Understanding Digital Media A Deep Dive into Machine-Readable Data Formats in 2024
Understanding Digital Media A Deep Dive into Machine-Readable Data Formats in 2024 - Binary Data Evolution From Storage Tapes to Quantum Computing 2024
The journey of binary data storage has been a remarkable one, moving from the early days of magnetic tapes to the cutting-edge frontier of quantum computing. Quantum computing leverages the strange properties of qubits, allowing for operations unlike those possible with traditional bits. Superposition and entanglement empower more intricate calculations and data manipulation. In 2024, we're poised to see quantum computing play a key role in long-term data preservation and in meeting the demands for storing increasingly massive amounts of data. This shift may bring about significant changes in how we manage and handle data across various domains. The prospect of cloud-based quantum computing services promises to make this powerful technology more accessible to researchers and businesses alike, potentially accelerating advancements in areas like artificial intelligence. Though the potential of quantum computing is exciting, there are still significant hurdles to overcome in realizing its full capabilities and ensuring it is deployed responsibly.
The journey of binary data storage, from its early days on tapes to the promising horizon of quantum computing, reveals a fascinating evolution. While magnetic tape technology, often viewed as a relic, continues to find a niche in long-term archival due to its durability and affordability, its limitations in access speed are evident. The shift to SSDs exemplified a paradigm change, introducing random access capabilities that have profoundly reshaped software development.
Quantum computing, still grappling with its foundational stages, offers a tantalizing potential to upend data processing. The ability of qubits to occupy multiple states simultaneously suggests a potential for computational leaps beyond anything classical computers can achieve. However, the persistent challenge of error rates remains a significant obstacle to widespread adoption. Efforts in error correction are showing progress, inching towards practical quantum applications.
The emergence of quantum computing has also spurred related fields. Quantum key distribution, for example, leverages quantum principles to establish incredibly secure communication networks. Meanwhile, researchers are exploring novel storage solutions like DNA-based systems, suggesting that the future of data storage might lie in fundamentally different approaches from traditional electronic mediums.
Quantum computing's potential impact on industries like AI is being actively researched. Researchers believe that quantum algorithms can accelerate machine learning by efficiently processing massive datasets, opening the door to advancements in areas like drug discovery or materials science. Furthermore, the field of quantum many-body simulations attempts to confront the formidable challenges inherent in quantum computing by investigating the dynamics of complex quantum systems.
The broader landscape of quantum computing is marked by optimism and caution. Several companies are now preparing to offer quantum computing as a cloud service in 2024, making the technology more accessible. Yet, discussions among experts acknowledge the remaining hurdles in developing fully functional and stable quantum computers. While these developments are encouraging, many practical applications remain on the horizon. The path to a quantum-powered future for data storage and processing, though uncertain, is being actively explored by researchers and engineers worldwide.
Understanding Digital Media A Deep Dive into Machine-Readable Data Formats in 2024 - Machine Learning Data Formats Transforming PDF Neural Networks
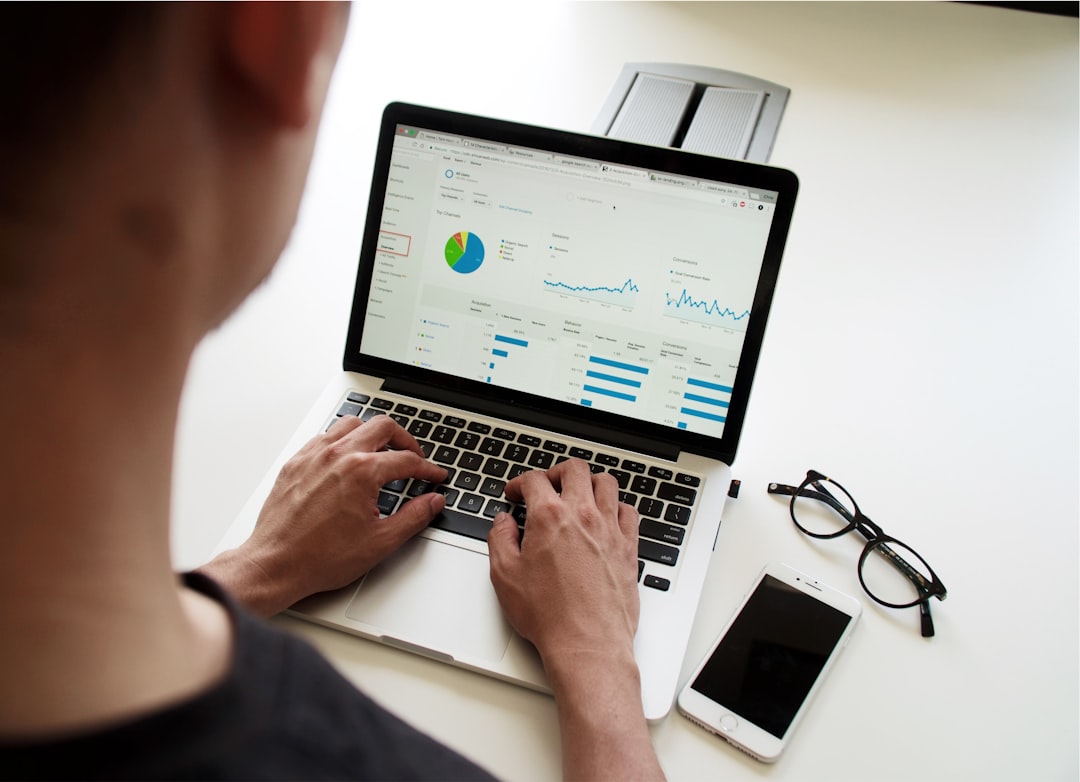
Within the evolving field of machine learning, the way PDF documents are handled by neural networks is undergoing a transformation driven by new data formats. The need for more efficient computation and storage in advanced deep learning applications has led to the development of specialized formats, like Microscaling MX. These formats aim to streamline the use of data, reducing the burden on computational resources and storage space.
Converting PDFs into these machine-readable formats requires specific data transformations, a process that essentially cleans and optimizes datasets to prepare them for analysis by algorithms. This cleaning process is vital for addressing issues like noisy data, missing information, and other inconsistencies that can impede a neural network's performance. By resolving these issues, the transformed data becomes better suited for the intricate calculations neural networks employ.
As the field progresses, the integration of these innovative data formats promises to unlock new avenues for gaining valuable insights from digital media, including the wealth of information stored in PDFs. It remains to be seen how far these developments will take us in terms of understanding and interpreting the content within PDFs, but the potential for improved analysis is clearly there. However, it's worth considering that simply reformatting data doesn't guarantee better results. The actual design and training of these neural networks remains paramount to achieving accurate and useful results.
### Machine Learning Data Formats Transforming PDF Neural Networks
1. **PDFs: A Complex Landscape for Neural Networks**: PDFs, with their blend of text, images, and vector graphics, present a unique challenge for neural networks. These networks usually thrive on clean, structured data, making PDF's multifaceted nature a hurdle in training and using them effectively.
2. **Extracting Meaning Through Embeddings**: Some more advanced neural network approaches utilize OCR to convert text within PDFs into a format that machines can readily understand. This allows for extraction of textual embeddings, which can be fed into machine learning models. However, this typically requires some initial processing to account for various font styles and layouts.
3. **Transfer Learning Adaptability**: Transfer learning is gaining traction in PDF processing, enabling models initially trained on general documents to adapt to specific types like legal or medical PDFs while preserving performance. This adaptability is crucial as PDF formats vary greatly across disciplines and require handling diverse layouts and terminology.
4. **Structuring with Segmentation**: Techniques like CNNs are increasingly used to segment PDF content into meaningful parts such as headers, tables, and paragraphs. This allows neural networks to process documents in a hierarchical way, leading to more accurate information retrieval.
5. **Sparsity and Attention Mechanisms**: The challenge of sparse data in PDFs has driven innovation in feature extraction. Many networks now utilize attention mechanisms to focus on relevant parts of a document, mitigating inefficiencies from irrelevant data.
6. **Layer Normalization for Stability**: As we see neural networks increasingly applied to PDF data, layer normalization has emerged as a useful technique to improve deep learning model training. By lessening internal variations in data, this method enhances network stability and training speed when handling complex document structures.
7. **The Difficulty of Benchmarking**: Establishing standard benchmarks for neural networks working with PDFs is tough due to their varied nature. The discrepancies in layout, font styles, and embedded components make it hard to create consistent datasets for comparing model performance.
8. **Expanding Datasets Through Data Augmentation**: When dealing with the intricacies of PDF data, researchers often use data augmentation techniques like rotation, resizing, and contrast adjustments. These methods increase the training dataset and help prevent the model from overfitting to specific document types.
9. **Neural Network Scalability**: The size of neural networks used for PDF processing varies considerably. There are small, efficient models for low-latency tasks and larger, more complex architectures for in-depth analysis. The chosen network size directly impacts the computational cost and inference speed in real-world use cases.
10. **The Trend Toward End-to-End Systems**: We are seeing a movement toward end-to-end machine learning systems that process PDFs within a unified framework. This points to the significance of integrating different data processing stages—from initial processing and extraction to classification and analysis—into a cohesive pipeline for increased efficiency.
Understanding Digital Media A Deep Dive into Machine-Readable Data Formats in 2024 - Audio Codecs War FLAC Takes Lead Over MP3 in Digital Music Space
The landscape of digital audio is experiencing a shift in codec dominance, with FLAC (Free Lossless Audio Codec) gaining prominence over the previously ubiquitous MP3 format. FLAC's strength lies in its ability to compress audio data without any loss of sound quality, a feature that has made it favored by audio enthusiasts. This contrasts with MP3, a lossy compression format that achieves smaller file sizes by discarding some audio information, leading to a decline in fidelity. The growing preference for FLAC suggests a trend among consumers towards higher-quality audio experiences. This shift highlights a broader trend towards appreciating superior audio fidelity in digital media. Although MP3 remains a convenient and widely used format, the increasing availability and adoption of FLAC and other codecs that prioritize sound quality, such as AAC and Ogg Vorbis, are driving a transition towards a more refined auditory experience. It is a compelling illustration of how evolving expectations and advancements in technology are reshaping the way we listen to and appreciate music.
In the realm of digital audio, the choice of codec significantly impacts the quality and size of audio files. FLAC (Free Lossless Audio Codec) has emerged as a compelling alternative to the long-standing dominant MP3 (MPEG-1 Audio Layer III) format. While MP3 became ubiquitous due to its compact file sizes, it relies on lossy compression, which inevitably discards some of the original audio data. This can result in a noticeable decrease in audio fidelity, particularly for discerning listeners.
FLAC, on the other hand, employs a lossless compression method. This means that all of the original audio data is preserved during compression, leading to a more accurate representation of the original recording. This characteristic makes FLAC particularly attractive for applications where high-fidelity audio reproduction is crucial, such as professional audio editing or listening to music on high-quality audio systems.
The choice between FLAC and MP3 often boils down to a trade-off between file size and sound quality. While MP3 files are generally smaller, they inherently sacrifice some of the audio detail present in the original recording. FLAC files, due to their lossless nature, are typically larger, but they capture the full range of sounds and nuances present in the original audio.
Besides FLAC and MP3, other audio codecs exist. AAC (Advanced Audio Coding) is considered an improvement over MP3 in terms of sound quality at comparable bitrates. Ogg Vorbis, while less common, also achieves better quality than MP3 with similar file sizes. Additionally, newer formats like MQA (Master Quality Authenticated) are designed to streamline the delivery of high-resolution audio through lossless compression specifically for streaming.
The ongoing evolution of audio codecs reflects the shifting priorities of users and technology. The popularity of MP3 stemmed from its practicality, enabling users to store and share music more efficiently. However, with the rise of higher quality audio hardware and listeners becoming more aware of audio quality differences, the demand for codecs like FLAC is steadily increasing.
The advantages of FLAC are multifaceted. It maintains the full dynamic range of the audio signal, avoiding the dynamic range compression that MP3 compression often introduces. This leads to a more natural and nuanced listening experience. Further, FLAC is able to preserve a wider frequency response compared to MP3, resulting in a more complete representation of the audio spectrum.
Yet, FLAC isn't without its drawbacks. It results in larger file sizes, which can be a consideration for storage space or bandwidth limitations. Decoders, while faster on modern hardware, may present limitations on older devices with slower processors.
Considering the wider context of audio production and listening, FLAC offers clear benefits for audio fidelity. The growing support and compatibility for FLAC within digital music ecosystems suggest that it may eventually supplant MP3 in specific niches, such as high-fidelity audio playback and professional audio workflows. This trend reflects a growing emphasis on the quality and preservation of audio information in the digital realm, as users increasingly appreciate the nuances of high-quality audio.
The battle between audio codecs continues to evolve with FLAC's rise in prominence challenging MP3's historical dominance. While MP3's legacy is firmly cemented, the future of digital audio likely involves a broader acceptance of lossless codecs, like FLAC, catering to the evolving demands for higher quality audio reproduction and appreciation for audio engineering nuance. The path forward may involve a blend of formats, with codecs like FLAC emerging in applications where fidelity is paramount and MP3 remaining a suitable choice for space-constrained environments or streaming services looking to optimize bandwidth. The continuous refinement and development of audio codecs are an integral part of the journey towards delivering richer, more nuanced experiences for those seeking high-quality audio in the digital space.
Understanding Digital Media A Deep Dive into Machine-Readable Data Formats in 2024 - Video Format Revolution WebM Challenges H265 Dominance
The video format landscape in 2024 is showing a dynamic shift, with WebM challenging the dominance of H.265. H.265, known for its high compression and quality, has been a popular choice, but WebM, being open-source, is becoming increasingly attractive for internet-based video. The cost associated with using proprietary formats like H.265, due to licensing fees, has become a worry for some, potentially pushing creators and platforms towards more open and affordable alternatives. WebM's ability to deliver good quality video at lower data rates is a key advantage, especially for online content producers trying to balance video quality with efficiency. This potential change reflects both technological advancements and evolving user preferences, as the video streaming industry continues to evolve and find balance with the needs of content distribution. The future of video format will likely continue to adapt to changing bandwidth capacity and expectations for quality across devices.
The world of video formats is in a state of flux, with the open-source WebM format presenting a compelling challenge to the dominance of H.265. Initially introduced in 2010, WebM, spearheaded by Google, utilizes the VP8 and VP9 codecs, emphasizing web compatibility and avoiding copyright restrictions. This contrasts with H.265 (HEVC), a more recent codec known for its remarkable efficiency. H.265 significantly reduces bitrate requirements, achieving up to 50% less than H.264, making it ideal for high-resolution content. However, the licensing landscape surrounding H.265 introduces complexity, potentially limiting its adoption.
WebM, by virtue of its free and open nature, offers a more accessible path for developers to implement video solutions. This open-source approach minimizes the licensing hurdles that can stall broader adoption. Notably, WebM has displayed improved streaming performance, especially in low-bandwidth environments. This is particularly useful for applications sensitive to latency, like video calls. On the other hand, H.265's decoding capabilities currently lean towards newer devices, creating a compatibility gap with older hardware and browsers.
The quality advantages of H.265 become pronounced when dealing with high-resolution formats. However, WebM appears to hold its own, particularly when facing constrained conditions like lower bandwidth. This makes it a more reliable choice for mobile devices and environments with unstable internet connections. The consequence of this is that real-world video streaming often provides a smoother user experience with WebM.
While H.265 delivers on visual quality, the conversion process places greater demands on processing resources and time. This can present a burden on services that regularly transcode videos. In comparison, WebM has a simpler encoding process. The licensing intricacies that accompany H.265, encompassing a maze of patents and fees, can be a deterrent. WebM's openness provides a clearer path to development and fosters a wider ecosystem.
Looking ahead, we may witness a future where WebM incorporates advancements from both H.265 and AV1, a promising new codec. This could yield a more widely compatible format that strikes a better balance between efficiency, quality, and legal clarity, meeting the needs of a wide range of video applications in the years to come. The potential convergence of strengths from these various formats underscores the ongoing evolution in this space. It remains to be seen how the interplay of quality, efficiency, and accessibility will influence the future adoption of video standards.
Understanding Digital Media A Deep Dive into Machine-Readable Data Formats in 2024 - XML vs JSON The Battle for Web Data Standardization
The ongoing discussion surrounding XML and JSON underscores a fundamental difference in how data is structured and shared on the web. JSON's rise to prominence stems from its concise design, ease of use, and natural compatibility with JavaScript. This makes it a popular choice for modern web applications that prioritize speed and efficiency. XML, on the other hand, with its more detailed syntax and support for rigorous validation through schemas, is better suited for situations where data integrity and complex, hierarchical structures are crucial. While both have their strengths, the choice between the two often comes down to the specific needs of the application. It’s not a simple “one-size-fits-all” solution, and developers need to carefully weigh the pros and cons to make the best decision for the task at hand. As the web evolves and becomes increasingly dynamic, the need to effectively manage and share data will only grow. The choice between XML and JSON will continue to be a key factor in how this is done, impacting how web developers handle data across a variety of systems and platforms.
XML and JSON are both established formats for exchanging data, particularly within the digital landscape. XML, developed by the World Wide Web Consortium in the late 1990s, is both human and machine-readable, offering versatility for various tasks. Its tag-based syntax makes the data structure explicit, which can be beneficial for complex scenarios, although it can result in larger file sizes compared to JSON.
JSON, or JavaScript Object Notation, has emerged as a popular lightweight alternative, especially within web applications. Its inherent compatibility with JavaScript simplifies parsing into objects within the language. This simplicity contributes to reduced file sizes and faster load times, particularly important with increasingly large datasets found on the internet. The decision to use JSON over XML often boils down to considerations about how data is handled and transmitted.
While both formats have their strengths, certain inherent traits might favor one over the other in specific situations. JSON, for example, natively supports data types like numbers, strings, arrays, and booleans. XML, on the other hand, treats most data as text, leading to more complexity during processing. When data integrity is critical, XML's schema definitions can enforce validation, which offers a more robust way to manage the structure of data. JSON Schema exists, but it's not as universally applied, leading to potential ambiguity in data interpretation across different systems.
XML's hierarchical nature allows it to represent intricate data relationships through tags and nested structures. This complexity can be both a boon and a drawback. It provides more descriptive information about the structure, but it can also slow down processing compared to JSON's potentially simpler structures. While JSON can also nest data, it often presents a flatter representation, which might not be as useful for representing very complex data sets.
The issue of error handling highlights another divergence. XML, with its schemas and defined structure, usually produces more detailed error messages when things go wrong. JSON is easier to troubleshoot for smaller errors, but catching issues in larger and more complex datasets can be more difficult due to the lack of enforced data structure.
Furthermore, JSON tends to be the favored format for data exchange through RESTful APIs in modern web development. This is due to its efficiency in both file size and structure, making it better aligned with the needs of many web-based systems. JSON's straightforward syntax leads to better performance during parsing, especially in JavaScript environments where JSON parsing is handled natively. XML's more complex syntax can add overhead, slowing down data transfer and limiting its suitability for real-time applications.
In the larger context, both XML and JSON play important roles in data interchange. XML finds relevance in more established applications and legacy systems that require strict data validation, while JSON is better suited for today's web-centric applications and dynamic environments where efficiency is prioritized. Ultimately, choosing between these formats hinges on a careful assessment of the specific use case and the unique characteristics of the data being handled. The decision is not simply about choosing one over the other, but understanding the nuances that lead to better outcomes when working with machine-readable data.
Understanding Digital Media A Deep Dive into Machine-Readable Data Formats in 2024 - Database Formats Graph Systems Outperform Traditional SQL in 2024
Within the evolving landscape of data management in 2024, graph database systems are showing a clear advantage over traditional SQL databases, particularly when dealing with intricate and interconnected datasets. Graph databases represent information using nodes, edges, and properties, naturally mirroring the relationships between pieces of data. This structure allows for more fluid schema adjustments and simplifies real-time data processing. The rise of generative AI and other demanding applications within businesses highlights the value of graph database flexibility and speed.
While SQL databases continue to hold a prominent position due to their widespread use among developers, they face increasing challenges when confronted with the complexities of modern data. SQL's rigid table structure, based on rows and columns, can become a barrier when data relationships are highly intertwined.
As the demand for managing ever-more complex data continues to rise, graph database systems are becoming increasingly attractive as a modern alternative. They offer a more adaptable and efficient approach for storing and accessing the type of machine-readable data critical in diverse domains like digital media. This shift underscores the changing nature of data management, where the ability to represent intricate relationships effectively becomes paramount.
Within the evolving landscape of data management in 2024, graph database systems are gaining significant traction, particularly in scenarios where data relationships are complex and interconnected. These systems, which represent data as nodes and edges, offer a distinct advantage over traditional SQL databases, particularly for handling intricate relationships.
One of the primary advantages of graph databases is their ability to adapt to changing data structures with relative ease. Unlike the rigid schemas of SQL, which necessitate significant restructuring when data requirements shift, graph systems support flexible, schema-less structures. This flexibility can drastically reduce the time required to adapt to changes in application needs and enables a more agile development process.
When it comes to querying complex relationships, graph databases have shown a dramatic improvement over their SQL counterparts. For example, tasks such as social network analysis or recommendation engine development, where the interplay of relationships is central, benefit greatly from the graph-based approach. Queries that would be computationally demanding and potentially slow in SQL often execute far more quickly in graph systems, often demonstrating an order of magnitude improvement in performance.
The natural fit for modeling interconnected data makes graph systems an attractive choice for engineers. Instead of working with tables and joining related data, which can be a source of performance issues and complexity in SQL, graph databases offer a more intuitive method to visualize and query how different elements relate to one another. This often leads to a clearer and more effective understanding of the data's inherent connections.
Moreover, graph databases can scale remarkably well within distributed environments. This capability is crucial as datasets become larger and more distributed. Implementing distributed graph systems often allows for horizontal scaling without the complexities often associated with scaling traditional SQL systems. This characteristic has led to their growing popularity in big data applications where scalability is paramount.
In many applications, gaining insights into relationships in real time is critical. Graph systems, through algorithms like PageRank, can quickly compute relationships and connections within a dataset. This speed is valuable in situations such as fraud detection, where swiftly identifying connections can be essential.
Beyond their performance advantages, graph databases are also capable of managing a diverse range of data types, including both structured and unstructured data. This capability allows for richer and more comprehensive datasets compared to SQL databases, which typically require predefined data types.
Furthermore, the query languages designed for graph databases, such as Cypher or Gremlin, enable engineers to express queries in a more nuanced and flexible way. These languages offer advantages over traditional SQL joins, especially in intricate data environments.
One significant bottleneck in SQL-based systems is the need for extensive join operations. Graph databases, through their structure, inherently minimize the need for these operations, translating into faster query execution times.
From a cost perspective, graph databases, especially open-source implementations, can be considerably more economical than traditional commercial SQL databases. This advantage is particularly relevant for startups and small-to-medium-sized businesses with limited budgets.
Lastly, the field of machine learning is increasingly benefiting from the integration of graph databases. Techniques like graph neural networks (GNNs) are becoming more prevalent, leveraging the underlying graph structure to uncover hidden patterns and relationships within data. This offers the potential for a more sophisticated form of predictive analytics.
The adoption of graph databases is rising as businesses and researchers grapple with complex data relationships. Though SQL remains a vital tool in many contexts, graph systems are becoming increasingly important, particularly for applications requiring faster querying, greater scalability, and a more intuitive way to interact with complex data relationships. This trend highlights the evolution of data management practices as technology progresses and challenges traditional approaches to data storage and querying.
More Posts from :