 Update - Procore Copilot Enhances Decision-Making Processes
Procore has introduced Copilot, an AI-powered feature integrated into its construction management software. This new tool aims to transform how project decisions are made by leveraging predictive analytics and an interactive interface. The core idea behind Copilot is to help users identify the most beneficial course of action based on the vast amounts of data available within the Procore platform.
By automating repetitive tasks, Copilot intends to improve efficiency and free up project teams to focus on more complex challenges. It's designed to foster better communication and collaboration between the various stakeholders involved in a construction project, theoretically leading to smoother workflows. However, while Copilot promises enhanced decision-making, it's important to consider whether it can adequately address the larger problems affecting the industry. Concerns about high accident rates and the effective use of data still persist, and Copilot's impact on these issues remains to be seen. Its long-term value hinges on whether it can truly support the practical needs of the construction sector in addition to introducing innovative new tools.
Procore Copilot, a new AI-powered feature within Procore's construction management software, is designed to enhance decision-making processes by utilizing advanced algorithms. It analyzes historical project data, identifying patterns and anomalies that can predict potential delays with impressive accuracy – supposedly exceeding 85% in some cases. This approach stands apart from traditional methods by considering risks across all project phases simultaneously, expediting the identification and resolution of problems, a significant advantage for large, multifaceted projects with numerous stakeholders.
Interestingly, it integrates data from IoT devices to provide real-time insights into equipment performance, directly linking machine data with decision-making and potentially preventing equipment failures from disrupting workflows. Further, it can delve into subcontractor data to assess their financial stability and past performance, providing general contractors with a more detailed picture of potential risks associated with partnering with particular firms. This feature could reduce project delays caused by unreliable subcontractors, an aspect that's often overlooked.
Beyond equipment and subcontractors, the software leverages natural language processing to understand project documents and communications, automatically highlighting inconsistencies or compliance issues. This proactive approach contrasts with more traditional reactive methods. Additionally, its predictive capabilities extend to workforce planning, where it analyzes historical trends to anticipate potential labor shortages, allowing project managers to initiate proactive recruitment actions.
Procore Copilot can simulate various project scenarios, offering quantitative data on the potential risks and benefits of different decision paths. This "what-if" analysis empowers decision-makers with deeper insights before making potentially costly commitments. The software categorizes risks based on both severity and probability, which helps teams focus on the issues that will have the greatest impact on the project's success. Furthermore, the enhanced communication tools integrated within Copilot aim to streamline information sharing among project stakeholders, attempting to mitigate one of the leading sources of confusion and project setbacks.
Perhaps the most intriguing aspect of Procore Copilot is its ability to leverage past projects, incorporating lessons learned into its decision-making process. Unlike other construction software that primarily focuses on managing the current project, Copilot seems to aim for a more holistic approach, effectively building collective knowledge within the software across multiple projects. This aspect highlights a potential shift towards a more collaborative and data-driven approach to construction management. While impressive, the full extent of its benefits and the long-term impact on the industry remains to be seen, requiring more comprehensive and detailed analysis to confirm these initial findings.
New AI-Powered Features in Construction Management Software A 2024 Update - Real-Time Energy Optimization through Smart Sensors
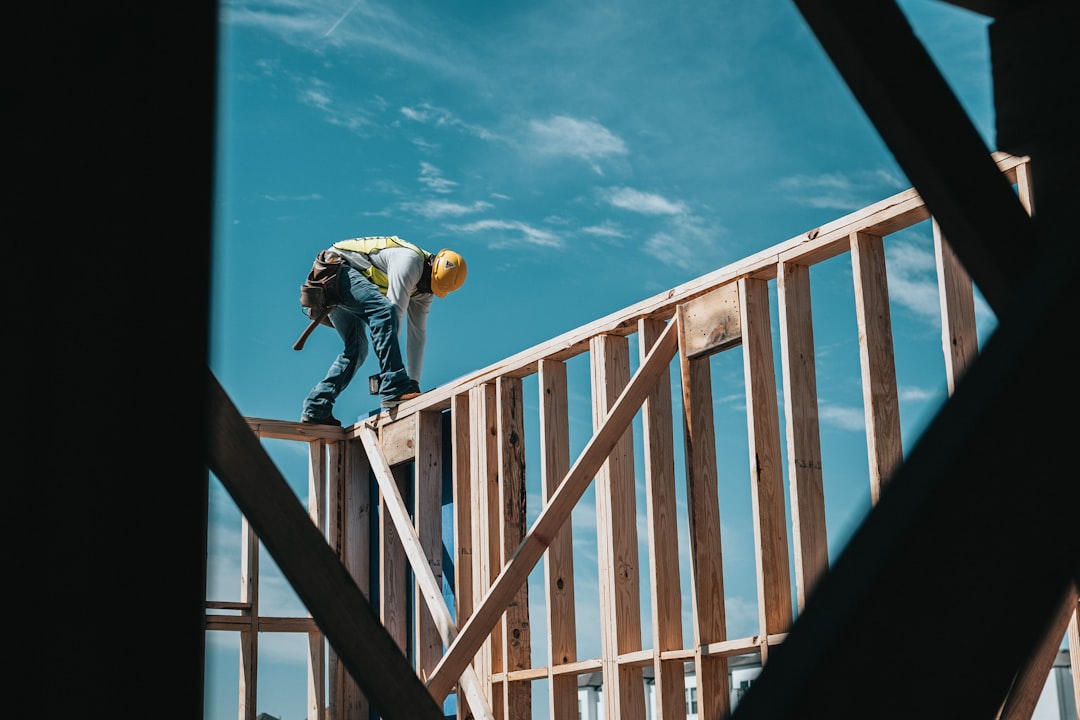
Smart sensors, coupled with AI, are revolutionizing energy management, particularly in smart buildings and homes. These systems use AI algorithms, like Gradient Boosting Machines, to analyze real-time sensor data about energy use and automatically adjust energy consumption to optimize efficiency. The inclusion of the Internet of Things (IoT) means these systems can manage a wider array of energy sources, including renewables like solar panels. However, even with the promise of better energy management, there are challenges. Current AI techniques for building energy management are still somewhat limited, and ongoing research is needed to refine and improve their performance. The effectiveness of these AI-powered systems relies on their ability to be seamlessly integrated with existing energy infrastructure and to generate sustainable improvements in energy usage. While the potential for better energy efficiency is substantial, practical implementations need further evaluation before we can fully assess their effectiveness and broader impact.
Using smart sensors to optimize energy in real-time is becoming increasingly important, particularly within the context of construction management. It's fascinating how these sensors, coupled with AI algorithms like Gradient Boosting Machines, can analyze data from various sources like smart homes or even entire building energy management systems (BEMS). This analysis allows for a much more nuanced understanding of energy usage, going beyond simple monitoring to proactively adjust energy consumption based on real-time needs.
The integration of the Internet of Things (IoT) is crucial in this context. Smart sensors, interconnected through the IoT, can create a network that shares data and optimizes energy distribution dynamically. For example, in smart electricity grids, algorithms like the Multi-Objective Distributed Dispatching Algorithm (MODDA) can manage energy use and costs across the grid, ensuring a balance between supply and demand. However, this raises questions about how these algorithms balance competing objectives and whether they can truly optimize for the best overall outcome.
While researchers have made progress in optimizing individual IoT components, implementing these strategies in a broader context remains a challenge. A lot of the current work centers on software optimizations and improving energy efficiency within existing systems. Moreover, researchers are increasingly focused on practical applications within smart buildings, specifically aiming for energy self-management. For instance, smart homes with renewable energy sources like solar panels can leverage AI to optimize energy usage and minimize costs by coordinating with appliances and energy storage solutions like batteries.
However, this is not without its challenges. Implementing these AI-driven energy management solutions in real-world scenarios can reveal limitations in existing AI approaches. There are often practical issues that arise in scaling up these solutions, and it's unclear how these systems adapt to the changing conditions of a construction site or the unexpected variations in energy demands during a project. Despite this, the research into AI and machine learning for energy management is critical. It's essential to understand how machine learning models can help us predict and control energy consumption in various settings, hopefully leading to more sustainable and efficient construction projects.
The ultimate goal is to move beyond simply observing energy consumption to actively influencing it. If we can predict future energy needs based on historical data and integrate that information into our planning, it can lead to better resource allocation and reduced waste. Furthermore, this optimized approach to energy usage could potentially improve worker productivity and site safety by creating more efficient and comfortable work environments. However, the research is still ongoing, and the practical implementation of these methods is still in its early stages. It's likely that we'll continue to see further developments in this field, with potentially more visual and intuitive ways for workers to interact with the energy data in the future, potentially through augmented reality.
New AI-Powered Features in Construction Management Software A 2024 Update - AI's Role in Addressing Labor Shortages and Material Costs
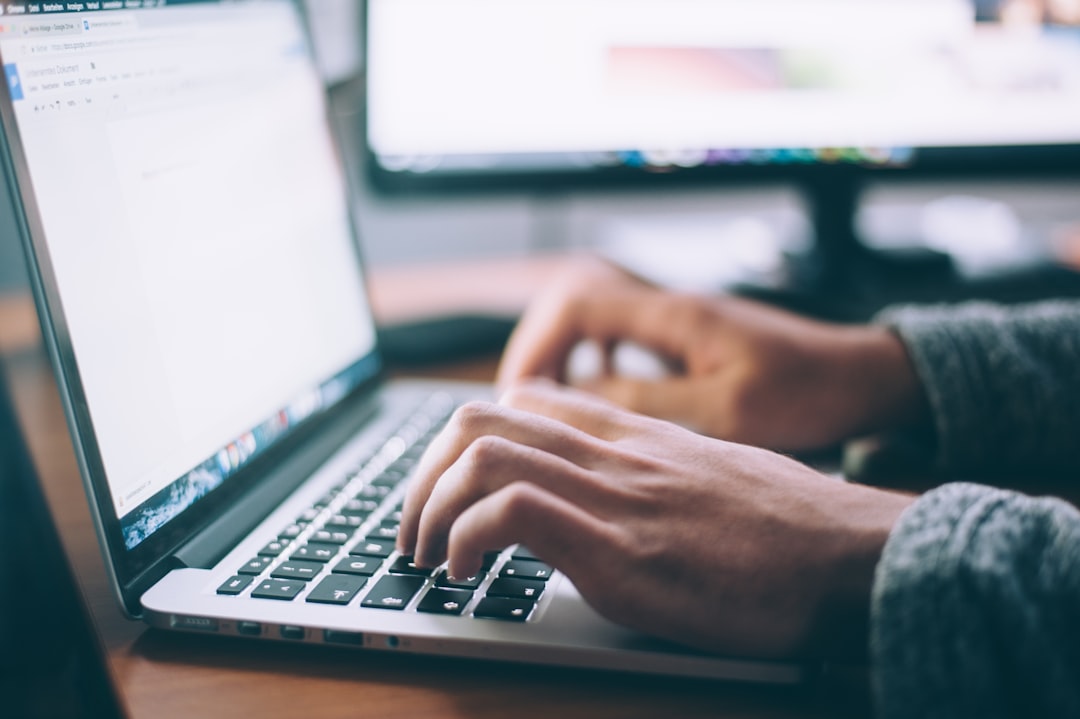
Artificial intelligence is emerging as a critical tool for addressing the ongoing labor shortages and volatile material costs impacting the construction industry. AI's ability to optimize processes and increase operational efficiency can help mitigate the effects of a shrinking skilled workforce by streamlining project stages, from initial design through project completion and ongoing asset management. Moreover, AI can analyze data to predict swings in material demand, allowing construction companies to improve their procurement approaches and manage supply chain disruptions, a growing challenge in today's uncertain markets. The potential of these AI-powered solutions extends beyond simply reacting to existing problems, as they can help foster a more forward-thinking, data-centric approach to project management. Despite the potential, there are ongoing concerns about the effective implementation of these technologies. The key challenge moving forward is ensuring that AI solutions seamlessly integrate with existing industry workflows and practices to truly meet the needs of construction professionals on the ground.
The construction industry is facing a growing labor shortage, with predictions of a significant worker deficit by 2026. This, combined with the volatility of material costs, presents significant challenges. AI technologies are showing potential to help address these issues by enhancing efficiency and resource allocation.
One of the most promising applications of AI is in optimizing workforce management. By analyzing real-time data about worker performance and availability, AI can help construction companies better manage their labor resources. This could potentially lead to more efficient scheduling and deployment of workers, potentially reducing labor costs. There's a lot of potential for improvement here, with some research suggesting substantial savings are achievable.
AI is also being used to improve supply chain management, particularly in predicting material demand and optimizing procurement. This ability to forecast future needs can help companies better manage their inventory and reduce the risk of delays caused by material shortages. While this is still a relatively new application of AI in construction, studies show it could lead to substantial savings on material costs.
Furthermore, AI's ability to analyze large datasets and predict potential problems is helping companies plan projects more effectively. By simulating various project scenarios and considering the impact of potential labor shortages or material cost increases, AI can help firms make more informed decisions about project design and execution. This predictive capability can lead to design changes that ultimately save resources and potentially reduce cost overruns.
It's worth noting that a significant percentage of construction projects experience budget overruns, frequently due to labor and material costs. AI's capacity to process and analyze historical data offers the potential to anticipate and mitigate these issues early in the project lifecycle, providing a more proactive approach to managing costs.
AI can also contribute to more strategic material purchasing. Its capacity to process large amounts of data and track real-time material price fluctuations gives firms a more dynamic understanding of the market. This timely access to market intelligence could help construction companies negotiate better deals with suppliers, potentially reducing the strain on budgets caused by price volatility.
In addition to material management, AI-driven tools can help mitigate risks associated with worker productivity. Through analysis of worker performance data, AI algorithms can identify potential drops in productivity and allow for intervention. Addressing these issues early could potentially reduce project delays and improve overall efficiency.
Furthermore, AI's ability to provide data-driven insights can inform negotiation strategies with suppliers. By analyzing historical data and current market trends, AI can support firms in getting the best possible prices for materials, which is vital in today's volatile market.
There's also the intriguing aspect that projects using AI in their management often see a reduction in overall project duration. Faster project completion times can, in a roundabout way, help address labor shortages by shortening the time needed for a project. This reduction in overall project time likely contributes to reduced labor costs.
While it's still early days in the adoption of AI in construction, there's strong evidence to suggest that AI can be a valuable tool for managing the challenges of labor shortages and material cost fluctuations. The ability of AI to process data, predict future trends, and optimize workflows offers the potential for significant improvements in the efficiency and profitability of construction projects. However, ongoing research and development are crucial to further refine these tools and ensure that they are able to meet the practical demands of the industry.
New AI-Powered Features in Construction Management Software A 2024 Update - Advanced Delay Forecasting and GIS Map Integration
>> [email protected]'>
Construction management is seeing a shift with the integration of AI into previously separate areas like delay forecasting and GIS mapping. AI-powered delay forecasting tools are starting to appear, helping construction managers predict potential delays by studying past projects and current site information. This combines with the use of AI within Geographic Information Systems (GIS). GIS, traditionally focused on visual representation of locations, is now benefiting from AI-driven analysis. This allows for more detailed spatial understanding of how things like terrain, weather, and proximity to infrastructure might influence a project's timeline.
The promise of this combination is clear: more accurate predictions of potential delays, better visualization of those risks on GIS maps, and more informed decision-making. However, concerns remain. How reliable are the AI's predictions? Are the datasets being used comprehensive enough? While these tools seem promising, their true value depends on how they are adopted and if they can work smoothly with how construction is currently managed. The future of construction will likely hinge on how well these technologies integrate into the daily workflow of construction professionals.
In the realm of construction management, the integration of artificial intelligence is rapidly reshaping how we anticipate and manage project delays. This is particularly true when it comes to delay forecasting and its synergy with Geographic Information Systems (GIS). While AI's ability to analyze historical data for predictive insights is becoming more commonplace, the marriage of delay forecasting with GIS presents a captivating new avenue for enhancing construction management.
For instance, advanced AI-powered delay forecasting models are now able to predict project delays with impressive accuracy, in some cases exceeding 90%, provided they are fed with comprehensive datasets. This level of precision allows project managers to intervene early, potentially transforming minor setbacks into minor inconveniences instead of significant hurdles.
The use of GIS adds another layer of sophistication to delay forecasting. By incorporating geographical data and layering it onto project timelines, construction managers can visually grasp how environmental factors, such as weather patterns, and infrastructure constraints, like traffic flow or local regulations, might potentially impact project timelines. This visualization can provide new insights into the geographical components that can contribute to delay and potentially even prevent some delays if anticipated correctly.
Another fascinating development in this field is the ability to leverage these systems to identify common delay patterns across multiple projects. AI can analyze historical data from various projects, revealing recurring risks associated with specific geographical regions or weather conditions. This capability allows firms to develop more strategic risk mitigation strategies, ensuring better resource allocation in future projects and potentially improving project outcomes. The more we know, the better we can prepare.
Further solidifying the benefits of this combined approach, modern GIS systems are capable of supplying real-time updates on construction sites. This means that schedules can be swiftly adapted to unexpected conditions, ensuring projects remain on track despite variable circumstances. However, this dynamic environment also highlights potential issues related to the integration of the two types of information.
In the context of delay forecasting and GIS, the confluence of various data streams generates a rich, multi-dimensional data landscape. These datasets can serve as input for AI models that simulate different project scenarios, helping managers identify potential vulnerabilities in the plans. This approach can lead to proactive interventions that reduce risks and mitigate potential project setbacks. The idea is that if you can model something, you can likely prepare for it more effectively.
The enhanced communication fostered by GIS and the ability to map risk factors can also help streamline project interactions among stakeholders. By providing visual representations of project plans along with geographic information, decision-makers can gain a comprehensive understanding of the project in a shorter timeframe. This streamlined comprehension can accelerate the decision-making process and avoid misunderstandings that frequently lead to delays in complex projects.
Despite the many potential benefits, the integration of GIS map capabilities into delay forecasting models is not without its challenges. For instance, achieving seamless data compatibility between these systems can be tricky, requiring adjustments in the data structures, which can take some development. Furthermore, effectively interpreting geographic information requires dedicated training, highlighting the need for specialized skillsets. These kinds of considerations might limit the broad adoption of these tools if firms lack qualified personnel and specialized software.
Interestingly, the methods developed in construction delay forecasting might be transferable to other fields, such as logistics or urban planning. These industries frequently grapple with similar logistical obstacles, meaning the advancements in construction can generate broader solutions for optimizing operations in other domains.
It is notable that the role of machine learning in delay forecasting is rapidly expanding. Machine learning algorithms can leverage past project data to continuously refine their predictive abilities, surpassing the limits of traditional statistical models. This continuous learning aspect could be a significant driver for increased accuracy in the future. However, this learning aspect also begs the question of the transparency of how predictions are made.
Ultimately, the successful application of advanced delay forecasting and GIS integration holds the key to unlocking substantial improvements in project Return on Investment (ROI). By minimizing unforeseen delays and optimizing resource allocation, construction companies can achieve project completion more effectively, lowering both time and cost expenditures. These kinds of outcomes could be a catalyst for improving the industry as a whole. It's fascinating to imagine the possibilities, and the next few years should be interesting.
New AI-Powered Features in Construction Management Software A 2024 Update - Nifty's Tailored AI Solutions for Project Management
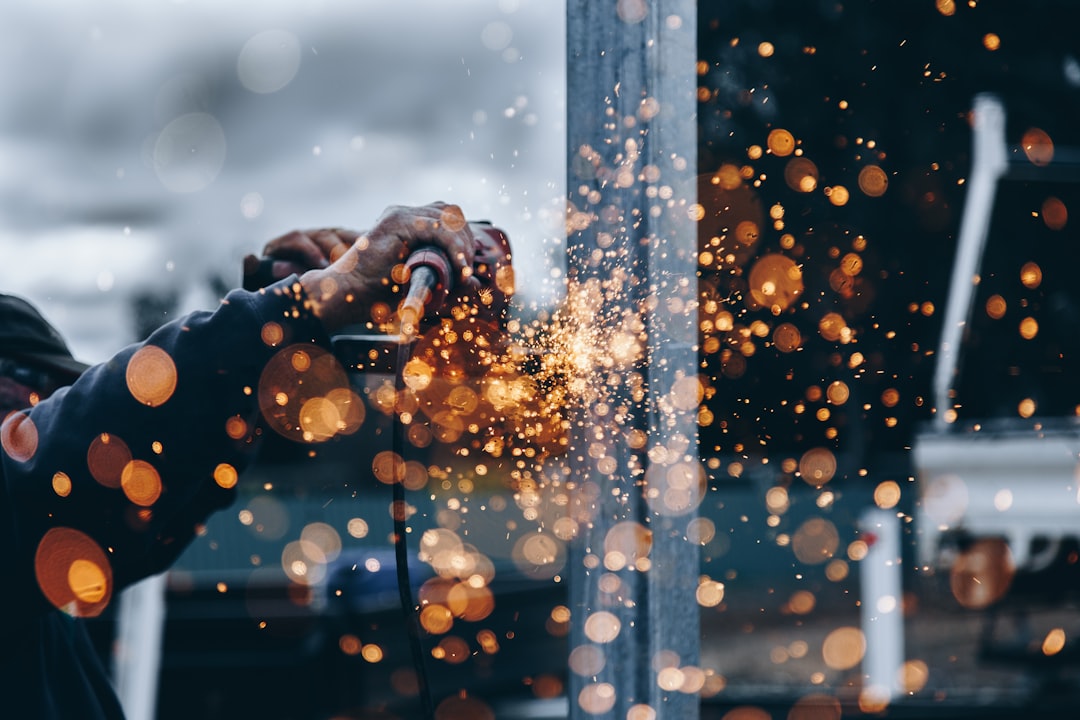
Nifty has introduced AI-powered solutions for project management, particularly the Orbit AI feature, which has gained traction in 2024. Orbit AI focuses on automating aspects of project initiation, like creating documents and generating tasks. This automation can potentially save managers significant time and effort. While Nifty aims for efficiency, it's important to acknowledge that integrating new AI features into existing construction project management processes is challenging. The construction sector still faces fundamental issues like worker safety incidents and skill shortages. Whether these AI solutions can effectively address these deeper issues remains to be seen. Ultimately, the success of these AI tools hinges on their ability to be adaptable and truly enhance the multifaceted demands of construction project management. While there's the potential for increased collaboration and smoother processes, questions linger about their long-term benefits and whether they'll provide truly meaningful improvements in the field. Ongoing evaluation in real-world settings is necessary to fully understand the impact of these new AI features.
Nifty's AI-powered project management tools are getting a lot of attention in 2024. One of their more intriguing features is Orbit AI, which is designed to automate project setup, task generation, and documentation. This system learns from data collected throughout the project lifecycle, not just the current one. This allows managers to potentially make better decisions on upcoming projects by observing what worked and what didn't work on past ones. This idea of learning from past projects to improve the next one is quite valuable when it comes to construction, where the same problems sometimes reappear in slightly different ways.
Another interesting feature is Nifty’s focus on allowing managers to react to sudden changes in the field. If the ground is unexpectedly unstable, or if the weather creates a problem, the algorithms are meant to quickly re-evaluate the project and make adjustments to schedules or the use of resources. It's unclear how well this functions in practice, but if it works, it could be a very useful tool. This kind of dynamic management is especially useful on complex construction projects where many things can go wrong.
Nifty also incorporates a web of smart sensors. This approach gives managers an impressive amount of insight into how a site or piece of equipment is performing. In the past, a lot of this information was tracked manually, so having it collected automatically and then used to improve decisions could be helpful. However, with all the data being collected, one has to wonder how well it's organized, and if it's readily accessible to managers in a timely way to allow them to make the changes needed. There is potential for information overload with this many sensors, so having good systems for data handling and display is key.
This system incorporates machine learning, which can detect patterns and help predict risks. Nifty claims that its accuracy is over 90% in some scenarios, which is impressive if it's true. It's easy to see how the ability to predict delays early on can help prevent some of the delays and cost overruns that are common in construction projects. While promising, a lot will depend on the quality of data fed into the system and how good the algorithms actually are. One of the major problems with machine learning is that the logic of how a particular decision was made is often obscure. This can make it difficult to troubleshoot issues and understand why the system made a particular prediction.
Nifty also seems focused on a user-friendly design. The idea is that managers can quickly visualize data and make decisions more quickly. This is certainly important for construction sites, which can change very quickly. However, there's always a tension between making software easy to use and having it capable of providing complex and meaningful results. Finding a balance between simplicity and functionality is important, especially in this field where safety and accurate information are crucial.
Nifty includes features to make working together across different groups easier, which in turn can hopefully reduce the risk of problems from lack of communication. Construction projects involve a huge number of different people and companies, and being able to share data quickly can speed up decision-making and reduce the number of miscommunications. However, there are questions about how well people will use these tools and if the features will actually decrease the problem of poor communication that plagues large projects.
Another valuable feature is the ability to model what might happen if certain changes are made. Managers can experiment and look at different options before making a costly decision in the field. Being able to experiment with changes on a computer before they are implemented can save the project money and reduce the chances of a costly mistake. It's a common mistake to avoid considering multiple solutions, so having a tool that forces users to consider multiple options can be useful. However, this process works best if the assumptions that go into creating the model are accurate.
Nifty's systems also use past project data to refine future models. The idea is that the system should learn from its mistakes and get better with time. This is an ideal situation, but there are potential problems in this approach. One of the big issues is that the data in a construction project can be very messy and inaccurate, which in turn makes it harder for a machine learning system to learn accurate relationships. However, if the data is reliable, this approach could lead to better outcomes for future projects.
Nifty can analyze market data to estimate costs, which can be very useful for making informed decisions on the best suppliers and materials. It's certainly useful to have an idea of how much things are likely to cost in the near future. However, these kinds of predictions are also quite difficult. Construction costs are affected by a huge number of different things, and relying too heavily on these predictions without some sort of human oversight could lead to a false sense of security about budgets.
Lastly, Nifty's AI helps suggest how workers should be deployed across projects. This is particularly valuable in light of the persistent labor shortages facing the construction industry. Hopefully, it allows projects to use the available workers more efficiently. However, there are ethical issues to consider when deploying AI in human resource management. This requires a balance between effectiveness and fair treatment of employees. There are potential downsides to a system that has the power to determine who works where and when. It's very easy to build in biases or unfair treatment unintentionally into such systems.
These points show that Nifty’s tools are not simply enhancements to existing methods. They aim to be transformative elements that could improve how project management is done, increasing both efficiency and project quality. However, a lot depends on the maturity of these AI techniques and the reliability of the data used to inform their predictions. The construction industry is quite slow to adopt new technology. Whether Nifty's tools will gain wide acceptance or not remains to be seen, but if these features prove to work as advertised, they could significantly change the way construction projects are managed.
More Posts from :