AI-Driven Sales Development How Machine Learning is Reshaping Lead Qualification in 2024
AI-Driven Sales Development How Machine Learning is Reshaping Lead Qualification in 2024 - Machine learning algorithms revolutionize lead scoring accuracy
Machine learning algorithms are revolutionizing how we assess leads, significantly boosting the accuracy of lead scoring. Instead of relying on fixed rules and limited data points, these algorithms analyze vast quantities of information to predict a lead's likelihood of converting into a customer. This shift from static to dynamic scoring allows sales teams to identify previously unseen patterns in how potential customers interact with a business. This real-time insight offers a much more nuanced view of leads, allowing sales professionals to refine their strategies and maximize their efforts. Moreover, these algorithms learn continuously from past interactions and outcomes, leading to consistently improved lead scoring accuracy over time. By leveraging AI-powered tools for lead scoring, businesses can optimize their lead management processes, leading to more efficient operations and ultimately, better sales results. This evolution in lead scoring is not just about streamlining tasks; it's about using data to make better, more informed decisions throughout the entire sales lifecycle.
Machine learning algorithms are transforming how we assess leads, moving beyond basic, rule-based systems. They can sift through massive datasets of past lead interactions incredibly fast, revealing subtle patterns and meaningful cues that would be hard for humans to discern. This enhanced pattern recognition leads to significantly improved scoring accuracy.
While traditional scoring often relies on a limited set of pre-defined factors, machine learning allows for the incorporation of dynamic, behavioral data. This means incorporating things like how prospects interact with email campaigns or navigate websites, creating a much richer understanding of each lead. This multi-faceted view often unearths high-potential leads that might otherwise be missed, particularly those that don't perfectly fit pre-defined profiles.
One of the interesting aspects of these machine learning systems is their adaptability. They can learn and adjust to changing circumstances. As new data becomes available, the algorithms are refined, allowing businesses to remain flexible and responsive in fast-paced environments without constantly reconfiguring their entire scoring process. However, there's a potential pitfall here—we need to be cautious that the algorithms don't become overly reliant on historical data and fail to recognize truly novel situations.
Moreover, the algorithms can help reduce inherent human biases. Rather than relying on gut feelings or subjective assessments, these systems evaluate leads using quantifiable data and statistical analyses, striving for a more objective and fair approach. While we don't want to discount the value of human intuition and expertise, using these methods can help us identify potential blind spots in our evaluation process.
Furthermore, these AI-powered systems enable real-time lead score adjustments. By incorporating live data streams, they can respond to current market fluctuations or news events. This can offer sales teams instantaneous insights that inform their engagement and outreach strategies. However, we should be careful about over-relying on these dynamic updates, especially if the data quality or underlying signal is unreliable.
The impact of these changes can be significant. Research suggests that integrating machine learning into lead scoring can lead to substantial improvements in conversion rates and, potentially, a higher return on marketing investments. However, as researchers, we need to exercise caution and avoid drawing overly simplistic conclusions. We must carefully consider the nuances of different sectors and understand how these algorithms are trained and applied in each case. The overall success will depend heavily on the quality of the data used to train the models, and a careful analysis of potential biases introduced during the training process.
AI-Driven Sales Development How Machine Learning is Reshaping Lead Qualification in 2024 - Predictive analytics enhance buyer behavior forecasting
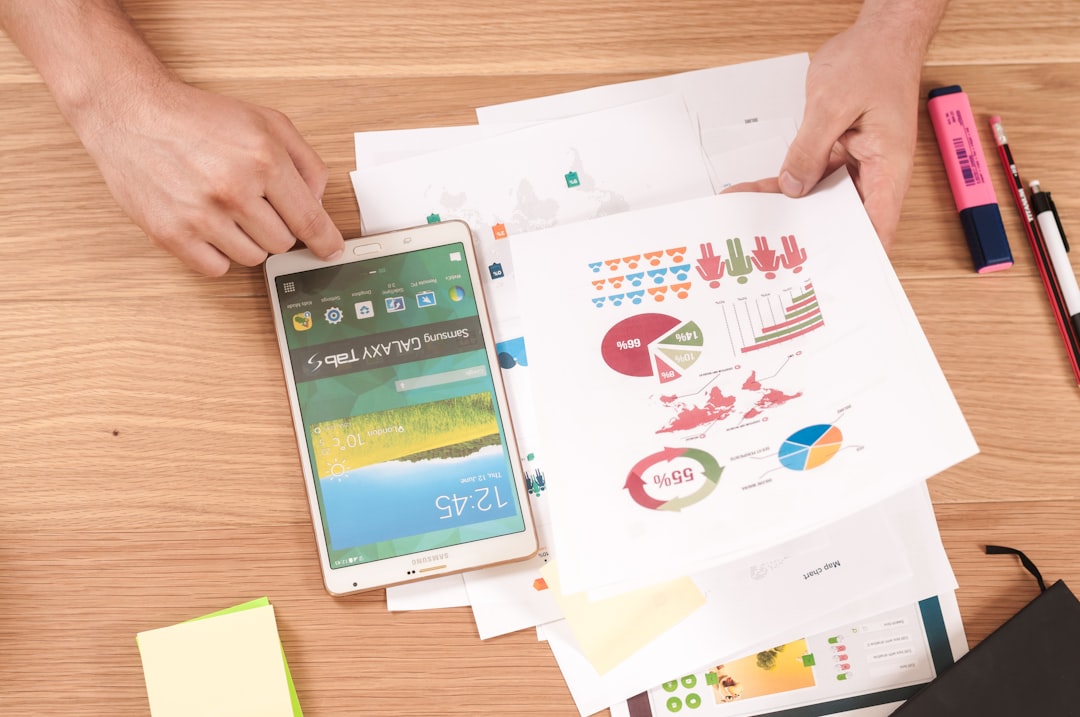
Predictive analytics is increasingly important for understanding how potential customers might act, a key part of AI in sales. By using past data and sophisticated machine learning methods, businesses can develop a better sense of what buyers will likely do, resulting in more accurate sales forecasts. This understanding of future buying patterns aids in optimizing inventory, mitigating the risk of either having too much or too little product on hand, thus improving efficiency. Furthermore, these predictive models can adjust as market trends evolve, allowing sales teams to make better decisions in real-time. However, relying too heavily on past data can be a limitation, as it might not anticipate completely new market shifts. While helpful, it's important to remember these predictive tools are built on what's happened before and might not always predict the unexpected.
Predictive analytics, powered by historical data and machine learning, offers a more refined approach to forecasting how potential buyers will act. It's essentially using past interactions and trends to predict future behaviors, which can be incredibly valuable in sales. We're not just talking about simple guesses here, though—the algorithms underpinning these systems can sift through huge amounts of data to uncover subtle patterns and connections that humans might miss. This deep dive into customer behavior allows businesses to understand what drives purchasing decisions and helps in making more effective decisions.
While it's still early days for widespread adoption, it appears that predictive analytics can significantly improve the accuracy of sales forecasting. This improved accuracy isn't just a nice-to-have; it can help businesses focus on the leads most likely to convert, which in turn can lead to better campaign ROI. However, there's a caveat here: we need to be mindful of the limitations of these tools. They're only as good as the data they're trained on. If the data is biased or unreliable, it can lead to skewed predictions, which can be problematic. For instance, if the dataset used to train the model primarily represents one customer demographic, the model might not accurately predict behavior in other groups.
The ability to predict buyer behavior shifts in real-time is fascinating. This adaptability can be crucial in markets where customer preferences change rapidly. Think about industries like fashion or consumer electronics – having the ability to immediately adjust strategies based on real-time insights can be a huge competitive advantage. But, as always with machine learning applications, it's important to be cautious about relying too heavily on predictions without carefully considering their context. It's not a magic bullet that will solve all sales forecasting problems.
One of the more interesting research angles here is the integration of natural language processing (NLP) into predictive analytics. Being able to gauge customer sentiment through online conversations—social media posts, product reviews, etc.—can significantly enrich the profiles of buyers. It adds another dimension to our understanding of customer behavior, which can inform better outreach and engagement strategies. However, there's a whole other set of ethical concerns that we need to consider with this type of data collection and analysis.
Overall, predictive analytics is a field that's ripe with research potential. There's still a lot we don't understand about the complex interplay between historical data, algorithm design, and actual behavior prediction. It's critical to approach this technology with a healthy dose of skepticism while recognizing its potential value for refining sales processes.
AI-Driven Sales Development How Machine Learning is Reshaping Lead Qualification in 2024 - AI-powered chatbots streamline initial lead interactions
AI-powered chatbots are becoming central to streamlining the initial interactions with potential customers, improving engagement right from the start. They utilize natural language processing and machine learning to deliver immediate answers to questions, gather important information about leads, and guide them through early stages of the sales process. Automating these first interactions simplifies things for both the customer and the business, and helps better qualify leads by understanding their needs and whether they are truly interested in a purchase. While the speed and efficiency of these bots are appealing, there is a risk they could miss the subtleties of human conversation, which can lead to impersonal experiences or misunderstandings of what a customer actually wants. Finding the right balance between the speed of AI and the value of human communication is key. The goal is for chatbots to enhance, not replace, the vital interactions that build genuine relationships with customers.
AI-powered chatbots are transforming how businesses initially interact with potential customers. They can be available 24/7, instantly responding to inquiries, which is something humans can't easily achieve. This constant availability not only boosts engagement but also captures leads outside normal business hours, expanding the potential customer pool.
Research indicates that using AI chatbots in the initial stages of lead interaction can substantially increase the quality of those leads. Some studies show that qualification rates can improve by as much as 70%. The reason for this improvement seems to be the chatbot's ability to gather a lot of information from a lead quickly and efficiently.
Chatbots can analyze the tone of a conversation using sentiment analysis, allowing them to adapt their responses and keep the interaction going. This personalized touchpoint from the very start can significantly impact how a potential customer perceives a brand.
One of the most striking advantages of AI chatbots is their ability to handle numerous conversations simultaneously without any reduction in performance. This is something that humans struggle with. This scalability is especially beneficial for businesses experiencing a large influx of inquiries, enabling them to manage it all without necessarily hiring a massive team.
Through the application of machine learning, chatbots can continuously learn from previous conversations and improve their responses. As they process more data, they refine their interactions, leading to more engaging and successful conversations with potential customers.
Furthermore, these chatbots can be programmed to track how engaging a particular lead is. Based on specific criteria, they can flag leads for human follow-up, allowing sales teams to focus their efforts on the most promising prospects. This helps streamline the entire lead qualification process.
The design of chatbot conversations often tries to mimic successful human interactions. This can include using humor and expressing empathy, hoping to make the experience more relatable for the potential customer. However, it also raises interesting questions about transparency—when an AI is acting like a person, should it be clear that it's not a real person?
When we connect chatbots with CRM systems, a feedback loop is established. User interactions inform lead scoring and qualification models. This means the chatbots’ real-time data can be combined with historical lead data for constant improvement.
Some AI chatbots can manage shifts in topic or user intent, maintaining the flow of the conversation. This flexibility is helpful because it reduces frustration for leads and improves the overall quality of the interaction.
Despite their advantages, chatbots can occasionally lead to issues due to misinterpretations or limitations in understanding context. These miscommunications not only hinder the lead qualification process but can also negatively affect customer experience. This highlights the importance of continuously monitoring and improving these AI systems.
AI-Driven Sales Development How Machine Learning is Reshaping Lead Qualification in 2024 - Real-time lead qualification adapts to market changes
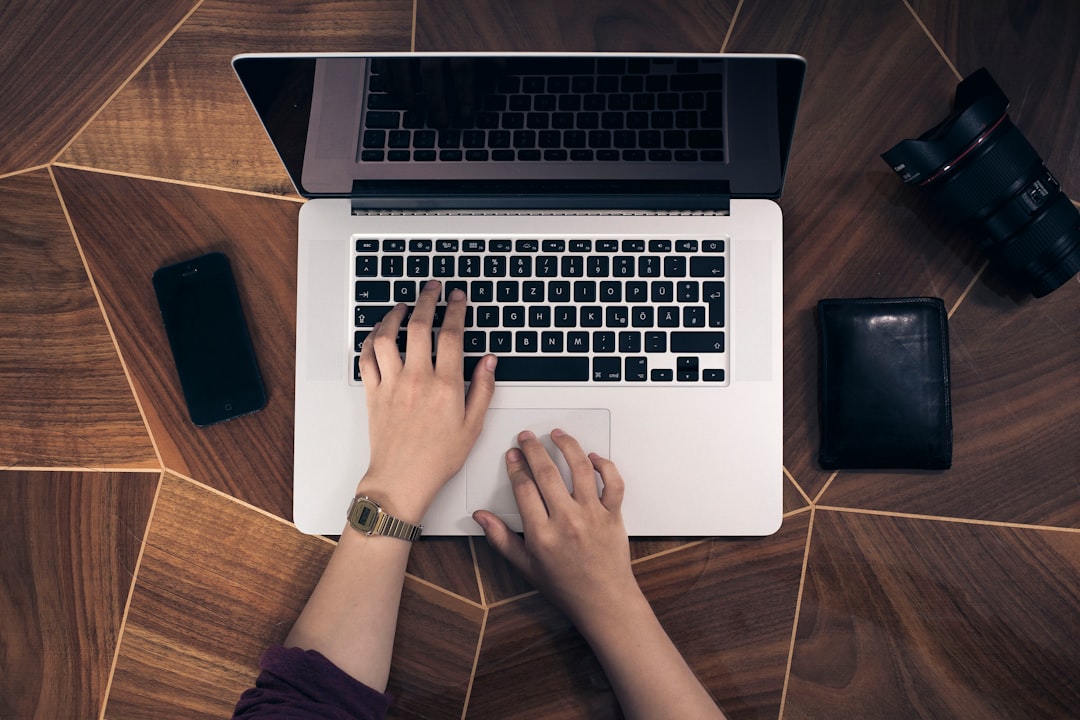
The evolving landscape of sales and marketing in 2024 demands that lead qualification processes be highly adaptable to changing market conditions. AI and machine learning are making this possible by enabling real-time adjustments to lead scoring. As new data emerges, AI systems can refine their evaluation criteria, allowing businesses to better understand and respond to shifts in customer behavior and overall market dynamics. This is especially valuable in industries where trends change quickly, ensuring companies don't fall behind.
However, relying solely on algorithms that learn from past data can be a double-edged sword. There's a risk that these systems might overemphasize historical patterns and struggle to recognize truly new situations or unforeseen changes. This potential blind spot requires careful attention and a balance between AI's inherent flexibility and the need for human oversight in interpreting data. Successfully leveraging real-time lead qualification in 2024 hinges on navigating this dynamic and ensuring that AI's adaptability doesn't overshadow a thoughtful approach to interpreting information.
The ability of AI to adapt lead qualification in real-time to market fluctuations is a significant development. These systems don't simply rely on broad historical trends; they can react within minutes to shifting consumer sentiment or unforeseen events. This agility allows businesses to refine sales strategies on the fly, rather than waiting for data to be processed and analyzed in batches.
Furthermore, these systems analyze much more granular details than traditional approaches. They can detect subtle behavioral cues like mouse movements or how long a prospect spends on a particular part of a webpage to get a better sense of their intent. This often leads to insights that traditional lead scoring techniques miss, providing a more nuanced understanding of individual leads.
Interestingly, these AI models are not only adept at identifying positive patterns but also learn from errors. By recognizing leads that initially seem promising but fail to convert, they refine their predictive models to become more precise over time. This continuous learning process is crucial to keep pace with evolving market dynamics.
However, one challenge with real-time systems is managing the immense volume of data available. These algorithms are designed to filter out the noise and focus on the signals that are most indicative of purchase intent. Effectively separating the essential information from distractions is crucial for generating useful insights.
Adding another layer of complexity, real-time qualification can factor in external events like economic reports or breaking news that may influence purchasing behavior. This contextual awareness is an advantage that older qualification methods lacked.
Many of these systems don't update their scoring criteria at fixed intervals, but instead adjust continuously. This allows them to react to sudden shifts in the competitive landscape or consumer behavior, making them especially useful in rapidly changing industries.
Additionally, the integration with social media is a fascinating development. AI can track and analyze real-time public sentiment around a brand or product and adjust lead scoring accordingly. This is especially useful for brands heavily reliant on social media presence.
While this rapid adaptability is beneficial, it can also lead to misallocation of resources if it reacts too strongly to fleeting trends. Human oversight is essential to ensure that the AI's actions are aligned with long-term business goals.
Different industries have distinct purchasing patterns, which is something that real-time lead qualification can adapt to. The ability to customize these systems based on industry-specific signals can significantly improve the accuracy of the process compared to generic, one-size-fits-all solutions.
Finally, using real-time data to qualify leads raises important ethical considerations. Data privacy and the responsible handling of customer information are paramount. Finding the balance between leveraging advanced analytics and respecting user privacy is crucial for maintaining trust while employing these powerful tools.
AI-Driven Sales Development How Machine Learning is Reshaping Lead Qualification in 2024 - Ethical considerations in AI-driven sales processes emerge
The increasing use of AI in sales processes, particularly in lead qualification, brings forth a new set of ethical considerations. While AI-powered systems can improve lead scoring accuracy and predict buyer behavior with greater precision, they also raise concerns about how we handle data. Questions around data privacy, potential biases built into algorithms, and the transparency of AI interactions with customers are becoming central to the conversation. It's becoming clear that businesses need to consider the broader implications of these technologies and establish ethical guidelines for their use. This means putting thought into how they collect and utilize data while ensuring these systems do not inadvertently discriminate against certain groups.
Furthermore, there's a growing acknowledgment that AI isn't a replacement for human interaction. Blending AI's capabilities with the unique strengths of sales professionals is vital. Striking the right balance between automation and the complexities of human communication—such as empathy and understanding—will be crucial in building trust and maintaining positive relationships with customers. The discussion surrounding ethical AI in sales is ongoing and critical for mitigating risks and building confidence in these emerging technologies. We must ensure AI tools enhance, rather than diminish, the overall experience for both the customer and sales professionals.
As AI increasingly shapes sales processes, several ethical concerns come into sharper focus. For instance, the algorithms we train on historical data can inadvertently inherit biases present in that data, potentially leading to discriminatory practices in lead scoring or customer targeting. This highlights the importance of scrutinizing the data used to train these systems and understanding how past prejudices might influence future outcomes.
The use of AI in lead qualification also raises questions about informed consent. Are customers fully aware of the extent to which their data is being collected and analyzed by these systems? Understanding the boundaries of data usage and ensuring transparency in how this information is utilized is crucial to fostering trust with customers.
Transparency itself becomes a critical ethical challenge when interacting with AI-powered sales systems. If customers are unaware they are communicating with a machine instead of a human, it can undermine the authenticity and trust built during brand interactions. This ambiguity necessitates clear communication about the role of AI in sales interactions.
Furthermore, while AI aims to optimize sales strategies, it can raise concerns about potentially prioritizing profit over customer well-being. Sales strategies that solely focus on maximizing profit through the manipulation of consumer behavior raise ethical questions about responsible data usage and the potential for exploitation.
The reliance on historical data can create a feedback loop that reinforces existing trends, which could stifle innovation. Novel customer preferences or emerging trends might not be adequately reflected in data sets trained on past behavior, leading to a sort of stagnation in the market.
Analyzing customer sentiment on social media through AI tools allows for rapid adjustments in sales strategies. However, this raises the ethical dilemma of whether actively responding to public opinion crosses a line into manipulative consumer behavior. Is there a point at which AI-driven responses become unduly influential on customer decisions?
The dynamic nature of real-time adjustments in sales strategies can also negatively affect brand reputation, especially if businesses react erratically to fleeting market trends. Impulsive responses that are not carefully considered in relation to long-term brand values and customer loyalty can damage brand image and consumer trust.
Developing clear ethical frameworks for AI in sales is still an evolving field. Balancing the profit-driven motivations of businesses with responsible data practices is a major challenge for organizations. The need for industry-wide standards and regulations to guide these practices becomes increasingly important in this context.
The power of AI to identify and predict customer behavior through continuous data analysis also raises privacy concerns. When sensitive personal information is collected and utilized without explicit user consent, it can lead to a breach of trust and potential privacy violations.
As AI systems become more complex and sophisticated, their decision-making processes may become less transparent. This lack of transparency can hinder efforts to ensure accountability for ethical violations or algorithmic discrimination, potentially eroding public trust in both AI and the businesses that deploy it. Maintaining a balance between technological advancement and ethical considerations is essential to ensure that AI enhances human experience rather than compromising it.
More Posts from :