AI-Driven Contract Analytics How Machine Learning is Transforming Legal Risk Assessment in 2024
AI-Driven Contract Analytics How Machine Learning is Transforming Legal Risk Assessment in 2024 - NLP advancements in contract clause identification
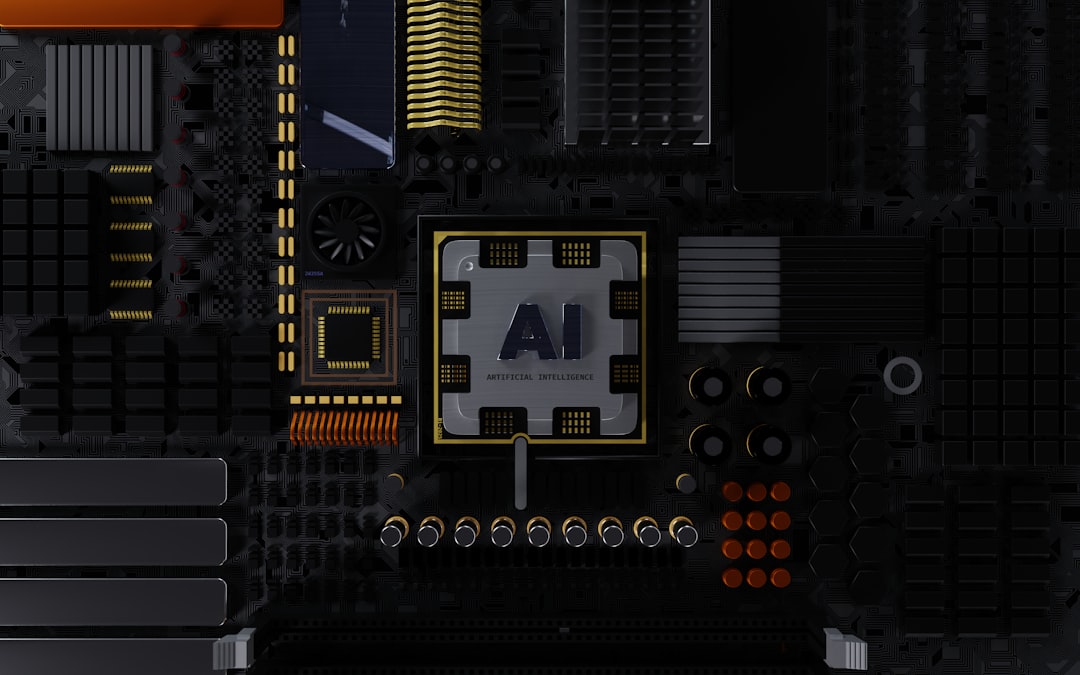
The field of Natural Language Processing (NLP) has seen a surge in capabilities, particularly in pinpointing and dissecting specific contract clauses. NLP techniques, fueled by advanced machine learning models, are automating the discovery of potential risks embedded within contracts. This automated approach enables businesses to process and understand massive amounts of contract data much more efficiently. Beyond simply speeding up review, NLP helps bolster compliance efforts by more accurately flagging high-risk clauses and contractual commitments.
As NLP progresses, it's increasingly clear that it's shifting the work of contract analysis. Legal experts are freed from the drudgery of manual contract review, allowing them to dedicate their energies towards higher-level strategic decisions.
However, we must be cautious about overdependence on automated solutions. There's a risk that the subtleties and nuances of contract language could be missed if we rely too heavily on AI alone. While automation brings clear benefits, a balanced approach is necessary to ensure accurate and effective contract analysis.
The field of NLP is making significant strides in automatically recognizing crucial clauses within contracts. We're seeing accuracy rates climb above 95% in some cases, which is dramatically lessening the time lawyers spend manually reviewing documents. This progress is largely due to the rise of transformer-based models in machine learning, which possess an unprecedented ability to grasp the context and meaning within contractual language.
Interestingly, some platforms are utilizing unsupervised learning, meaning they can categorize clauses without needing huge datasets of manually labeled examples. This makes it much easier for organizations to begin using these tools for legal analytics. Beyond simply finding explicit clauses, researchers are exploring ways to make NLP understand the implicit obligations often buried in vaguely worded contracts. This has the potential to reveal hidden risks we might not otherwise catch.
Another exciting avenue is transfer learning. This lets us take NLP models trained on one area of law and adapt them for use in another, needing significantly less retraining. Expanding the reach of these tools, NLP is now equipped to decipher contracts written in various languages, paving the way for global legal risk assessments.
The integration with blockchain is still in its early stages, but it hints at a future where smart contracts can execute autonomously once NLP has identified the appropriate conditions. Ensuring transparency is crucial in this space, which is why explainable AI methods are being incorporated into NLP algorithms. This helps lawyers understand how and why a clause is being flagged, a critical concern for legal professionals.
Looking ahead, we're seeing features like real-time contract review emerging. These could offer instant feedback on revisions, with NLP instantly flagging potential legal problems. Furthermore, some advanced models are beginning to integrate legal domain knowledge, letting them grasp jurisdiction-specific vocabulary and tailor risk assessments to regional legal contexts. This trend towards greater specialization could lead to even more refined and accurate legal analysis going forward.
AI-Driven Contract Analytics How Machine Learning is Transforming Legal Risk Assessment in 2024 - Machine learning algorithms for risk prediction in legal agreements
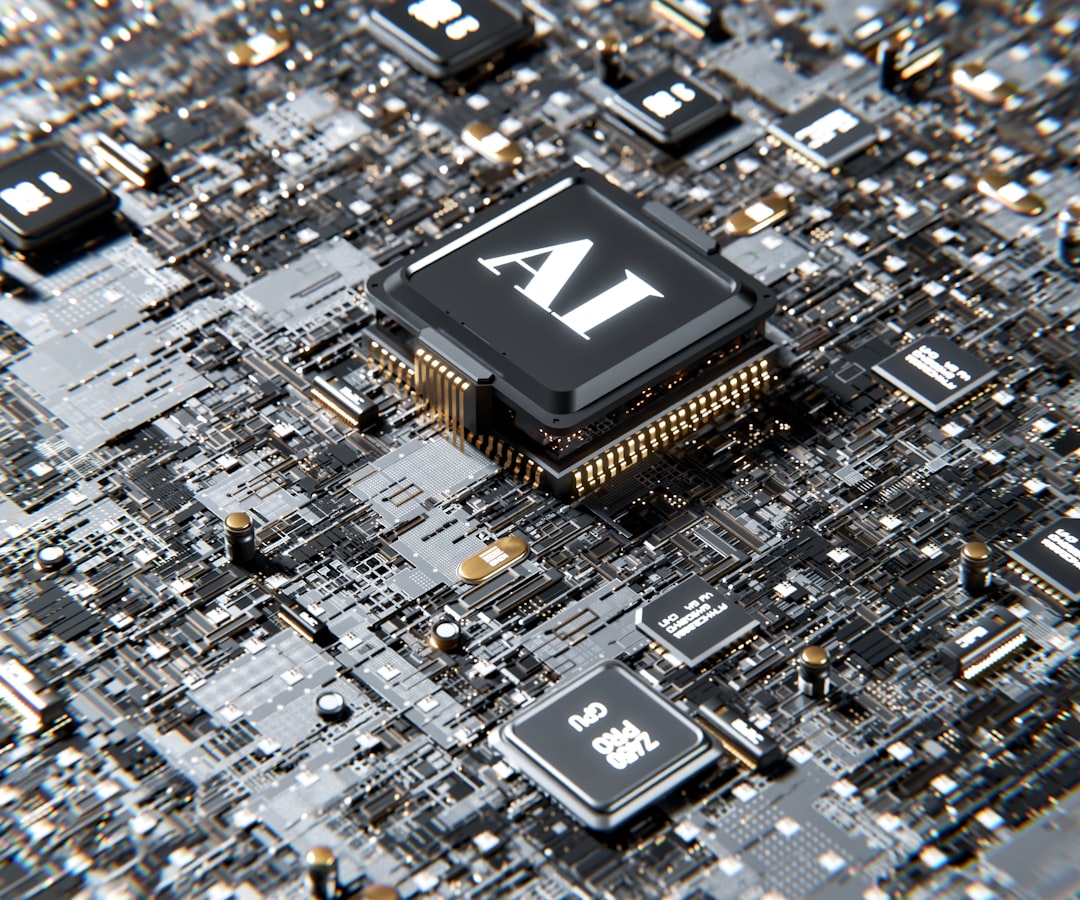
Machine learning algorithms are increasingly being used to predict risks within legal agreements. These algorithms can analyze vast quantities of contract data, uncovering intricate patterns and relationships that would be difficult or impossible for humans to spot through traditional methods. This leads to more accurate assessments of potential legal risks and the likelihood of future litigation.
By automating the process of risk identification, machine learning can help legal professionals quickly flag potential compliance issues and problematic contractual clauses early on. This allows for proactive mitigation of risks and a more efficient management of the entire contract lifecycle.
Yet, it's crucial to acknowledge that relying solely on automated systems can lead to unintended consequences. The complex and nuanced language of legal agreements can be difficult for AI systems to fully grasp, and there's a risk that crucial details might be overlooked if humans are not involved in the process. While machine learning significantly improves the speed and accuracy of risk analysis, human expertise and judgment remain vital to ensure that the subtleties of legal language are properly interpreted.
The field of legal risk assessment is undergoing a transformation thanks to these algorithmic advancements. Though the possibilities are significant, it's essential to maintain a balanced approach, incorporating human oversight into the automated risk prediction process. This ensures that the benefits of machine learning are realized without compromising the accuracy and integrity of legal analysis.
Machine learning algorithms are increasingly being used to predict risks within legal agreements, essentially trying to anticipate potential legal battles. These algorithms, often combined with natural language processing (NLP), can automatically identify and analyze risk factors hidden within contracts. This shift is making legal work more efficient and streamlined, changing how lawyers approach their tasks.
While traditional methods rely on predefined rules, machine learning models can detect intricate patterns within data that we might not have even considered. This can lead to more accurate risk predictions and insights. These algorithms are adept at processing massive volumes of contract data to interpret risks, aiding legal professionals in identifying compliance issues or unfavorable contract terms early in the process.
These methods essentially leverage historical case data to predict future outcomes, offering valuable strategic insights for lawyers and their clients. It's essentially applying data science to legal strategies, reshaping legal research and decision-making. We are seeing a significant reduction in human error in contract analysis with AI-driven tools, enhancing the accuracy of risk assessments.
However, the integration of AI into legal systems is not without challenges. It represents a major change in how legal work has been traditionally performed, raising questions and uncertainties. Generative AI models are starting to impact the industry, necessitating the development of proper regulations to address potential future risks.
One intriguing avenue is incremental learning. It allows these algorithms to continuously learn from new data without needing a complete retraining cycle, allowing for better adaptation to changing legal landscapes and emerging risks. Another area is predicting potential contract breaches or disputes, offering a more proactive approach to risk management. Some systems are even getting good at understanding the intent and meaning behind language, not just the literal words, which can uncover hidden risks buried in vaguely written clauses.
While AI tools can reduce contract review time, sometimes by a lot, the impact on legal billing practices is still being explored. Additionally, these tools are being used across multiple jurisdictions, making it possible to pinpoint legal risks related to regional laws. The combination of AI and traditional tools is common right now, offering some benefits, but also raising integration concerns.
It's important to remember that even the most sophisticated AI isn't perfect. Human oversight remains essential to verify the AI's results, highlighting the need for a cooperative, rather than entirely automated, approach. This whole field raises some ethical questions around data privacy and potential biases in the training data that could impact outcomes.
The trend towards more autonomous contracts, or smart contracts, is also fueled by advancements in AI. However, the legal implications of such automated contract enforcement are still being understood. The performance of these risk prediction algorithms is heavily dependent on the quality of the data used to train them. This underscores the importance of solid data management practices within legal analytics. Overall, while this field is new and still developing, AI and machine learning have a lot of potential for enhancing legal risk assessment, though we must remain cautious and aware of the implications.
AI-Driven Contract Analytics How Machine Learning is Transforming Legal Risk Assessment in 2024 - Automated due diligence processes using AI in M&A transactions
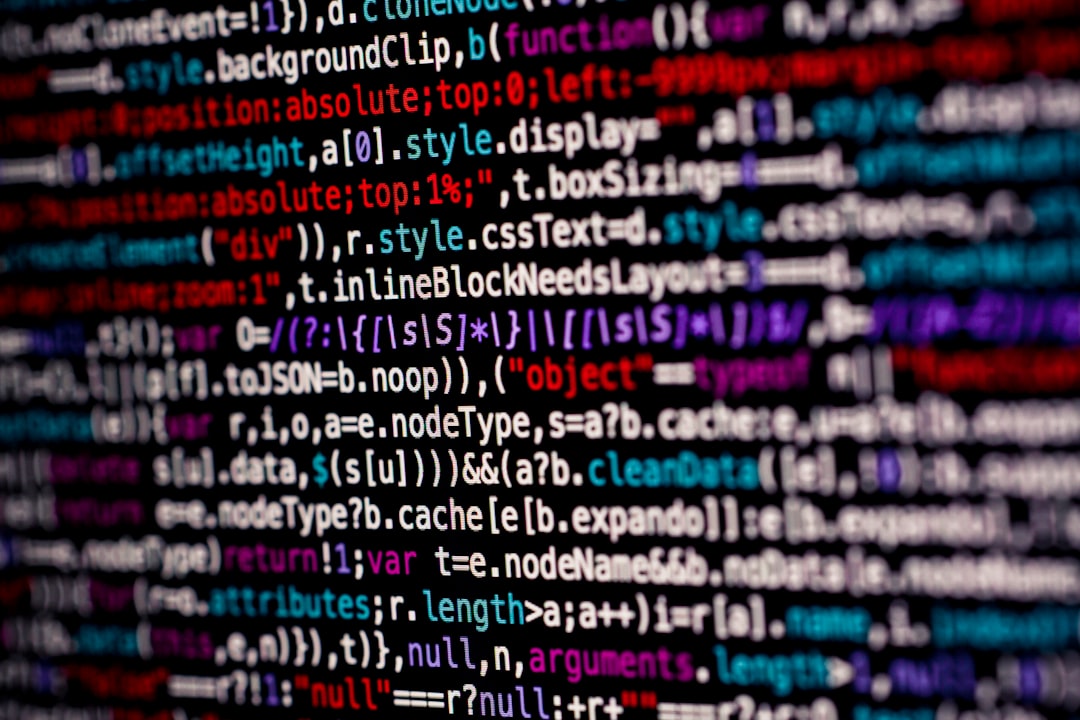
Artificial intelligence is rapidly changing how due diligence is conducted in mergers and acquisitions. AI-powered tools are automating many parts of the process, from gathering information to spotting potential problems. This allows for quicker and more in-depth analysis of potential deals, compared to the more manual and time-consuming traditional methods. AI can scan massive amounts of data, identifying key issues and understanding how the businesses might work together after a merger more efficiently.
However, we need to be careful about relying too heavily on automation. Legal language and the specifics of each situation can be complex, so human oversight is still needed to make sure the due diligence process is thorough and accurate. While AI greatly speeds up and improves the due diligence process, it's not a replacement for human expertise in this crucial stage of a deal.
AI is reshaping how due diligence is handled in mergers and acquisitions (M&A), particularly in speeding up the process and improving the accuracy of deal assessments. AI tools are automating tasks like data collection, analysis, and risk identification. For example, some systems can analyze thousands of loan agreements in seconds, a task that used to take a massive amount of time manually.
We're seeing a trend of using AI to improve the depth and breadth of the M&A process. The idea is to use data analysis to support synergy models and flag any issues before agreements are finalized. The potential for AI and machine learning to examine massive datasets is making it possible to do due diligence in ways humans just can't. These AI tools effectively mimic human analysis, but at a faster pace and with improved accuracy.
The benefits of this automation are clear: it frees up valuable time and resources. Businesses can then focus their efforts on other important parts of the M&A process. Furthermore, it helps make things more consistent and detailed, for tasks like document review and risk assessments. It's becoming increasingly common for companies to leverage AI in their M&A strategies, not just in the execution stage, but even from the initial planning phases.
The shift to virtual data rooms (VDRs) coupled with AI has really changed how due diligence is done, making it significantly more efficient and effective. While there are potential benefits, it's still important to remain cautious. Over-reliance on these tools could mean missing subtle details in the language of contracts. It's essential to find a balance, using AI as a tool to enhance the process, but not eliminating the critical role of human judgment in ensuring accuracy. We are just starting to see the impact of these tools in this area, and how they may continue to change M&A transactions in the years to come.
AI-Driven Contract Analytics How Machine Learning is Transforming Legal Risk Assessment in 2024 - Real-time contract performance monitoring through AI analytics
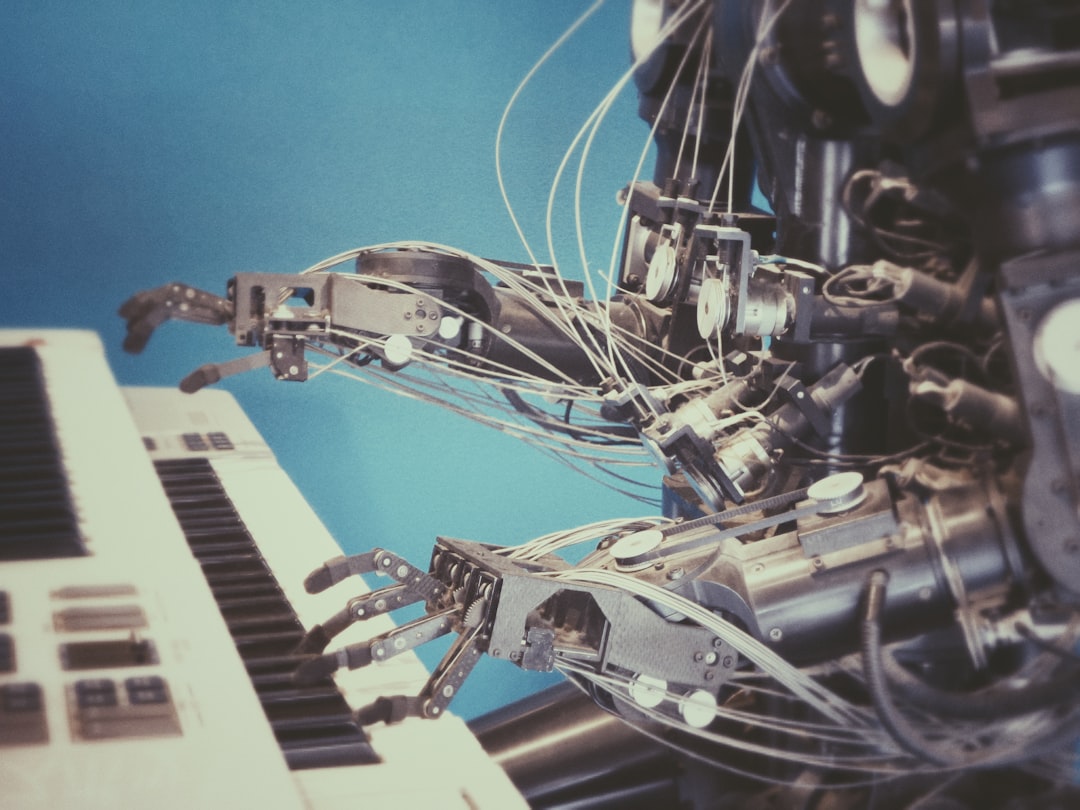
Real-time contract performance monitoring, powered by AI analytics, offers a dynamic approach to managing contractual obligations. AI can continuously analyze contract data, quickly pinpointing instances where performance falls short or compliance issues emerge. This proactive approach, enabled by AI, has been shown to reduce compliance problems significantly, potentially by as much as 40%, while boosting efficiency. By incorporating predictive analytics, AI can anticipate potential future problems, allowing for proactive steps to prevent contract disputes and related risks. This approach improves the overall contract management process, leading to better transparency and accountability.
The ability of AI to offer real-time insights into contract performance facilitates swift interventions when needed, leading to a more responsive contract lifecycle. However, it's important to acknowledge the potential for over-reliance on AI in this context. The complex and nuanced language found in legal agreements might still present challenges for even the most advanced AI systems. This means humans remain a critical part of the process, ensuring that the fine points of contract language are not overlooked, and the potential for error in the automation process is acknowledged. Ultimately, striking a balance between AI's strengths and the need for human expertise is essential to achieve the desired results in contract performance monitoring.
The use of AI analytics for real-time contract performance monitoring is gaining traction, with many organizations seeing a reduction in contract cycle times, sometimes by as much as half. This rapid feedback loop allows companies to react quickly to shifts in the market or changes in compliance requirements. It's fascinating how these tools aren't just flagging risks, but also predicting the chance of contract disputes or breaches by analyzing historical performance. This predictive aspect provides a valuable lens for anticipating potential issues.
One unexpected application is benchmarking contract performance against industry standards. This allows companies to see where their performance might be lagging and adjust their strategies. Some newer AI models are moving beyond the legal text itself and incorporating data from external sources, like court rulings and regulatory changes. This creates a much richer view of contract obligations and risk.
There are some really interesting AI approaches emerging. For example, platforms that leverage machine learning to generate dynamic risk profiles for contracts, which then adapt as new information surfaces. This allows legal teams to be much more proactive. However, a critical challenge is ensuring the quality of the data fed into these systems. Inaccurate initial contract information can lead to incorrect conclusions, which emphasizes the importance of strong data governance.
I find the use of AI for sentiment analysis during contract negotiations particularly intriguing. It's potentially able to predict the probability of reaching an agreement by understanding the tone and feelings of the parties involved, offering a glimpse into stakeholder satisfaction before the ink is even dry on the contract. These analytics tools are starting to be paired with communication platforms, letting stakeholders get alerts about deviations from contract performance instantly. This kind of real-time collaboration across departments like legal, procurement, and operations seems like it could improve how organizations manage their contracts.
Additionally, some systems use predictive analytics to spot patterns hinting at worsening vendor relationships. This ability to intervene early could be crucial for companies trying to avoid negative impacts to their operations. It's surprising how, in some cases, the more organizations automate contract monitoring with AI, the more hidden compliance issues they discover. This points to a broader theme: the potential of AI to unlock much greater transparency within organizations.
While the use of AI in this area is promising, the field is still relatively new and in development. We must remain aware of the limitations and ensure that humans are part of the loop. However, it's clear that this space has the potential to revolutionize contract management and provide insights that were not previously available.
AI-Driven Contract Analytics How Machine Learning is Transforming Legal Risk Assessment in 2024 - Integration of blockchain technology with AI for smart contract management
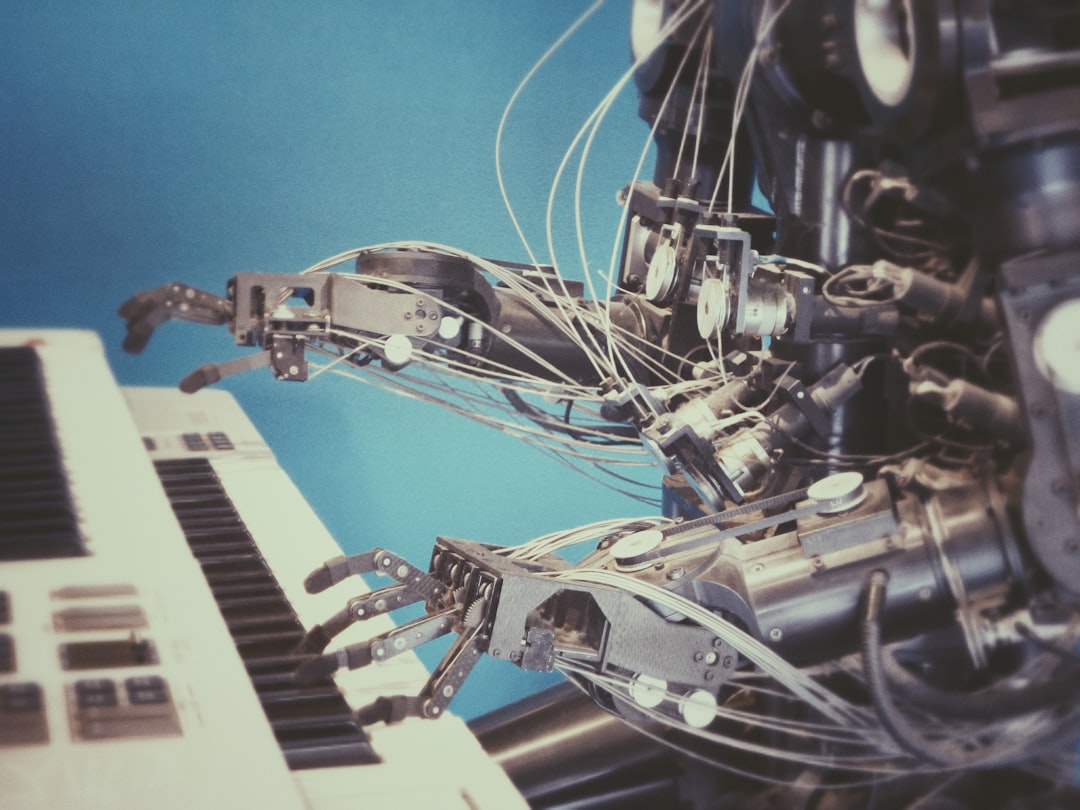
The blending of blockchain technology and artificial intelligence (AI) to manage smart contracts represents a significant step forward in automated and legally compliant transactions. Smart contracts gain the ability to handle more complex tasks by combining the decentralized trust inherent in blockchain with the analytical prowess of AI. This integration streamlines operations across diverse fields like finance and supply chains while introducing greater transparency and security than traditional contractual approaches. However, the merger of these two areas also presents hurdles regarding data integrity, security threats, and the need for thorough reviews of these evolving systems. As this technology continues to evolve, it's expected to revolutionize business practices, but it also necessitates careful consideration of the appropriate balance between automation and human oversight in the management of contracts.
Blending blockchain technology with artificial intelligence (AI) for managing smart contracts offers the potential to dramatically speed up contract execution, potentially down to milliseconds. This combination allows contracts to automatically execute when pre-defined conditions are met, a huge leap forward from the often-sluggish pace of traditional contract processing.
One key advantage of using blockchain for smart contracts is the inherent tamper-proof nature of the data. Because contracts built on blockchain are resistant to changes, any alterations to the contract terms create a traceable audit trail. AI systems can then automatically verify these modifications, ensuring that no adjustments slip under the radar.
Early adopters of AI in smart contract management have reported error reductions in contract compliance of up to 30%. AI algorithms can independently confirm contract stipulations against real-time blockchain data, instantly identifying contradictions or violations.
While promising, smart contracts still have some hurdles to overcome, particularly the complexities of coding and the requirement for extremely precise and unambiguous language in the contracts themselves. Programming errors can have unintended consequences, highlighting the importance of continued human oversight, even in a fully automated system.
AI can significantly bolster the analytical capabilities of blockchain by using past data to anticipate potential legal risks associated with a contract's execution. This predictive analysis can help spot parties likely to default or flag potential compliance issues, enabling proactive risk management.
The marriage of AI and blockchain enables automated triggering of events within contracts. For instance, payments could be instantly released upon fulfilling conditions verified by AI assessments, which could streamline financial transactions across various sectors.
Surprisingly, this combined approach allows for the creation of dynamic contracts. These contracts can adjust in real time based on AI insights derived from continuous data analysis. Contract terms could be fine-tuned based on performance metrics tracked on the blockchain.
Legal professionals are increasingly turning to AI to audit smart contracts hosted on blockchain networks, ensuring adherence to relevant regulations. This growing field illustrates AI's crucial role in governance and legal oversight.
Combining AI and blockchain could potentially lead to "self-executing" conflict resolution within contracts themselves. Advanced algorithms could analyze circumstances leading to disputes and autonomously determine resolutions based on predefined clauses within the contract.
Interestingly, early AI and blockchain implementations have encountered scalability challenges. The intricate nature of maintaining both systems in harmony means engineering dependable frameworks remains a work in progress. This requires continued advancements in both fields to ensure seamless integration.
AI-Driven Contract Analytics How Machine Learning is Transforming Legal Risk Assessment in 2024 - Ethical considerations and bias mitigation in AI-driven legal assessments
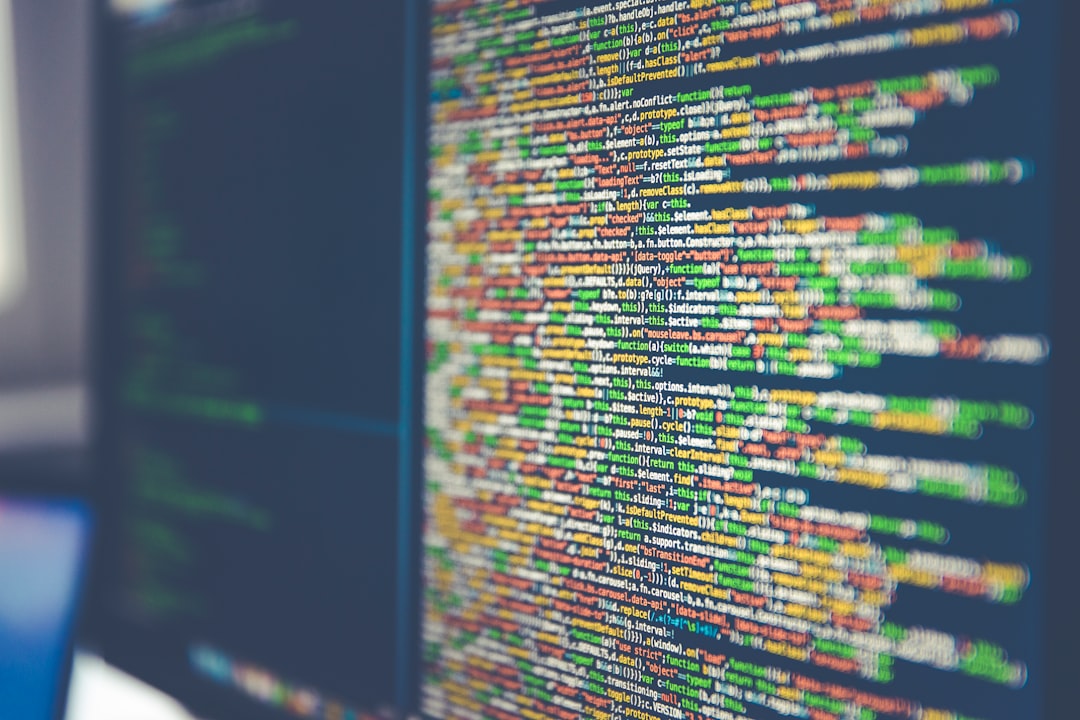
The integration of AI into legal assessments, particularly within contract analytics, is rapidly changing the field. However, this progress necessitates a strong focus on ethical considerations and bias mitigation. As AI algorithms learn from vast datasets, there's a potential for them to inadvertently perpetuate or amplify existing biases present within the legal system itself. This can lead to unfair or discriminatory outcomes, especially when these systems are used for tasks like risk prediction and decision-making.
Addressing this risk requires a proactive approach to bias mitigation. Developers and legal professionals must implement strategies to identify and eliminate potential biases embedded in the AI models. Transparency in how these algorithms function is also crucial, as it allows for greater scrutiny and the identification of potential issues. Moreover, robust ethical frameworks centered on fairness, accountability, and transparency are necessary to guide the development and implementation of AI in legal settings.
While AI-driven solutions offer compelling advantages in terms of speed and efficiency, it's vital to acknowledge that human oversight and judgment remain indispensable. AI should be viewed as a tool to assist human experts, not to replace them. Continuously evaluating the ethical implications of AI-driven legal assessment is crucial, ensuring that these advancements serve justice and fairness, not perpetuate inequality.
When we use AI to analyze legal documents and make assessments, we need to think carefully about the ethical implications. One of the big concerns is that the AI might be biased. If the algorithms are trained on historical data that reflects existing societal biases, they can end up perpetuating those biases in their assessments. For example, an AI trained on historical legal data might unfairly favor one demographic group over another, which is clearly not okay in a legal setting where fairness is paramount.
Even with the rapid advancements in AI, it's clear we still need a healthy dose of human oversight. If the AI's interpretation of a legal document is faulty, it could lead to bad recommendations, and that's where a legal professional's insight comes in. They can help make sure the AI's findings are put into context and any potential problems are understood.
The quality of the data used to train these AI models is crucial. If the data is inaccurate or out-of-date, the AI is likely to generate unreliable risk assessments. It really emphasizes how important proper data management practices are when implementing these AI solutions.
Another complex issue is accountability. If an AI makes a mistake and a bad decision is made, who is responsible? Is it the developers who built the system, the people who are using it, or the AI itself? The line gets blurry, and it's a significant challenge to address in a legal context.
There are some unexpected risks to consider when automating parts of legal work. While it's great that AI can help reduce workload, there's also a danger that it could make legal professionals overly reliant on technology and lead to complacency. This could cause them to overlook significant risks that they might have otherwise caught if they were paying closer attention.
AI systems aren't always great at understanding the nuances and cultural contexts of legal language. Contracts can use extremely specific and technical language that might be misinterpreted by the AI. This leads to questions about how well these systems can accurately capture the actual intent behind a contract.
As AI technology matures, so do the rules and regulations around it. Legal professionals need to stay on top of these changes to make sure they're following the rules when using AI-powered tools. It adds another layer of complexity to the implementation of these systems.
The black-box nature of some AI models is concerning. When AI makes a decision or flags an issue, it's not always clear how it reached that conclusion. Legal stakeholders need more transparency so they can better understand how the AI is working and what it's basing its judgments on. This is leading to increased calls for "explainable AI" that allows us to better grasp the decision-making process.
It's interesting that even the more advanced neural networks are not immune to biases. They can inadvertently learn from biases present in the training data, which then leads to inaccurate risk assessments that unfairly impact specific individuals or groups.
The best way forward is likely to be a blend of AI and human expertise. By combining AI's ability to process massive amounts of data with a human's ability to interpret the context and nuances of legal language, we can potentially build a more equitable and effective legal environment. It's all about striking a good balance.
More Posts from :