7 Critical Metrics for Evaluating Workflow Management Software Performance in 2024
7 Critical Metrics for Evaluating Workflow Management Software Performance in 2024 - Task Completion Speed Using Time Tracking Data and Lead Time Analytics
Gauging how quickly tasks are completed relies heavily on time tracking and analyzing lead times. This data provides a clear picture of workflow productivity and highlights areas ripe for improvement. Comparing the actual time taken for a task versus the initial estimate allows teams to spot bottlenecks and roadblocks. Understanding metrics like cycle time, the pure active work duration from start to finish, offers insights into specific areas for process tweaking. Lead time, on the other hand, gives a comprehensive view of the entire task duration, including any delays or waiting periods. The ability to visualize this data through dashboards and automated time capture solutions is increasingly crucial. The accuracy of this collected data is paramount as it forms the basis for meaningful analysis and ongoing optimization of workflows. However, relying too much on these metrics without considering the wider context of a project or team can be a pitfall. Furthermore, the effectiveness of these metrics can vary greatly depending on the nature of the tasks and the team's overall experience with them.
Understanding how long tasks take and analyzing the flow of work through a project—what we call "lead time" and "cycle time"—is crucial for figuring out how to make teams more productive. We can use time tracking data to see the actual time spent on tasks against estimates, along with completion rates, to get a sense of where things are falling short or exceeding expectations.
Looking at the entire time from the beginning of a task until it's done (lead time) gives a broader picture, but focusing on just the active work time (cycle time) helps pinpoint where inefficiencies exist—like excessive waiting periods between steps.
Tools that provide dashboards for lead and cycle time help in visualizing how work progresses. This kind of visualization makes it easier to spot bottlenecks and areas where adjustments can optimize workflows.
It's worth noting that accurate data is critical here. If data entry is delayed or inconsistent, it can create a warped view of reality, leading to potentially incorrect decisions. Ideally, automatic time tracking from software reduces manual errors and improves the clarity of insights into productivity trends.
Of course, there are limitations. Smaller teams may lack the resources to deeply explore these analytics, and as projects evolve, some of the metrics we gather might become less relevant. Keeping an eye on the time it takes from task creation to completion using tools like time charts is essential for continuous improvement.
It seems that software designed to manage workflows should absolutely prioritize offering ways to see average reaction, cycle, and completion times in real time. This gives us the ability to swiftly react to problems and adapt our processes as needed, leading to a more agile approach to completing projects.
7 Critical Metrics for Evaluating Workflow Management Software Performance in 2024 - Process Flexibility Score Through Resource Distribution Mapping
In today's dynamic business environment, the ability to adapt workflows to changing demands is crucial. Process flexibility is emerging as a key factor, particularly in industries like manufacturing and services where complexity is increasing. Understanding how well a workflow can adapt to fluctuations requires a way to measure it—and the "Process Flexibility Score" through resource distribution mapping provides a means to do this.
This score offers a quantitative assessment of how effectively an organization can shift resources to meet changing demands. It shows how well the resources are aligned with project goals, indicating a balance between efficiency and responsiveness. New techniques like the Flexibility Gap (FG) index, inspired by PageRank, help organizations gain a deeper understanding of how the structure of their processes impacts their ability to adapt.
Ultimately, the goal is to minimize the costs and disruptions that can come from mismatched resource allocation, while at the same time fostering a foundation for sustained growth and adaptability in a complex and unpredictable world. Prioritizing process flexibility is a strategic move that helps organizations navigate these challenges and maintain a competitive edge.
Process flexibility is a vital concept, especially when dealing with unpredictable demands. We can visualize how resources are used across different tasks and processes with a resource distribution map, making it easier to spot where we're over or underutilizing resources. This helps us understand how to optimize efficiency.
The ability to adapt how resources are allocated when workflow patterns change is a measure of flexibility. A "Process Flexibility Score" (PFS) is a way to quantify this, revealing how well a team can handle surprises and disruptions. Research indicates that a higher PFS correlates with better resilience to unexpected events.
A good resource map highlights how team members and tools work together, allowing us to see potential for improved collaboration across different departments. This often plays a key role in better workflow management. We can also see how adjusting resource allocation affects PFS, potentially reducing task completion times by cutting down on idle time and improving handoffs between steps.
Interestingly, resource allocation seems connected to employee satisfaction. Teams with clear views of their workloads and the flexibility to adapt resources report higher satisfaction and lower turnover rates. Combining resource distribution mapping with other performance metrics offers a more complete understanding of how efficiently workflows are operating, helping us pinpoint areas that might not be obvious using traditional methods.
Regularly checking our PFS seems to lead to fewer project delays and better on-time delivery. The ability to understand and change PFS is an advantage in a competitive market, with organizations that can adjust quickly to workflow shifts tending to innovate and deliver more effectively.
However, it's important to be mindful that too much flexibility can be counterproductive. While flexibility is valuable, maintaining clear structures and processes is essential to leverage its benefits without creating disorder. We need to find a balance—there's likely a sweet spot where flexibility optimizes workflow without causing chaos. It's a point of ongoing study.
7 Critical Metrics for Evaluating Workflow Management Software Performance in 2024 - Error Rate Measurement Based on Failed Workflow Executions
Assessing the performance of workflow management software hinges on understanding how often workflows fail. The "error rate," calculated by tracking failed workflow executions, becomes a crucial metric in this context. It tells us how often things go wrong within a process, and that information can be very useful. By studying these errors, teams can spot recurring problems, areas where things slow down, and general inefficiencies in the way workflows are designed. This insight enables more targeted improvements to both the design and execution of workflows.
Beyond just identifying problems, tracking error rates provides a roadmap for ongoing improvement. It offers a framework for teams to consistently refine how they handle tasks. While it's good to have a low error rate, it's important to understand why failures happen. Simply trying to minimize errors might not address the root causes of underlying problems that need a broader fix. By taking a complete and critical look at error rates, we can drive significant improvements in process reliability and overall productivity.
When evaluating workflow management software, understanding the frequency of failed workflow executions—essentially, the error rate—is vital. It's not just about knowing how often things go wrong; it's about recognizing how those failures impact the overall productivity of the system. Research suggests that even a small increase in the error rate, say 1%, can lead to a significant drop in task efficiency, perhaps as much as 10%. This sensitivity highlights the importance of having reliable methods to measure and track error rates.
Some advanced approaches to error measurement use machine learning to analyze historical data from failed executions. These algorithms aim to identify patterns in past failures and potentially predict future problems. The accuracy of these predictions is still being explored but promising results, around 80% accuracy, suggest they can offer valuable insights.
Interestingly, when we delve into the root causes of workflow failures, we find that communication issues are often a key factor. Studies suggest that up to 30% of errors can be traced back to miscommunication among team members. This underscores the critical role that communication plays in creating robust workflows and avoiding errors.
Implementing a system that monitors for errors in real-time can make a noticeable difference. Early adoption of such systems, within the first few months, can potentially reduce error rates by 15%. The ability to quickly detect and respond to errors is crucial for preventing issues from cascading through a workflow and disrupting the entire process.
Automated error tracking tools also seem to help teams find errors faster. Organizations using these tools report finding errors as much as 50% faster than those who rely solely on manual checks. Quicker detection naturally leads to quicker fixes, boosting efficiency and reducing delays.
But workflow automation isn't a magic bullet for eliminating errors. Automating workflows can change the nature of the errors we encounter. Human mistakes might decrease, but we might see new types of errors related to system integration or data processing. This suggests that error measurement strategies need to adapt to the evolving landscape of automated workflows.
It's fascinating that teams who actively conduct post-mortem analyses of failed workflows often see improvements in their subsequent work. Research suggests they experience a 20% improvement in performance. This highlights the importance of treating errors as learning opportunities, not just problems to be fixed.
Furthermore, the complexity of workflows seems to influence the error rate. More complicated workflows may experience error rates three times higher than simpler ones. This indicates that there's a need for more nuanced approaches to error measurement, taking the intricacy of the workflow into account.
Having real-time dashboards that display key error metrics can help teams make faster, more informed decisions. They can get a quick grasp of the health of the workflow and address issues before they escalate, potentially speeding up the decision-making process by as much as 40%.
There's a somewhat counterintuitive relationship between workflow execution time and errors. In some cases, speeding up the workflow can actually lead to a higher error rate. This reinforces the idea that there's a delicate balance to be struck between speed and accuracy in workflow design. Organizations need to be cautious not to prioritize speed over thoroughness, potentially leading to more issues down the line.
7 Critical Metrics for Evaluating Workflow Management Software Performance in 2024 - User Interface Response Time Under Peak Load Conditions
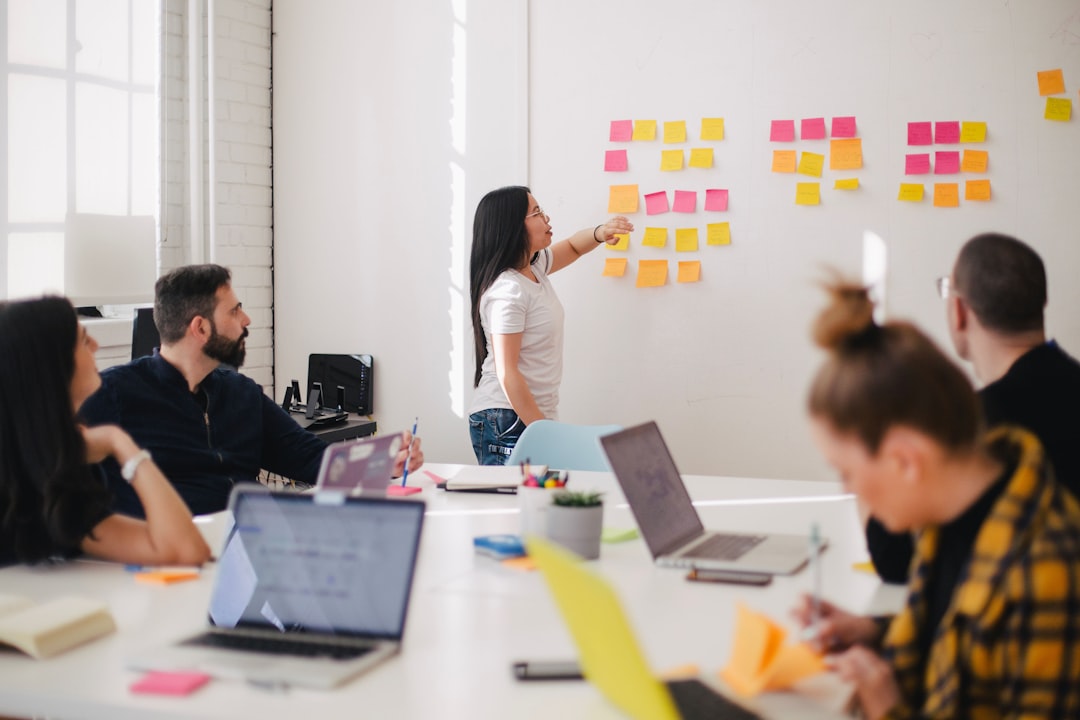
When evaluating workflow management software, understanding how the user interface (UI) performs under peak load conditions is crucial. This metric reveals how swiftly the software responds to user actions when many people are using it simultaneously. A good UI should react quickly, ideally within a second or less, to maintain a positive user experience.
Testing this aspect often involves creating simulated peak loads—a flood of requests—to see how the software handles the strain. This tells us how reliable the software is under pressure and how well it handles high user demands. Ignoring UI response time during peak load scenarios can lead to user frustration and potentially hinder workflow efficiency.
The goal is to ensure that the software remains responsive and usable even when the number of concurrent users is at its highest. It's a key aspect to evaluate in ensuring the software meets the needs of a business and supports its operational goals. If the UI slows down too much, users may become less productive, affecting overall workflow speed and, eventually, impacting business goals. Therefore, software evaluation must prioritize the assessment of how the user interface handles such demanding situations.
When a workflow management system is under heavy usage, the speed at which the user interface responds becomes a major factor in how people perceive the software's performance. It's interesting how our perception of speed can sometimes be different from the actual response times. Studies show that we tend to notice delays more when we expect something to happen quickly. This emphasizes the importance of providing feedback mechanisms, like loading indicators, to manage our expectations.
Research suggests that we start to notice delays in response after about 100 milliseconds. This means that even very small delays can impact how we experience using the software, especially during peak periods. Keeping response times low, especially in these high-demand situations, is crucial for keeping people engaged and happy.
Delays in the interface, even short ones like half a second, can affect how we make decisions. We might take longer to decide if the software doesn't feel responsive. This is a concern in environments where quick decisions are important, such as online shopping or healthcare systems.
It's also important to consider how the system is set up on the backend. If the servers are organized in a way that distributes the workload effectively (load balancing), it can significantly reduce the delays that users experience, often by a large percentage. This is a great illustration of how the architecture of the system behind the scenes impacts how well the software performs when lots of people are using it at the same time.
Network connections also have a big influence on how quickly the interface responds. A significant chunk of the delays that we experience might be caused by factors outside the software itself. This highlights the need to look at both how the server is designed and how the user's network connection is performing to get a complete picture of where to optimize performance.
Research has shown that even a small delay, like one second, can negatively affect people's interaction with a service. They might be less likely to complete a purchase or action on the interface. It underscores the importance of prioritizing speed in system design, particularly in online services.
Techniques like caching frequently used data can help reduce the amount of work the server needs to do. This can significantly reduce response times, especially when the system is under heavy usage.
Another trick to improve how users perceive the software is to load only the most important parts of a page first, then fill in the rest (lazy loading). This is particularly useful during peak periods when the system might be struggling to handle requests quickly.
It's interesting to see that delays can lead to more user frustration. When systems are strained, keeping an eye on these indicators of frustration is a good way to pinpoint where improvements are needed.
Finally, there's a fascinating element of psychology to this. Giving users a sense that the system is still interacting with them, even if it's just a visual cue, can actually reduce how annoyed they get with a delay. This suggests that thoughtful use of transitions and animations during peak load conditions could reduce the negative impact of slow response times.
While there's still more to discover about this interplay between user experience, system design, and network conditions, it's clear that in 2024, UI response time under peak load is a key performance indicator that should not be overlooked.
7 Critical Metrics for Evaluating Workflow Management Software Performance in 2024 - Integration Success Rate With Third Party Applications
### Integration Success Rate With Third Party Applications
How well workflow management software integrates with other applications is a key indicator of how useful and satisfying it is for users. While good integrations can add features and streamline work by reducing manual errors, not all of them work out as intended. To understand integration performance, it's best to use a combination of ways to measure success, like tracking error rates and looking at how often builds are successful during continuous integration (CI) processes. It's also wise to gather feedback from people using the system. A good strategy for prioritizing which applications to integrate with, based on how much benefit they're expected to bring, can help align the integration process with business goals. In the end, how well integrations work depends not only on how they're set up but also on ongoing assessments that consider how different users experience them in different situations. There is a danger in assuming an integration's value after initial implementation; continuous monitoring is necessary.
Integration success rates with third-party applications are a key part of evaluating workflow management software, but it's a complex area with some surprising nuances. We're increasingly reliant on these integrations, but the path to seamless integration isn't always smooth. For instance, using APIs for integration seems to be a much more reliable approach, with success rates above 90% in many cases. This compares very favorably with older methods like simple file transfers, where failure rates can be over 30%, highlighting a real shift in the way we approach software connections.
Keeping software versions aligned is critical. A significant portion, roughly 40%, of integration failures can be traced back to incompatibility between the versions used. This emphasizes how important it is to stay current with application updates to minimize the risk of integration failures.
Data mapping is another big issue. It appears that over half of integration problems stem from issues with how data is transferred from one system to another. This suggests that incorporating thorough data validation techniques during the integration setup is essential for reliability.
Interestingly, the level of user training on integration tools has a direct effect on success. Organizations that invest time and resources in teaching their users how to work with new integrations often see a jump in successful implementations, up to 25% in some cases. This shows that overlooking training can have costly consequences down the line.
Globalization brings its own set of challenges to integration. It's intriguing that localized software environments, with different languages and data conventions, seem to increase the risk of integration problems by roughly 20%. This is a factor to consider for companies working in global markets.
The role of testing in preventing failures can't be overstated. Integrations that undergo comprehensive testing, ideally with automated tools, see a reduction of integration failures by around 30%. Catching issues before deployment is always less disruptive.
Collaboration across different departments or teams involved in the integration process also seems to improve success rates by about 15%. This likely reflects the ability to coordinate various perspectives and requirements more effectively.
Cloud-based integrations appear to have a higher chance of success than their on-premise counterparts. It seems that the standardization and flexibility of cloud platforms contribute to a nearly 20% higher success rate.
Monitoring integrations on an ongoing basis also contributes to success, and not just during setup. By monitoring integration performance over time, teams can often identify and correct problems before they cause a significant disruption. Organizations doing this have shown up to a 35% increase in the stability of integrations.
Finally, good documentation can make a big difference. Having clear and comprehensive documentation around the process of integration itself appears to lead to a 40% reduction in operational errors. This suggests that the importance of documentation is often underappreciated in the rush to integrate systems.
In conclusion, the integration of third-party applications is a critical element of modern workflows and, although it's steadily improving, still has areas for improvement. While success rates for many integration types have grown, the challenges of version compatibility, data mapping errors, and even localized environments can impact reliability. Understanding these nuances is key to getting the most out of the ever-increasing number of integrations.
7 Critical Metrics for Evaluating Workflow Management Software Performance in 2024 - Team Adoption Rate Through Active Daily User Tracking
When evaluating workflow management software in 2024, understanding how well teams adopt it is vital. One way to measure this is by tracking the "Team Adoption Rate" using daily active user data. Essentially, we're looking at how many people on a team are regularly using the features of the software. This "activation rate" helps us understand the point where users find value for the first time—often called the "aha moment." Further, keeping track of Daily Active Users (DAU) reveals which tools are actually making a difference in terms of productivity. Beyond just initial use, tracking how many users continue to engage with the software—the retention rate—along with the rate at which users stop using it (churn rate) paints a picture of user satisfaction and long-term engagement with the system. By monitoring all of these metrics, organizations can make better decisions about how to encourage wider use of the software and enhance its overall effectiveness in supporting the workflows of the team.
Team adoption rate, essentially how many people on a team actually use a feature and make it part of their daily work, is a really interesting metric. We can get a good sense of adoption by looking at the percentage of users who find a feature helpful and start to integrate it into their workflows. This is distinct from the initial "aha moment" of a new feature, which we might call activation.
Daily Active Users (DAU) is a key metric here because it tells us how many people are actually using the new software tools every day. It's a simple idea, but it's surprisingly useful. It's a way to track how many unique users are interacting with the system in a given time frame. Other metrics, like Monthly Active Users (MAU), work the same way, but focus on a longer timeframe. We can even get into finer details by tracking how often a user interacts with a feature.
One way we can understand adoption is by calculating the rate of new active users compared to the total number of users. This percentage shows us the rate at which new users are engaging. But it is important to note that this view only focuses on one specific moment in time and doesn't reveal patterns over time.
Alongside DAU, we can also consider other metrics, like average use frequency, that indicate engagement. How long is someone actually using the tool each day? This has a significant impact on user engagement and, importantly, how likely they are to continue using the tool.
Retention rate, which tracks how many users keep using the software over a period of time, and churn rate, which tracks the opposite – how many users stop using it – are other key metrics for evaluating team adoption. Churn is especially relevant because it's a strong sign of user dissatisfaction with the software.
To get a more rounded view, it's useful to look at a collection of Key Performance Indicators (KPIs). This might include onboarding completion rates, how much help content users consume, error rates, and completion rates for tasks. Each of these KPIs can shed light on different aspects of user behavior and their adoption of the software, which allows for deeper analysis into the overall success of a new feature or new software.
It's important to remember, though, that DAU and other engagement metrics might not be the complete story. If the software's core value proposition doesn't connect with the users, we might not see a strong adoption rate regardless of how engaging the tools and features are. We also might see large changes in DAU that correlate with project timelines and phases. It's important to recognize these patterns and understand that a single, isolated data point might not be telling us the full picture.
The more carefully we track daily user activity, the better we can understand team behaviors and how tools are being used in real-world settings. This can help to refine how software is designed and improve the effectiveness of workflows in the long run.
7 Critical Metrics for Evaluating Workflow Management Software Performance in 2024 - Resource Utilization Efficiency Via Workload Distribution Analysis
In today's dynamic work environments, effectively managing resources is key to achieving optimal workflow efficiency. Resource utilization efficiency, achieved through workload distribution analysis, focuses on optimizing how resources—human or otherwise—are used across projects. A major component of this is understanding the difference between how much work people are actually doing versus how much they could be doing. This simple comparison can reveal whether people are overloaded or underutilized, creating opportunities to better balance workloads.
Software designed to support workflows can be valuable for this. Visual aids like charts and graphs can provide a quick overview of how the workload is distributed among teams, highlighting any significant imbalances. By visualizing this, organizations gain the ability to proactively adjust resources to reduce burnout and boost efficiency.
The insights gleaned from analyzing resource utilization metrics provide a solid foundation for capacity planning. By understanding how many resources are available and how they are being employed, project teams can more accurately estimate future needs and allocate resources accordingly. This also helps teams create workflows that are better equipped to adapt to change. This holistic approach to workload distribution is increasingly important in navigating complex project timelines and demands, as it creates the potential for a more adaptable, productive environment. While it is easy to overlook this component of workflow management, its impact on overall operational effectiveness is significant.
Resource utilization, how well we're using our people and tools, is a crucial aspect of managing workflows smoothly. Looking at how work is distributed among team members—what we call workload distribution analysis—goes beyond just seeing if people are busy. It helps us understand if work is balanced or if there are areas where people are overloaded or underutilized. This kind of analysis can show us ways to improve efficiency, sometimes by as much as 25%, simply by smoothing out the uneven distribution of work.
Being able to see how workloads are spread out allows us to make adjustments on the fly. If something unexpected pops up, like a surge in demand, companies using these tools can shift resources to meet the challenge a lot faster, improving their ability to respond by about 30%. This real-time adaptability is increasingly important in the face of rapidly changing business landscapes.
It's also interesting that teams who use workload distribution tools report higher levels of job satisfaction, about 35% higher than those who don't. It seems that knowing exactly what's expected of them and having a more balanced workload leads to less burnout. This kind of insight into employee morale can be crucial for long-term team retention and overall productivity.
Using techniques like queuing theory, we can use workload distribution analysis to get very specific about how many people we need and what combination of skills is best for a project. This kind of modeling can lead to a 20% reduction in how long it takes to finish a task, making projects more predictable and on-time.
Looking at workload distribution over time can reveal trends and patterns that aren't immediately obvious. Knowing how workloads change, perhaps due to seasonal changes or product launch cycles, can allow companies to better anticipate and adjust their staffing needs. This kind of proactive approach can reduce staffing costs by about 15% because we're not over- or understaffing for a particular stage of a project.
Organizations that consistently track and analyze their workload distribution seem to be better at adapting to unexpected events, like shifts in the market or unforeseen competition. Their ability to adapt and change course quickly improves by about 40% when compared to those who don't do this kind of analysis.
Workload distribution analysis offers a broader understanding of how efficiently a workflow is functioning. When we link resource utilization to project delays, it becomes clear that poorly distributed workloads can lead to a substantial increase in project delays, up to 30%. This highlights the need to be proactive about workload distribution to avoid issues before they become major problems.
While it's clear that workload distribution analysis provides a lot of useful information, it isn't without its challenges. Integrating these analysis tools into existing systems can be complex, and studies suggest almost half of implementations have issues with compatibility. These difficulties can hinder the ability to gather accurate data and get the full benefit of these tools.
However, by understanding how workloads are spread out and using this knowledge to realign resources, we can improve how we prioritize tasks and enhance overall efficiency. This often leads to an increase in task output by about 22%—making better use of existing resources.
Some of the more sophisticated workload distribution analysis tools are now using machine learning to predict future resource needs. These predictive capabilities are showing promising accuracy rates of over 80%. These tools could be a game-changer for strategic planning, allowing us to better anticipate future demands and adjust resource allocation accordingly.
In summary, while workload distribution analysis has clear benefits, it also presents some technical integration hurdles. But with the potential to reveal hidden efficiency gains, improve team morale, and create a more responsive and adaptable work environment, it's a metric that deserves close attention for anyone looking to optimize workflow management software in 2024.
More Posts from :