UC Berkeley's UX Design Program Introduces AI-Enhanced Portfolio Assessment System for Fall 2024 Cohort
UC Berkeley's UX Design Program Introduces AI-Enhanced Portfolio Assessment System for Fall 2024 Cohort - AI Algorithm Evaluates Student Design Case Studies Through 47 Key Performance Metrics
For the upcoming Fall 2024 cohort, UC Berkeley's UX Design program is implementing a new AI-driven system for evaluating student portfolios. This system analyzes student design case studies using a complex algorithm that considers 47 specific performance criteria. This is part of a larger trend within education to employ AI for tasks like assessment and feedback. Proponents suggest that this approach can help create more individualized feedback and potentially improve student learning outcomes by analyzing design quality in a systematic way. The hope is that through AI, learning is enhanced and the assessment process itself is improved.
However, it's important to recognize the potential downsides. Questions remain regarding the role of human judgment and oversight in such a system, and whether the quality of AI-generated feedback is sufficient. The increasing use of AI in higher education necessitates careful consideration of its impact on both students and the overall educational experience.
At the heart of this new system lies an AI algorithm that meticulously scrutinizes student design case studies using a remarkable 47 key performance metrics. This extensive set of metrics highlights the multifaceted nature of UX design, requiring a diverse array of skills and abilities. The significance of each metric is carefully calibrated based on its alignment with industry standards, which provides a granular and nuanced understanding of a student's capabilities.
The hope is that this approach lessens the influence of human biases inherent in traditional assessment methods, fostering a more objective analysis of each student’s work. Notably, the AI doesn’t merely analyze the final product; it also captures behavioral data during the design process, offering insights into how students approach problems and develop solutions. Essentially, the algorithm acts as a comparator, measuring student portfolios against a pre-established standard of industry benchmarks and thereby offering feedback that aligns with current market requirements.
Further, the expectation is that this method creates greater transparency in grading. Students will receive a clearer picture of where they stand in relation to the desired skillset and expectations. Because the AI system is founded on machine learning principles, it has the potential to continuously refine its evaluation processes as it ingests new data and feedback, dynamically adjusting to shifts in design practices and trends.
Early observations suggest that AI-powered feedback can actually boost student engagement in the learning process. The rapid nature of this feedback creates a framework for iterative learning, where students can swiftly react and improve upon their designs. However, while these 47 metrics provide a broad and comprehensive assessment, critics caution against over-reliance on AI. They contend that AI may struggle to capture the more nuanced, intuitive, and even intangible qualities of design that experienced human reviewers often readily grasp. This raises a crucial question of balance: how do we leverage AI while retaining the human element essential for a truly holistic understanding of a designer's abilities?
UC Berkeley's UX Design Program Introduces AI-Enhanced Portfolio Assessment System for Fall 2024 Cohort - New Portfolio Grading System Reduces Assessment Time from 2 Weeks to 3 Days
UC Berkeley's UX Design program is implementing a new portfolio grading system for the Fall 2024 cohort, promising a significant reduction in assessment time. Instead of the previous two-week turnaround, evaluations are now expected to be completed within a mere three days. This accelerated process relies on an AI-enhanced system that analyzes student design work, aiming for faster and potentially more accurate feedback. By prioritizing portfolios as a key indicator of individual learning and progress, the program intends to provide a more agile feedback loop that promotes continuous improvement. While the quicker feedback cycle is a positive development, it does raise concerns. Some may question whether AI can fully capture the complex and often subjective nuances of design work, potentially sacrificing some of the insights traditionally offered by human reviewers. Ultimately, the success of this streamlined system will hinge on finding the right balance between leveraging technology's efficiency and preserving the crucial human element within the educational process, ensuring that students receive both efficient feedback and a complete evaluation of their abilities.
The UX Design program at UC Berkeley has introduced a new portfolio grading system for the Fall 2024 cohort, which promises to drastically reduce the evaluation time. Instead of the usual two weeks, portfolios are now assessed within three days. This dramatic shift, representing a reduction of about 85%, is enabled by incorporating an AI-powered system. This change could streamline the grading process, allowing for quicker feedback loops and potentially accommodating a greater number of students.
This shift to AI-driven assessment aims for greater objectivity. The new system analyzes student work based on 47 performance metrics that are tightly connected to industry standards. Ideally, this approach minimizes biases inherent in human evaluations. However, this also raises concerns about the possible diminishing role of human assessment. While the AI system captures the students' final work, it also analyzes the design process itself, which could yield valuable insights into how students tackle challenges and develop solutions.
There's a clear emphasis on aligning the assessment process with current UX industry standards. The metrics used by the AI are directly related to the skills sought after in today's marketplace. This can make the feedback generated by the system directly applicable to real-world scenarios, which theoretically could boost the 'job readiness' of graduates. Interestingly, early observations suggest that the speed of the feedback loop provided by the AI may increase student engagement, offering a continuous learning cycle where students receive rapid, data-driven insights and react quickly to improve their work.
Yet, the trade-offs are worth considering. Some argue that the reliance on AI for these complex assessments might miss certain nuances inherent to design that are more easily picked up by trained human eyes. Essentially, the question becomes: can an algorithm capture the full spectrum of design talent, including aspects that are more intuitive and less easily quantified? The potential for oversight or bias in the AI's operation is a factor. Further, the use of AI for grading inevitably leads to discussions around data privacy and ethical use of student information. Ensuring that the collection and use of design and behavioral data remains transparent and protected is crucial. This new assessment method certainly showcases a move toward a more innovative, potentially more efficient approach to evaluating student work. However, it's important to consider the potential limitations and the need to strike a careful balance between AI-powered assessment and human insight in ensuring a well-rounded evaluation of a student's design skills.
UC Berkeley's UX Design Program Introduces AI-Enhanced Portfolio Assessment System for Fall 2024 Cohort - Machine Learning Model Trained on 15 Years of UC Berkeley UX Graduate Projects
As part of its innovative AI-enhanced portfolio assessment system for the Fall 2024 cohort, UC Berkeley's UX Design program has developed a machine learning model. This model is trained on a vast dataset encompassing 15 years of graduate student projects, effectively creating a historical record of design work within the program. The goal is to refine the AI's ability to evaluate student portfolios by providing it with a deeper understanding of successful UX design practices. By learning from past projects, the model can better identify specific skills and qualities that are highly valued in the field, allowing for a more detailed and accurate feedback process for current students.
While the use of machine learning offers the potential for faster, more objective evaluations, it also presents a challenge: can an algorithm fully capture the intricate and sometimes subjective aspects of design? Some might argue that certain qualities, like creativity and intuitive design sense, are difficult for AI to fully grasp. There's a concern that overly relying on AI might overlook the nuances that experienced human reviewers readily discern. Therefore, successfully integrating this technology into the assessment process will require a careful balancing act. The program must find a way to leverage the benefits of machine learning, such as efficiency and objectivity, while ensuring that the human element, vital for a truly comprehensive evaluation of a student's potential, remains a crucial part of the evaluation process.
The AI model at the core of this new portfolio grading system has been trained using a substantial dataset – 15 years' worth of UX graduate projects from UC Berkeley. This extensive historical record gives the model a unique perspective on how UX design has evolved over time, revealing trends and changes in design approaches and methodologies.
The model uses a comprehensive set of 47 metrics to assess student projects. This is noteworthy because it goes beyond just the visual elements, aiming to evaluate a wide range of aspects of design—from basic functionality and usability to the level of engagement a design might generate in users. Notably, the metrics are rooted in both research and real-world UX practices, suggesting a deliberate effort to make the system's feedback relevant to the current design landscape.
Beyond evaluating the final product, the AI system also analyzes the design process itself. This includes observing student behaviors as they work through projects. It’s intriguing that the system aims to capture how students approach problem-solving, a characteristic that's hard to measure objectively. This could provide insights into a student's design thinking and creative abilities.
One of the major advantages of this AI-driven approach is speed. The program claims it can slash evaluation time from a two-week process to a mere three days. The ability to give students rapid feedback can transform the learning experience, encouraging more iterative design practices where students can quickly respond to feedback and improve their work. This also suggests the system is designed to continuously learn and adapt. As it encounters more student work and feedback, the machine learning framework can potentially calibrate its assessment methods, ensuring they stay relevant to current design expectations and industry standards.
The use of AI also has the potential to streamline the evaluation process, allowing the program to scale more effectively. This could be particularly helpful for programs facing increasing enrollments in their UX design fields. The application of a data-driven approach to grading also seeks to mitigate biases that can be present in traditional grading methods. However, the use of AI in a field like design, where intuitive elements often play a major role, raises questions. While the AI system aims for objectivity, there's concern that it might miss the subtleties and nuances that experienced human reviewers might easily grasp.
Finally, the use of student data in this system necessitates careful attention to ethical considerations. The collection and use of both design work and student behavioral data require transparent policies and procedures to ensure responsible handling. It will be fascinating to observe how the use of AI within a UX program unfolds and the impact it will have on students and the future of UX design education.
UC Berkeley's UX Design Program Introduces AI-Enhanced Portfolio Assessment System for Fall 2024 Cohort - Real Time Feedback System Guides Students Through Portfolio Development Phase
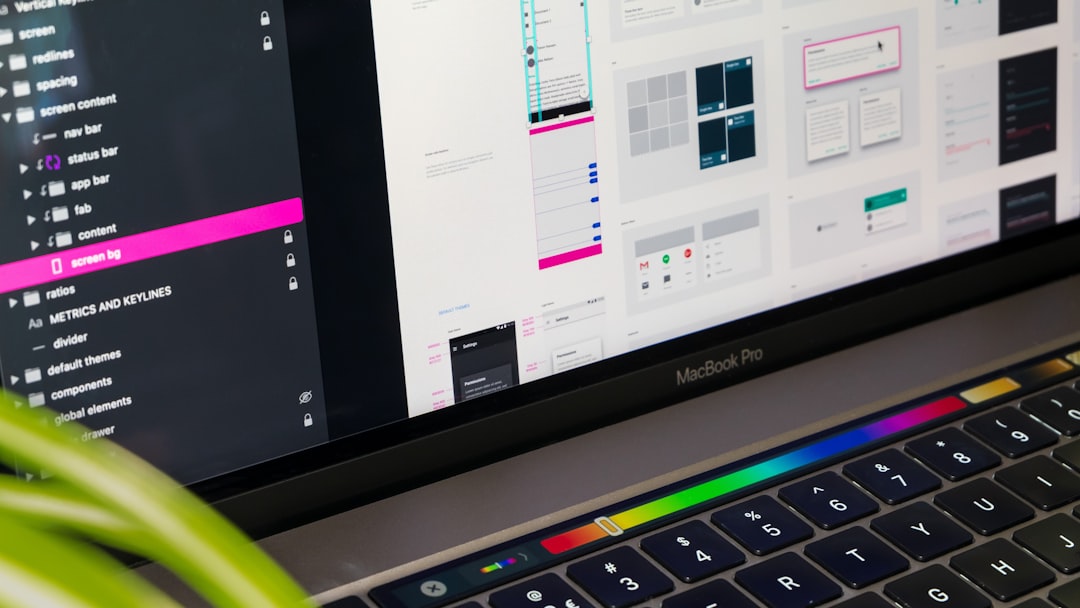
The UX Design program at UC Berkeley is introducing a real-time feedback system for its Fall 2024 cohort, designed to help students navigate the often-challenging process of creating their design portfolios. This new system provides ongoing feedback as students develop their work, encouraging them to refine their projects and think more deeply about their design choices. The program views the portfolio as a crucial tool for tracking student growth and progress, a trend that highlights how portfolios are becoming increasingly important in evaluating students' learning and achievements. This method aims to improve students' critical thinking abilities and reduce the anxiety that traditional assessments can trigger. However, there are worries that the new system, in its focus on data and metrics, might not fully capture the more subtle and creative aspects of design, which are typically well-evaluated by experienced human reviewers. Ultimately, this underscores the importance of finding a balance between the efficiency of this automated feedback and the value of human judgment in ensuring a well-rounded evaluation of each student's design skills and potential.
The UX Design program at UC Berkeley has integrated a new AI-powered system for assessing student portfolios, aiming to streamline the feedback process and potentially enhance learning outcomes. This system leverages a machine learning model trained on 15 years of past student projects. This extensive historical dataset provides the AI with insights into the evolution of UX design practices and successful project characteristics.
This assessment system uses 47 key performance metrics to evaluate student work in a multi-faceted manner. The system goes beyond merely assessing the final design product, exploring how students approach design challenges, a vital component of the creative process. This data can shed light on a student's problem-solving skills and unique design thinking.
Perhaps the most notable aspect is the dramatic reduction in grading time, from the prior two weeks to a rapid three days. This accelerated feedback loop, a reduction of approximately 85%, allows students to iterate more quickly on their designs, leading to a more dynamic learning process. Furthermore, the metrics employed by the system are closely linked to current industry standards, suggesting a deliberate focus on real-world applicability of the skills being taught. This hopefully translates to graduates with better job readiness.
The AI algorithm can also adapt and refine its own criteria over time. As it's exposed to a greater number of student projects and receives feedback, it can progressively optimize its assessment methods. The hope is that this objectivity in the AI system can reduce biases that might otherwise be present in traditional evaluation methods, thereby ensuring a more equitable assessment environment.
However, there are also legitimate concerns regarding the capabilities of the AI. It remains to be seen whether the system can fully capture the nuanced aspects of design that experienced human evaluators typically grasp, including aspects of creativity and intuition, which are less quantifiable. It's essential to acknowledge this potential limitation.
Beyond the technical aspects, the program must also address data privacy issues. The AI's analysis of student work, including design process behavior, raises legitimate concerns about ethical handling of sensitive student information. Transparency and strict adherence to established privacy guidelines are vital to allay these concerns and ensure student trust in the program's methods.
This initiative at Berkeley represents a significant move towards integrating AI into design education. It's an intriguing development and the potential impact on students and the future of UX design education will be fascinating to observe. While the speed and objectivity offered by the system are attractive, careful consideration must be given to its limitations and ethical considerations to ensure that it effectively serves the needs of students.
UC Berkeley's UX Design Program Introduces AI-Enhanced Portfolio Assessment System for Fall 2024 Cohort - Cross Referenced Assessment Between AI System and Professional UX Designers
The UC Berkeley UX Design Program's new portfolio assessment system, debuting in Fall 2024, introduces a novel approach by cross-referencing evaluations from an AI system and experienced UX designers. This dual assessment aims to improve the speed and impartiality of evaluations, while retaining the capacity to understand the subtle and creative aspects of design that are often better perceived by human professionals. The AI component leverages a set of 47 key performance metrics to provide prompt feedback on student design projects, which in turn, can lead to quicker design iteration and improvement for students. However, there are worries that relying too heavily on an AI-driven assessment might cause a loss of the nuanced understanding and creative judgment that human UX designers bring to the process. The success of this approach hinges on carefully managing the tension between the efficiencies offered by technology and the irreplaceable role of human experts in evaluating the full spectrum of design talent.
The AI system being introduced at UC Berkeley's UX Design program leverages a unique training dataset—15 years of graduate projects—to gain a deep understanding of how UX design standards have evolved. This historical perspective allows the AI to learn from successful design patterns and better identify valuable skills in student portfolios.
Furthermore, the machine learning foundation of this system allows it to continuously adapt and refine its evaluation methods. As the AI receives and analyzes new student submissions, it's expected to adjust to changing industry trends and design practices. This self-improving aspect promises a dynamic and modern assessment method.
One of the most striking features is the shortened portfolio review time. Instead of the usual two weeks, portfolios are assessed in just three days. This significantly reduced timeframe not only streamlines the grading process but also promotes a more iterative learning cycle for students. They can receive rapid feedback and adjust their designs quickly, which can encourage a more dynamic and engaged learning process.
Beyond just the final design, the AI can analyze the design process itself. This means it can gain insights into how students approach problems and think through their design decisions, providing a window into their problem-solving skills and creative processes. These "behind-the-scenes" observations can offer a more complete picture of a student's abilities beyond simply the final outcome.
To facilitate a structured and industry-aligned assessment, the system utilizes 47 performance metrics closely related to current UX job market requirements. This framework provides students with concrete benchmarks for their skills and gives them a clear understanding of how their abilities align with the needs of potential employers.
The adoption of AI in assessment aims to reduce the impact of human biases that can sometimes influence traditional grading methods. By using data-driven metrics, the system strives for a more objective evaluation, leading to a fairer and potentially more consistent assessment environment for all students.
However, a common concern is whether the AI can capture the more intangible, creative elements of UX design. While AI excels at objective metrics, some believe that aspects like creativity and intuitive design sense are difficult for algorithms to grasp. Seasoned human evaluators typically excel in assessing these nuances, raising questions about the overall capacity of the AI model.
This new system further highlights a growing trend in design education, where portfolios are increasingly becoming the primary way to demonstrate student progress. This shift suggests that how we evaluate design skills in academia is evolving, with portfolios taking on a more central role.
Finally, the use of AI to evaluate design work naturally raises questions about data privacy. The system collects information about the student design process and the work itself, making it crucial that appropriate measures are taken to protect student data and ensure transparency about how it is used. This is essential to maintaining student trust in the system and the program.
Early findings suggest that this system, with its faster feedback cycle, might lead to more student engagement. Students could find themselves more actively invested in the design process, anticipating quick feedback and proactively seeking to refine and improve their work based on immediate results. This heightened student engagement could foster a more productive and energized learning environment.
UC Berkeley's UX Design Program Introduces AI-Enhanced Portfolio Assessment System for Fall 2024 Cohort - Portfolio Database Integration Connects Students With 200 Bay Area Tech Companies
UC Berkeley's UX Design program has integrated a new portfolio database system, aiming to connect students with over 200 technology companies based in the Bay Area. This connection intends to facilitate a smoother transition from the classroom to professional opportunities. The program recognizes the value of showcasing students' design work and projects to potential employers, suggesting a growing need to bridge the gap between theory and practice in UX design. By giving students a broader reach within the Bay Area tech industry, the program hopes to increase the visibility of its graduates and potentially improve employment outcomes. However, for this initiative to truly succeed, it's crucial that the program develops robust and meaningful partnerships with these tech companies. These relationships need to provide students with substantial networking and project opportunities, and ensure that the students' skills are indeed in line with the real-world needs of the companies. The program's efforts to bolster student career readiness are commendable, but ultimately, the success of the portfolio database integration will rely on the quality of the connections it fosters and the degree to which they lead to beneficial outcomes for both students and the companies involved.
The UX Design program at UC Berkeley is leveraging a new portfolio database that connects students with over 200 technology firms based in the Bay Area. This connection potentially opens up a variety of opportunities for students, such as internships, collaborative projects, and potential job placements. This integration aims to bridge the gap between academic learning and the practical world of UX design, which is a good thing in theory.
It's interesting that they are using a real-time feedback system in conjunction with portfolio assessment. This allows students to get immediate responses to their design work, encouraging faster design iterations and potentially fostering a more dynamic learning environment. It will be insightful to see whether this approach truly increases student engagement, especially when compared to traditional portfolio review practices, which often include a significant delay in feedback.
The assessment itself utilizes 47 distinct performance metrics. This extensive set of metrics aims to give a detailed overview of a student's capabilities, covering a range of design aspects like functionality and user interaction. It will be important to see how this multi-faceted approach compares with the assessments from the past.
Furthermore, the AI algorithm behind this system has been trained using a considerable dataset spanning 15 years of UX graduate projects from UC Berkeley itself. This extensive historical perspective gives the algorithm a nuanced understanding of design trends, providing potentially valuable insights into the progression of UX practices. The degree to which this model captures the actual evolution and nuances of design will be something to monitor.
Interestingly, the metrics employed in the AI-based evaluations are closely aligned with current UX industry standards. This alignment potentially translates into a more practical and relevant education for students, making them better prepared for entry-level UX jobs. It will be helpful to track if there is evidence of improved "job readiness" with graduates from this program, relative to past cohorts or other similar programs.
In addition to the final design outcome, the system captures data about a student's approach and behavior during the design process itself. This includes things like problem-solving strategies and their workflows. This type of behavioral analysis offers a unique perspective on the creative process, something that's often difficult to evaluate objectively. This level of granularity could be quite valuable, especially if the information can be analyzed without jeopardizing student privacy.
This AI-driven system is also designed to be dynamic and adaptive. As it receives and analyzes more data, the system can refine and improve its evaluation methods. It will be interesting to see how well it manages to adapt to the rapidly changing landscape of UX design trends and the demands of the UX job market.
One aspect of this program that seems unique is the combination of AI assessment with evaluations from human UX designers. This approach aims to strike a balance between the advantages of fast, data-driven feedback and the more nuanced understanding and creative judgment that human reviewers can provide. It will be critical to observe how the different perspectives and judgments of the AI and human reviewers are reconciled or combined to form an overall grade.
However, it's worth noting that there are potential ethical implications regarding the collection and use of student data. The program needs to develop and enforce clear policies that protect students' privacy and are transparent about how their information is being used. The need to avoid ethical pitfalls is paramount to preserve the trust of current and prospective students.
The reduction in evaluation time from two weeks down to just three days is a significant change. While this can significantly streamline the assessment process, allowing the program to handle a larger number of students or potentially offer a more frequent cycle of feedback, it will be important to ensure that such a quick turnaround does not diminish the overall quality and rigor of the evaluation process.
This new AI-enhanced assessment system definitely represents a potentially beneficial evolution in UX design education. However, it's crucial to closely evaluate the performance and effectiveness of this new approach to determine its ultimate impact on student outcomes, the field of UX design, and educational practices more broadly.
More Posts from :