CRM Software in 2024 AI Integration Reshapes Customer Management Landscape
CRM Software in 2024 AI Integration Reshapes Customer Management Landscape - AI-powered Predictive Analytics Reshape Customer Segmentation
In 2024, AI is fundamentally altering how we define and interact with customer segments. AI-driven predictive analytics delve deeper into customer behavior than ever before, enabling a level of personalization previously unimaginable. Marketers are now able to craft campaigns that speak directly to the nuances of individual customer preferences and needs. This granular approach to segmentation not only fosters stronger engagement but can also contribute to increased customer loyalty—people tend to respond more favorably to interactions that recognize and cater to their unique characteristics.
Furthermore, the integration of AI is automating numerous customer management processes. This automation not only reduces manual effort but also helps maintain clean and accurate customer data. This is vital for optimizing the customer journey, ensuring relevant and consistent interactions. In this new environment, companies that embrace AI are gaining a distinct edge. They are better equipped to understand the intricacies of their customer base and respond accordingly, ultimately cultivating richer, more meaningful relationships. While this shift promises substantial benefits, the reliance on increasingly complex AI systems also raises concerns about data privacy and control that businesses must navigate carefully.
It's fascinating how AI-powered predictive analytics are transforming how we understand and categorize customers. By meticulously examining past behaviors, demographic data, and customer interactions, these systems can segment customer bases with remarkable precision, potentially reaching a 95% accuracy rate. This level of granularity is a game-changer, significantly minimizing the risk of misdirected marketing efforts.
These algorithms are uncovering subtle patterns in customer actions that previously went unnoticed. This allows companies to adapt their marketing tactics in real-time, making interactions more relevant and engaging. Research suggests that implementing AI-driven segmentation can lead to a substantial increase in sales conversions, possibly exceeding 20%, which hints at a significant return on investment for companies embracing this technology.
What's particularly impressive is the ability of AI to anticipate customer needs and preferences based on information from various interactions. Businesses can now proactively tailor offers to customers, potentially meeting their needs before they even articulate them. The continuous learning nature of machine learning further enhances predictive analytics. These systems learn and adapt to ever-changing customer behaviors, making them more effective over time.
Furthermore, predictive models can help anticipate customer attrition – predicting which customers are at risk of churning. This early warning system gives companies the opportunity to implement targeted retention strategies to keep these customers engaged. It's not just about segmentation, though. These models are also capable of identifying emerging market trends, enabling businesses to strategically adapt their offerings and stay ahead of evolving customer preferences.
The real-time capabilities of AI are truly impressive. While conventional methods often rely on batch processing, AI-powered systems can analyze data in real-time, enabling immediate adjustments to marketing based on live data streams. Moreover, predictive models can handle massive volumes of diverse data from numerous sources, streamlining the segmentation process and eliminating the bottlenecks and errors often associated with manual data analysis. This automation translates into significant time and resource savings.
Many companies are witnessing substantial reductions in marketing expenses, often exceeding 30%, after integrating predictive analytics into their CRM systems. This efficiency gain stems from highly targeted campaigns that produce better results while minimizing the waste associated with generic, mass-market approaches. It's clear that in the ever-evolving customer management landscape, AI-powered predictive analytics are playing a pivotal role in enabling businesses to interact with customers more effectively.
CRM Software in 2024 AI Integration Reshapes Customer Management Landscape - Natural Language Processing Enhances Chatbot Capabilities
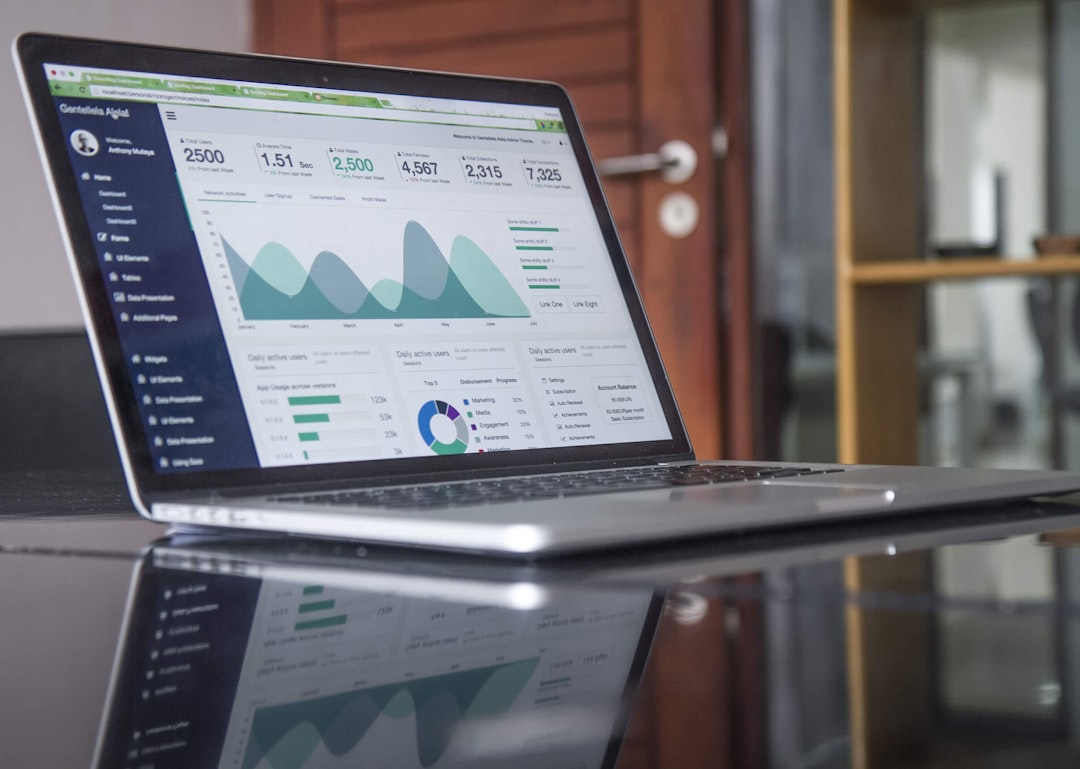
Within the evolving landscape of CRM software, Natural Language Processing (NLP) is revolutionizing how chatbots interact with customers. These chatbots are moving beyond simple, pre-programmed responses, now able to understand and respond to more complex and nuanced language. This means more natural conversations and a more personalized experience for the customer.
The addition of large language models (LLMs) further boosts these capabilities, allowing chatbots to generate more sophisticated and contextually appropriate responses. However, the gap between how companies believe they're personalizing customer experiences and what customers actually perceive remains significant. This highlights the importance of utilizing data intelligently and thoughtfully within AI-powered CRM systems.
By taking over routine, repetitive tasks, NLP-powered chatbots free up human customer service agents to handle more complex issues. Simultaneously, the data gathered by these interactions can provide valuable insights into customer behaviors and preferences, helping companies fine-tune their overall strategies to enhance customer relationships in an increasingly competitive marketplace. While there's clearly progress, effectively utilizing NLP within CRM is a dynamic challenge, requiring constant evaluation and adjustment.
Natural Language Processing (NLP) is significantly boosting the capabilities of chatbots, allowing them to understand and respond to customer interactions in more nuanced and sophisticated ways. They can now assess the sentiment behind customer messages, differentiating between positive, negative, and neutral interactions with impressive accuracy—researchers report accuracy rates as high as 85%. This ability to understand the emotional tone of interactions allows companies to quickly spot and address customer dissatisfaction, potentially improving service responsiveness.
Interestingly, these newer NLP models are bridging the gap between formal language and everyday conversation by recognizing slang and colloquialisms. This helps chatbots engage with users in a more natural and relatable manner, fostering better interactions. Moreover, they're no longer flustered by multi-turn conversations. Through improved algorithms, chatbots can maintain context across multiple exchanges, allowing for more meaningful dialogues and reducing the common frustration of lost conversational threads.
NLP algorithms are also becoming increasingly adept at distinguishing between different ways of phrasing the same request, meaning chatbots can handle a wider range of questions and expressions more efficiently. This ability to handle variations in language reduces the likelihood of miscommunication and contributes to a more positive customer experience. They can even extract key information from longer communications—like customer histories or documents—and summarize it for faster, more targeted responses.
It's quite remarkable how NLP can dynamically identify entities and categorize information within conversations. For instance, if a customer mentions a specific product, the chatbot can recognize it and incorporate that information into subsequent responses, leading to a more personalized experience. The expanding use of voice-activated chatbots further highlights the power of NLP—converting speech to text and understanding intent in hands-free settings. This is expected to become a significant method of customer interaction in the near future.
Furthermore, NLP-powered chatbots are getting better at detecting emotional cues and subtleties in language. This means they have the potential to generate responses that are more empathetic and resonate more deeply with the user, fostering stronger connections. NLP's capability to handle multiple languages is also crucial in a globalized market. It allows companies to provide consistent and high-quality support to customers across various language barriers without needing extensive translation or support teams for each language.
Finally, NLP plays a critical role in allowing chatbots to scale and handle a large number of concurrent interactions. This capability significantly reduces reliance on human agents during peak demand periods, leading to potential cost savings and improved response times—both essential factors in maintaining a competitive edge in customer service. While NLP has made remarkable advancements, the complexities of human language continue to present challenges for researchers. It will be interesting to see how these capabilities evolve and how NLP continues to reshape customer interactions moving forward.
CRM Software in 2024 AI Integration Reshapes Customer Management Landscape - Machine Learning Algorithms Optimize Sales Forecasting
In 2024, machine learning algorithms are fundamentally changing how sales forecasting is done. Businesses are seeing improved accuracy in sales predictions, with some reaching over 75% accuracy using AI-powered tools. This is a big deal, since many companies (about 67%) are still unsure about the reliability of their traditional forecasting methods. CRM systems are now being enhanced by AI, giving businesses more powerful analytical tools and a deeper understanding of customer behavior, which leads to better engagement. While these algorithms can be very effective, their complex nature means they aren't necessarily easy to use, and companies need to consider how to best integrate them into their existing systems. The impact of these algorithms is that they're essentially changing the very core of customer relationship management, demanding a new level of data-driven decision-making from businesses moving forward.
In 2024, machine learning algorithms are proving quite adept at analyzing historical sales data, picking up on trends and patterns that often escape human analysts. Some of these models are reaching accuracy rates above 90%, which is pretty impressive for helping guide better decisions and manage inventory more effectively.
Unlike traditional forecasting which often relies on simpler methods like linear regression, machine learning models can juggle multiple variables at once and understand non-linear relationships. This makes for a more holistic view of all the factors impacting sales.
For example, understanding how seasonality influences sales is much more refined with these algorithms. They can learn and study different cyclical patterns over time, which is quite helpful for getting ready for periods of peak sales and optimizing supply chains accordingly.
Some of the more advanced machine learning techniques use what are called ensemble methods, where they combine predictions from several different models. This helps to lessen the impact of outliers on the forecast, resulting in more reliable data-driven insights.
Not only is the general accuracy of forecasting improved, but in some industries, these models have cut forecast errors by over 50%. This means less wasted money and greater profitability since businesses can align product availability with anticipated demand much more precisely.
One cool thing about machine learning sales forecasting is that it's not static. These models can constantly adapt as new data comes in, allowing businesses to update predictions in real-time. This dynamic nature gives a huge edge over the older methods that require tedious recalibrations.
Even more intriguing is how machine learning is being used to analyze customer sentiment from places like social media and online reviews. By linking this sentiment with sales patterns, businesses get insights into potential shifts in the market before they even show up in standard sales cycles.
Machine learning models that integrate macroeconomic indicators, like unemployment rates or consumer confidence, are also cropping up. These models can help businesses anticipate shifts in market conditions and adjust their strategies beforehand.
It's interesting that the use of Internet of Things (IoT) data within machine learning sales forecasting is growing. Data from smart devices can offer real-time insights into customer behavior and how products are being used, making forecasts even more reliable.
While these methods have clear benefits, one big challenge is the quality of data used. If the data is inaccurate or incomplete, the forecasts can be misleading. This highlights just how important data governance is when implementing these complex algorithms.
CRM Software in 2024 AI Integration Reshapes Customer Management Landscape - Automated Sentiment Analysis Improves Customer Feedback Processing
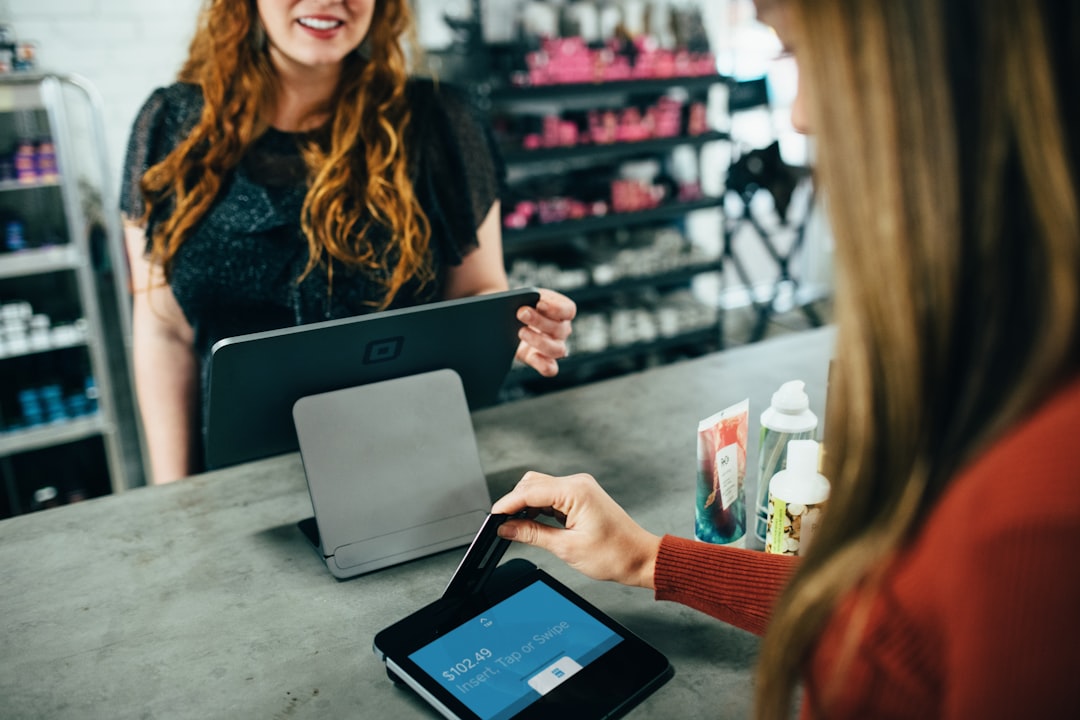
Automated sentiment analysis is revolutionizing how businesses handle customer feedback, providing a deeper understanding of customer emotions, preferences, and concerns. Using AI techniques like natural language processing and machine learning, companies can categorize customer sentiment into positive, negative, and neutral categories, offering near-instant insights that directly improve customer experience. These AI tools can handle massive amounts of data with greater efficiency and accuracy compared to manual review, making them highly scalable. Further, automated sentiment analysis allows companies to proactively identify and resolve customer issues before they become larger problems, thereby minimizing potential damage to their reputation and nurturing stronger customer connections. As AI continues its integration into customer relationship management (CRM) systems, this technology empowers organizations to make better data-driven decisions that are more attuned to customer wants and needs.
AI-powered sentiment analysis is transforming how businesses process customer feedback in 2024. It can handle massive volumes of qualitative data—like social media posts or reviews—at a speed far exceeding human capabilities. This is allowing companies to gain valuable insights from a much broader range of customer interactions in real-time.
Current AI models demonstrate impressive accuracy, exceeding 90% in some cases, in pinpointing the subtle emotional nuances within customer feedback. This granularity is enabling companies to finely tune their product offerings and services, making adjustments based on specific customer sentiments, like degrees of satisfaction or frustration. This level of precision has proven to be particularly helpful in streamlining customer service. When integrated into CRM platforms, sentiment analysis allows for nearly instantaneous responses to negative feedback, significantly improving response times and customer retention.
Interestingly, the implications of sentiment analysis extend beyond simply enhancing customer service. The insights gleaned from automated analysis are feeding directly into strategic marketing efforts. By identifying which product aspects resonate most strongly on an emotional level with customers, marketers can design highly targeted campaigns, boosting the effectiveness of their efforts.
It seems that AI-powered sentiment analysis is also a valuable tool for understanding and potentially even predicting broader market trends. Businesses that implement this technology are not simply reacting to feedback; they're gathering insights that allow them to anticipate shifts in consumer preferences before they become widespread. This is leading to more proactive and effective product development and marketing strategies.
Furthermore, sentiment analysis is revealing inconsistencies between how customers perceive a product and how it actually performs. This can uncover misalignments in marketing messages or product delivery that might otherwise go unnoticed.
The algorithms powering these sentiment analysis tools are continually learning and evolving. They are adapting to changes in language, slang, and colloquialisms, ensuring they remain relevant as customer communication styles change over time. This adaptive nature is crucial for maintaining the effectiveness of the analysis.
This ability to quickly process and understand customer sentiment is especially valuable during product launches. Real-time analysis allows companies to gauge the initial response and, if necessary, quickly adjust marketing strategies or product features before negative sentiment builds momentum.
Beyond identifying unhappy customers, AI-powered sentiment analysis is revealing brand advocates. Companies can now pinpoint customers who are enthusiastic and positive about their products, allowing them to design strategies for upselling or tailoring loyalty programs to their needs. This is shifting how we think about building stronger, more meaningful customer relationships.
Finally, and importantly, as data privacy regulations are becoming increasingly stringent, AI sentiment analysis tools are being developed with compliance as a core design feature. Businesses can now utilize these technologies to glean actionable insights without putting sensitive customer data at risk, demonstrating a shift towards more ethical and responsible data handling.
CRM Software in 2024 AI Integration Reshapes Customer Management Landscape - AI-driven Personalization Engines Tailor Marketing Campaigns
In 2024, AI is fundamentally altering how marketing campaigns are designed and delivered. AI-powered personalization engines are now capable of creating highly targeted marketing messages and content based on detailed insights gleaned from customer data. These engines analyze a wide range of information, including purchase history, browsing behavior, and even social media interactions, to generate customized experiences. The goal is to create what's called "hyperpersonalization"—marketing that speaks directly to the individual customer. Many companies report improved results with AI-generated content compared to traditional approaches, demonstrating the power of this technology to enhance customer engagement.
However, the rise of these sophisticated AI tools also brings a new set of complexities. Building and managing AI-driven personalization systems requires a level of expertise that many businesses may not have in-house. This is leading to an increased reliance on outside specialists and partnerships, as organizations seek to leverage the benefits of AI without the burden of developing those complex capabilities internally. As we move forward, navigating the ethical and practical challenges of data privacy and management will become even more important for organizations embracing this type of personalized marketing. While it presents exciting opportunities for businesses to connect with customers on a deeper level, it's clear that AI-powered personalization is also a technology that needs to be carefully managed.
AI-powered personalization engines have gone beyond basic customer groupings, achieving impressive accuracy—up to 95%—in predicting individual customer preferences based on behavior and past interactions. This fine-grained precision not only makes targeting more effective but also helps significantly reduce errors in personalized marketing efforts. It's like having a highly refined microscope for understanding individual customers.
Interestingly, companies utilizing these engines are reporting a reduction of up to 30% in overall marketing expenses. This financial benefit comes from the ability to create focused campaigns that resonate with specific customer segments, instead of using the old method of casting a wide net with generic messages. It's a shift from shouting into the crowd to having a conversation with individuals.
These personalization systems are capable of analyzing real-time data from many different places, including social media, customer interactions, and browsing histories. This allows for instant adjustments to marketing efforts. This rapid response capability is a big contrast to traditional methods that take longer to process and adapt to changes. It's almost as if the engine has real-time reflexes.
One surprising aspect of AI-driven personalization is its potential to predict not only what a customer wants but also when they're likely to want it. By using predictive analytics, it can forecast the ideal time for promotions and offers. This anticipatory approach can lead to significant increases in conversions when customer interest is at its peak. It's like anticipating a need before the customer even realizes they have it.
It's been observed that companies using advanced personalization engines frequently see sales conversion improvements of over 20%. This increase really shows how impactful it is to directly address the specific needs of customers and make marketing efforts more relevant. It's a move from a "one size fits all" approach to a custom-tailored fit.
However, a significant hurdle is the complexity of integrating these systems into existing CRM systems. Many companies struggle to properly align their data infrastructure and analytical tools to fully benefit from AI-powered personalization. It's like trying to fit a new high-powered engine into an old car—it requires careful planning and modifications.
The engines are getting better at understanding evolving language trends and adjusting content accordingly. This ability to adapt allows businesses to maintain a contemporary feel that resonates with customers, boosting engagement. It's almost like the engine is learning the language of its users.
One of the less highlighted facets of these systems is the need to address data privacy considerations. With growing scrutiny over how data is handled, compliance with regulations is essential. This is pushing companies to develop ethical frameworks for how they gather and analyze customer information. It's a matter of both technological and ethical evolution.
Furthermore, these personalization systems can analyze groups of similar customers, identifying which segments respond best to particular marketing strategies. This data-driven approach informs future campaigns and refines targeting accuracy. It's like having a group of expert analysts providing insights into what works and what doesn't.
Finally, these personalization engines aren't just static tools; they include machine learning algorithms that constantly fine-tune their models based on ongoing customer interactions. This continuous learning process keeps personalization strategies fresh and relevant, greatly improving their effectiveness over the long term. The engine is continuously learning and evolving, just like the customers it aims to serve.
CRM Software in 2024 AI Integration Reshapes Customer Management Landscape - Voice Recognition Technology Streamlines Data Entry and Retrieval
Voice recognition technology is becoming a standard feature within CRM systems, simplifying how data is captured and accessed. Businesses in 2024 are utilizing this capability to make customer interactions smoother and automate routine data entry, freeing employees to handle more intricate issues. But, as these systems are integrated, companies have to carefully consider data correctness and user privacy. Voice-enabled CRM offers a user-friendly approach, but it also places pressure on developers to revise workflows for compatibility. While voice recognition promises efficiencies, the shift necessitates continuous scrutiny of its effects on customer management methods. It's a change that requires careful consideration.
Voice recognition technology is becoming increasingly integrated into CRM systems, offering a fresh perspective on how we handle data entry and retrieval in 2024. It's fascinating how quickly these systems can convert spoken words into text, often at speeds exceeding 160 words per minute. This speed advantage can be a real game-changer, allowing for faster updates to customer information and potentially reducing the time spent on mundane data entry tasks. While the accuracy of voice recognition isn't perfect, improvements in the underlying algorithms have pushed error rates down to around 5% in optimal environments. This higher level of accuracy means fewer mistakes in capturing customer interactions, which is crucial for maintaining data integrity.
One of the more interesting aspects of this technology is the hands-free nature of operation. Employees can now input data while simultaneously handling other tasks, making workflows more efficient. This could be particularly beneficial in scenarios where customer interactions are combined with tasks like managing physical documents or accessing customer files. It's intriguing how the algorithms are becoming more sophisticated in understanding natural language, even adapting to specific industry jargon and terminology. This adaptability makes the technology more useful across a wider range of businesses, and it's something that will likely continue to evolve as the technology matures.
The integration of voice recognition is also making CRM systems more accessible for employees with physical disabilities. This capability has the potential to foster a more diverse and inclusive workforce. Furthermore, the integration of voice recognition with IoT devices offers a new level of agility to sales and customer service teams, allowing them to access data in real-time and respond more quickly to customer inquiries. The ability to process multiple languages and dialects is another powerful aspect. This feature can streamline customer engagement across a variety of global markets without needing separate systems.
Researchers are also exploring the possibility of analyzing emotional tone within voice interactions. While still in its early stages, this functionality could potentially allow CRM systems to detect subtle cues in a customer's voice and adapt responses to create a more empathetic customer experience. Interestingly, some systems are incorporating machine learning to proactively flag missing data or inconsistencies during voice entries. This capability can help maintain data quality and minimize errors during data entry. Security is always a key concern when dealing with customer data, and voice recognition systems are starting to implement biometric authentication to ensure access is limited to authorized individuals. This development adds an additional layer of security to sensitive customer information, a feature that's sure to become more prevalent in the future.
It's clear that voice recognition technology is still evolving, but its impact on data entry and retrieval within CRM systems is undeniable. As the technology matures, it's likely to become even more integrated into our workflows, continuing to reshape how businesses interact with their customers and manage their data.
More Posts from :