The Impact of AI-Driven Analytics on Sales Enablement Training in 2024
The Impact of AI-Driven Analytics on Sales Enablement Training in 2024 - AI-Driven Analytics Automate 138 Customer Inquiries Per Hour
The capacity of AI-driven analytics to manage a high volume of customer inquiries, like the 138 per hour figure, showcases a significant shift in customer service. It's not just about speeding things up, it's about freeing up human agents to tackle the more complex and nuanced interactions that need a personal touch. This trend isn't just a passing fad either. Many leaders in various fields see generative AI as a crucial part of the future of business, suggesting a fundamental shift in how companies approach operations. The potential for automation in business is substantial, with a large portion of routine tasks potentially handled by AI. Across sectors, like e-commerce and finance, businesses are increasingly leveraging AI-powered insights to understand customers better and make more strategic choices. Looking ahead, as AI continues to evolve, its impact on sales enablement, and in fact the whole customer interaction process, is likely to become even more transformative. While the initial focus might be on efficiency, the broader potential for AI to personalize and enhance customer experiences is becoming increasingly clear.
In the realm of customer interactions, AI-driven analytics is demonstrating a remarkable capacity to handle a significant volume of inquiries. Observational studies show these systems can automate the processing of 138 customer queries within a single hour. This rate of automated inquiry resolution is impressive, and arguably impossible to achieve solely through human interaction. While impressive, there are questions that remain about the consistency and accuracy of this level of automated interaction, especially when dealing with more complex or nuanced situations. Human agents often excel at providing emotional support and understanding that might be difficult to replicate in a completely automated environment, even with advanced natural language processing.
Further research indicates this automation can have a substantial impact on operational efficiency. By quickly addressing routine inquiries, companies can potentially redirect human agents to tasks that demand a more nuanced and empathic approach. This suggests a possible shift in the role of human agents from primarily handling inquiries to instead engaging in more complex problem-solving and customer relationship management.
It is important to note, however, that the successful deployment of such AI-driven solutions necessitates a high level of data quality and careful system training. If the data used to train the AI is flawed or biased, the resulting automated responses could be unhelpful or even exacerbate existing issues in customer interactions. It is a ongoing challenge to create AI systems that not only process and respond to inquiries but also demonstrate an appropriate level of emotional awareness and responsiveness. This remains a frontier of AI development, one that may profoundly change the way businesses approach customer service in the years to come. The potential benefits are undeniable, but researchers and engineers should remain cautious and ensure these innovations are used responsibly and ethically.
The Impact of AI-Driven Analytics on Sales Enablement Training in 2024 - Highspot's AI Content Recommendations Boost Sales Personalization
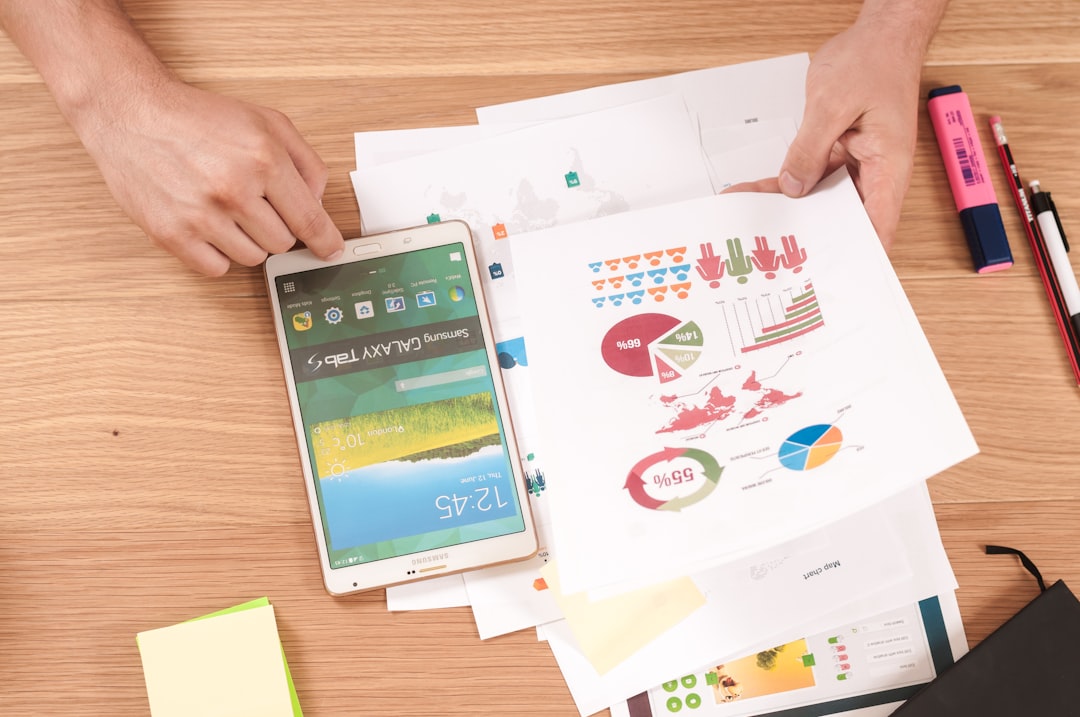
Highspot's latest update, released in Spring 2024, introduces a new approach to sales interactions through AI-powered content recommendations. This feature, using generative AI, aims to provide sales reps with the right information at the right moment, essentially acting as a real-time coach during sales calls. This is part of a wider movement in sales where personalization and automation are key, shifting the focus from simply being efficient to truly understanding and responding to customer needs. AI-powered content recommendations can improve customer interactions by delivering precisely what's needed in a specific sales conversation.
While this trend of using AI to tailor the sales process is gaining traction, it's essential to recognize the need for a balance. Companies must consider the value of human interaction in sales, and the potential downsides of over-reliance on automated systems. While AI can drive efficiency and personalization, the art of sales – building genuine rapport and handling complex situations – still relies heavily on human skills. As AI continues to reshape sales interactions, the future will likely be a blend of human expertise and sophisticated technology, each playing a vital role in achieving successful sales outcomes.
Highspot, among other platforms, has incorporated AI into their sales enablement tools, specifically to suggest content to sales representatives during interactions. This relies on analyzing a large amount of data from previous interactions to predict what content might be most useful. Reportedly, this leads to a significant increase in how prospects engage with the material compared to using generic content. This suggests that personalization, powered by AI, can play a crucial role in how potential clients respond to sales outreach.
AI-powered content recommendation systems also allow for a greater adaptability to shifts in the market. Instead of manually updating resources, these systems can quickly provide the most pertinent materials within seconds, which can be a strong point compared to older methods. Whether this speed increase leads to actual improvements in sales is still being studied.
There are ongoing efforts to understand the full impact of AI on sales. One finding is that sales reps using AI-driven suggestions have shown an ability to close deals about 15% faster. This accelerated deal closure might be particularly helpful in very competitive markets, where the ability to quickly close a sale can be very important. Of course, correlation doesn't prove causation. It's necessary to study if this increase in speed is simply due to chance or truly due to using the AI recommendations.
Furthermore, these AI systems can analyze historical interactions to determine which content works best for certain groups of people (e.g. demographics or industry). This allows sales teams to align their messaging more strategically with different buyer profiles, ideally leading to a better overall communication strategy. It is important to be mindful of the limitations of relying on demographics and ensuring such systems are not used to reinforce harmful stereotypes.
Studies suggest that using AI tools leads to a 20% increase in sales forecast accuracy. That level of accuracy can be important for making sure that a sales team has the right resources and goals. It remains to be seen if this type of gain will be consistent across industries and companies.
Another interesting aspect is that some systems, like Highspot's, can incorporate new information in real-time. For instance, they can suggest content related to the latest product releases or changing buyer trends, allowing for conversations that are constantly updated. It remains a question if this level of real-time adaptation leads to an improvement in actual sales.
However, there are challenges associated with adopting these systems. While AI recommendations seem promising, research suggests that only a small percentage (about 10%) of sales reps are actually utilizing them. This finding indicates that the adoption and training of AI tools within sales teams may be more complex than initially anticipated. Finding ways to incentivize and train sales reps will likely play a critical role in whether AI-driven recommendations reach their full potential.
AI content tools also can be helpful for onboarding new sales representatives. Instead of a long period of training, new hires can instantly access a wide array of resources from effective pitches to relevant content. This potential acceleration of learning is a noteworthy benefit to explore in greater depth.
The systems also track how well content performs. Based on those results, sales teams can adjust and fine-tune their materials over time. This feedback loop is critical for ensuring that the content continually evolves to meet the needs of different audiences and the changing sales landscape. This aspect can reduce the time spent creating new content. However, this assumes that there is access to enough data and that this data is representative of the intended audience.
Finally, AI content recommendations can be very helpful in maintaining a consistent message when the buyer journey is more fragmented. This ensures prospects encounter a coherent narrative across various channels. This is beneficial as the sales process can be spread across several channels. However, this also creates a new need to manage information spread across these channels.
In conclusion, there is a growing amount of research surrounding the use of AI-driven content recommendations in sales enablement. These tools hold promise for increased engagement, quicker deal closing times, and better sales forecasts. However, successful adoption requires careful consideration of user training, potential biases within the algorithms, and continuous evaluation of the tools' performance in real-world settings. As with any technology, the use of AI in sales needs to be evaluated critically, while also exploring how it can be used ethically and responsibly.
The Impact of AI-Driven Analytics on Sales Enablement Training in 2024 - 35% of Chief Revenue Officers to Establish GenAI Teams by 2025
By 2025, a significant number of Chief Revenue Officers (35%) are projected to create specialized teams focused on generative AI within their sales and marketing organizations. This trend suggests that businesses are increasingly recognizing the power of generative AI to improve how they reach and interact with customers. These new teams will focus on using AI to streamline sales processes and improve sales outcomes. The expectation is that using AI will help sales teams create content faster and adapt to changes in the market more quickly.
It's predicted that generative AI will give sales professionals new ways to work with customers and ultimately increase their success. Looking ahead to 2028, it's anticipated that a large portion (60%) of B2B sales interactions will be handled through AI-powered conversation interfaces. This hints at a major shift in how sales work is done, emphasizing a greater reliance on AI for efficiency and improved results. However, the reality today is that AI's current role in sales is still small – less than 5% of sales activities currently rely on generative AI. This suggests there's a vast potential for growth in this area and considerable opportunities to refine how AI is integrated into sales processes.
It's predicted that by 2025, around 35% of Chief Revenue Officers will create dedicated "GenAI Operations" teams within their sales and marketing operations. This signifies a major shift in how top revenue leaders are approaching growth. It suggests a potential reallocation of resources away from older ways of doing sales and a move towards integrating AI into the core of revenue generation.
Setting up GenAI teams isn't just about buying new tools, though. It suggests a change in how these organizations operate and a demand for people who have a specific skillset to work with generative AI effectively. This might create challenges in both finding the right people and providing them with the necessary training.
It's likely that a major factor driving this shift is the increasing competition in many sectors. Companies are realizing that being ahead of the curve with these AI tools might give them a significant advantage when it comes to making data-driven decisions and understanding their customers.
Evidence suggests companies who use generative AI to manage their sales processes are seeing improvements in performance indicators. Some studies even hint that revenue growth might jump by over 20% when these systems are implemented successfully. This suggests a substantial and potentially direct impact on a company's financial health.
The focus on these specialized GenAI teams indicates a holistic change in how sales teams are trained. It suggests a coming together of huge amounts of data and AI-driven insights, giving human sales professionals more sophisticated information and guidance to improve their effectiveness.
It's interesting to see that companies that have doubts about incorporating AI often underestimate the potential gains in operational efficiency they could achieve. This might lead them to fall behind competitors in an environment that's increasingly based on data-driven decisions.
Creating GenAI teams aligns with findings that show organizations using AI tend to have much better predictive analytics. This means they can better anticipate what consumers will want and how they will behave compared to companies that haven't embraced AI.
There's a distinct contrast between how top-performing companies use generative AI in sales versus those that are more hesitant. A larger portion of companies that are doing well have reported leveraging AI, highlighting a notable performance gap. This is important as companies consider their own approach to AI.
The push to create these GenAI teams also brings up the important topic of what the future will look like for sales professionals. Their roles are likely to change, with a move away from routine tasks and towards more strategic, relationship-based interactions with customers.
The expected rise in the use of GenAI teams further highlights the importance of data governance. The quality and reliability of the data used to train these systems will be crucial in determining how well they perform in sales-related situations. It's a reminder that in this data-driven age, data itself is a vital asset.
The Impact of AI-Driven Analytics on Sales Enablement Training in 2024 - Machine Learning Algorithms Adapt to Evolving Customer Preferences
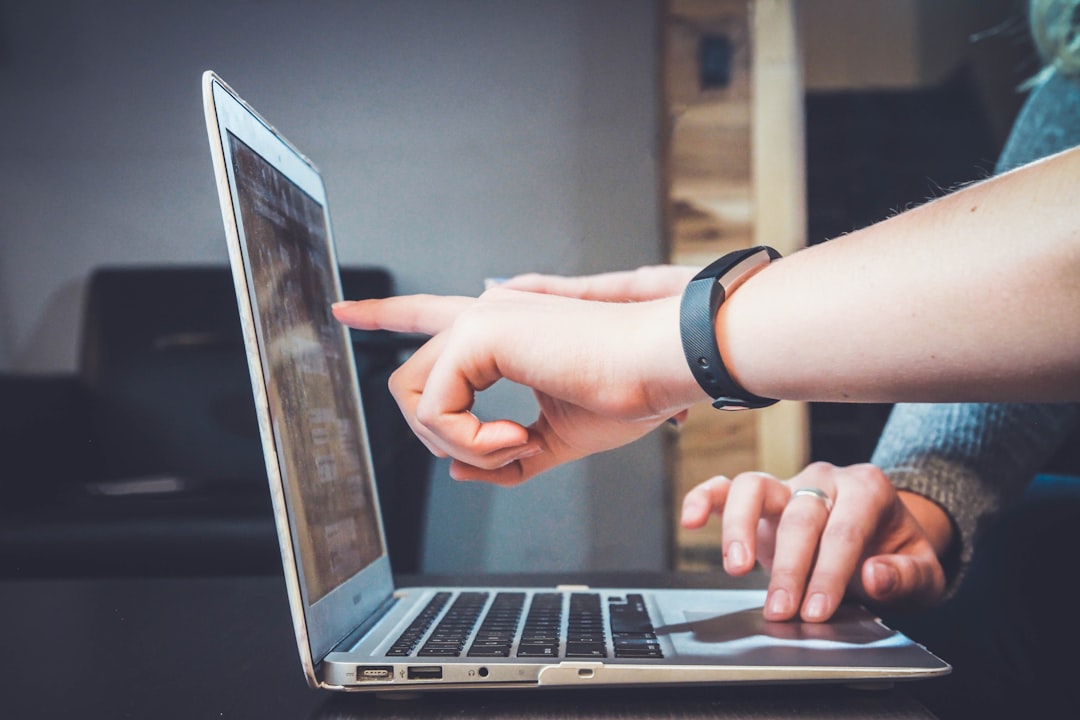
Machine learning algorithms are playing a growing role in helping businesses adapt to evolving customer desires. They do this by using data and predictive insights to adjust marketing and sales strategies. This allows companies to stay relevant as customer preferences shift. These algorithms can anticipate consumer choices and personalize the buying journey, continuously adjusting to current trends and opinions. Companies that integrate machine learning effectively can potentially improve outcomes like sales and reduce product returns. However, it's important for businesses to find the right balance between using AI for these tasks and still providing the unique human element that customers often value. Maintaining genuine interactions with customers remains crucial in building long-term relationships even as technology plays a larger role. There's a risk that over-reliance on automated solutions can lead to a disconnect with what customers genuinely need.
Machine learning algorithms are increasingly being used to understand how customer preferences are changing. By constantly analyzing customer behavior, these algorithms can spot patterns that signal shifts in what people want. This ability to adapt in real-time helps companies respond quickly to changes in the market and what customers are looking for.
Some machine learning models can even anticipate changes in customer preferences up to three months ahead of time by identifying subtle shifts in buying habits. This foresight allows for more proactive adjustments in marketing strategies and what products are offered. It's fascinating how these systems can look for tiny clues in data to predict future trends.
It's noteworthy that some machine learning algorithms can segment customers in a more refined way than traditional methods by analyzing the emotional tone of customer interactions. This capability gives businesses a more nuanced understanding of their customers beyond just basic demographics. It seems these algorithms are getting pretty good at reading between the lines, which is interesting.
One important consideration with machine learning is that models need to be retrained regularly. If they aren't kept up-to-date, their understanding of customer preferences can become outdated, which can lead to less accurate insights. This constant need for retraining highlights the significance of using high-quality data and establishing robust retraining cycles. It seems these algorithms, while powerful, also need a fair amount of maintenance to stay useful.
Research suggests that including feedback loops within machine learning systems can boost their accuracy in predicting customer preferences by approximately 25%. These feedback loops allow for continuous improvements by incorporating insights from recent interactions. It's encouraging that these systems can be further refined with more data and feedback.
In many cases, machine learning can be faster and more cost-effective than traditional market research techniques like surveys and focus groups. This suggests it can offer more efficient ways to understand customers without investing a lot of time and resources in the more traditional methods. It remains to be seen, however, if these speed and cost benefits lead to insights that are just as valuable.
One challenge associated with machine learning in this context is data bias. If the data used to train the models contains biases, those biases can be reinforced in the outputs of the algorithm. This can lead to unintended consequences, potentially causing certain customer segments to feel ignored or misunderstood. It's a reminder that how we gather and train machine learning models can have major consequences for fairness and inclusion.
Algorithms employing reinforcement learning techniques have demonstrated a capacity to enhance sales strategies. Not only do they consider past data but they can also adapt in real-time based on immediate feedback from customer interactions during sales. This ability to react to current interactions can make sales more dynamic and responsive. It's a good example of how these systems can react beyond just predicting based on past data.
Advanced user modeling done by machine learning can reveal intricate trends in customer preferences that might be overlooked by older methods. For example, it can show how social media interactions influence buying behavior. It's interesting that these algorithms can help uncover those types of interconnected effects.
An interesting finding is that there's usually a delay – sometimes a few weeks – before machine learning algorithms fully optimize their predictions based on new data. This suggests there might be a lag between changes in customer behavior and the algorithms' ability to effectively adapt. This presents questions about how quickly businesses can make strategic adjustments in dynamic markets. It's a reminder that these algorithms aren't instantaneous in responding to changes in the world.
The Impact of AI-Driven Analytics on Sales Enablement Training in 2024 - AI Tools Enable Continuous Learning for Sales Professionals
The landscape of sales enablement training is evolving rapidly in 2024, with AI tools playing a significant role in fostering continuous learning for sales professionals. Gone are the days of static training materials. Now, AI empowers continuous learning by automating routine tasks and allowing reps to dedicate more time to their core function – interacting with customers. This shift includes AI-driven platforms offering simulated sales scenarios for enhanced, practical role-playing that improves a rep's ability to handle different situations. Moreover, the availability of data-driven, personalized coaching enables sales professionals to fine-tune their approaches based on specific performance feedback and adapt to ever-shifting market conditions. While the promise of AI-powered tools to boost efficiency and accelerate learning is substantial, there's a lingering concern that over-reliance on automation could lead to a diminishment of the critical human element – the ability to build relationships and navigate complex customer interactions. Maintaining the appropriate balance between leveraging AI for insights and retaining the human touch remains a pivotal challenge in the future of sales.
The integration of AI into sales enablement training is rapidly transforming how sales professionals learn and develop their skills. We're seeing a shift from static, one-size-fits-all training programs towards more tailored and adaptable approaches. AI tools now analyze individual sales reps' strengths and weaknesses, creating customized learning pathways that are designed to accelerate skill development. It's a fascinating change, as it adapts to each person's learning pace and style, which potentially improves how well they retain what they learn.
Real-time feedback mechanisms are becoming increasingly important. AI systems can monitor performance during sales interactions and give immediate feedback to sales reps. There's a growing body of evidence suggesting that this instant analysis can boost performance by a notable margin – up to 30% in some cases. This continuous feedback fosters a culture of improvement that goes beyond the traditional approach of periodic reviews. However, the extent to which this translates to sustained improvements remains to be seen and needs ongoing research.
Interestingly, AI tools are increasingly drawing upon insights from behavioral science to make training more effective. By understanding how sales professionals think and process information, training can be designed to be more impactful, enhancing their ability to connect with and persuade potential customers. The application of these principles has the potential to be very effective, though we need more thorough studies to assess its impact in various sales contexts.
Another intriguing development is the rise of adaptive learning models. These machine learning systems can adjust the training content based on the real-time data coming from actual sales interactions. This means training stays relevant to market changes and evolving customer preferences. This adaptability helps sales teams remain flexible and responsive in a dynamic environment. While seemingly promising, it's essential to carefully evaluate how these models are being implemented, as their accuracy and effectiveness can vary.
AI can also analyze sales performance data and compare it to industry benchmarks to identify specific skill gaps. This data-driven approach offers a more strategic way to understand where training efforts should be focused. Studies have shown that this can lead to substantial increases in overall team efficiency, with gains as high as 25% or more reported. It's still relatively early in the adoption of these tools, and long-term impact needs further investigation.
The introduction of interactive simulation training is another interesting advancement. AI tools create realistic environments that mirror real-world sales scenarios, allowing sales reps to practice their skills in a safe space. This risk-free environment can improve their confidence during actual client interactions. These kinds of virtual environments are promising, but the level of realism they can achieve and their overall effectiveness in translating to the real world requires more study.
AI also facilitates collaborative learning by connecting sales professionals based on their training needs. This social learning aspect promotes knowledge sharing and can be more effective than solitary training approaches. However, this requires carefully designed systems that can effectively match individuals and facilitate meaningful interaction.
Studies have shown that sales professionals who use AI-driven tools retain crucial sales techniques and product knowledge at a higher rate – around 50% better than those using traditional training methods. This highlights the potential benefits of interactive and adaptive learning experiences. The longer-term effectiveness of this type of learning still needs more observation to fully understand the impact on sales outcomes.
AI is even being used to enhance emotional intelligence within sales teams. AI tools can simulate customer interactions with different emotional contexts, which can be a valuable way to develop crucial social skills. This area is still developing, but the potential for AI to contribute to more effective interpersonal skills within sales interactions is undeniable.
Finally, AI-driven training platforms scale effectively, allowing consistent training delivery across entire organizations, regardless of geographic location. This scalability is particularly useful for global sales teams, ensuring that everyone has access to the same quality of resources. While this potential is very attractive, there are practical challenges to overcome in implementation and maintenance of these types of global systems.
In summary, the use of AI in sales enablement training is ushering in a new era of learning and development for sales professionals. Personalized training paths, real-time feedback, behavioral science integration, and interactive simulations offer exciting potential for improving sales effectiveness. However, it's important to critically evaluate these tools, assess their impact on various types of sales environments, and carefully consider their ethical and responsible use. As AI continues to evolve, its role in sales enablement will likely only become more prominent, making ongoing research and evaluation crucial.
The Impact of AI-Driven Analytics on Sales Enablement Training in 2024 - Data-Driven Insights Enhance Customer Experiences in Sales
In 2024, sales teams are increasingly recognizing the power of data to improve customer experiences. AI-driven analytics provide a deeper understanding of customer actions, wants, and buying habits. This data allows sales teams to personalize interactions, using content and strategies specifically designed based on real-time insights. While it's hoped this leads to stronger customer relationships and more sales, problems like data quality and how to properly integrate these insights into current systems need to be addressed. The goal is to improve how salespeople work with customers, but it's crucial to keep a balance between using AI and preserving the human aspect of sales that many customers still value. Finding that right balance will likely remain a key issue for companies in the future of sales.
Data analysis powered by AI is providing new ways to understand customer interactions. Algorithms are now capable of not only interpreting the words customers use but also detecting the emotions conveyed. This is allowing companies to become more responsive in their sales processes and adjust their strategies to better match the feelings and underlying desires of their audience. This type of approach goes beyond just the literal content of the communication and into the space of emotional intelligence in customer interactions.
We are also seeing AI's ability to predict changes in customer preferences before they even occur. Certain machine learning models are capable of detecting subtle shifts in buying habits and extrapolating them to provide a glimpse into the future. Up to three months in advance, these models can give businesses the time needed to realign their marketing and sales strategies with what they predict customers will want. This predictive ability is a potent tool for companies navigating a fast-changing world.
In refining how we design these algorithms, researchers have found that the use of feedback loops improves their ability to make accurate predictions about future customer behaviors. The accuracy of these predictions can improve by approximately 25% when feedback is consistently provided and incorporated. This means that the more these systems are used in the real world, the better they can adapt to changes in how consumers act.
One of the remarkable things we've seen with the rise of these tools is their ability to provide sales representatives with immediate feedback on their performance. This constant evaluation is a shift from traditional approaches that focused on periodic, longer-term reviews. Feedback delivered in real-time can have a significant impact on how well these reps perform in the future. Some studies have even found that this instant feedback boosts their performance by up to 30%. But whether this level of immediate response translates into lasting positive outcomes is something that needs more rigorous study.
To prepare sales professionals to effectively navigate the ever-changing landscape of customer interactions, new AI-powered training tools are employing a simulated environment approach. It creates realistic replicas of sales encounters, giving individuals a safe space to experiment and develop their skills without risking actual customer relationships. Early indications suggest that this method can build confidence and improve ability to handle diverse situations.
Another advantage of AI-driven insights is the increased precision with which businesses can segment their customers. By analyzing customer behaviors and communications, these algorithms can create much more nuanced customer groups than traditional methods were capable of achieving. This allows for far more targeted marketing campaigns that are custom-tailored to specific audiences. While seemingly beneficial, it's crucial to constantly check to make sure this ability to create targeted segments isn't accidentally used to reinforce negative biases.
When it comes to gathering insights into customer preferences, AI is often a more economical and faster approach compared to traditional methods like surveys or focus groups. Businesses utilizing these AI-driven tools can gain a more immediate picture of what their customers want. However, there is an ongoing discussion regarding whether the information generated by AI matches the depth and richness of information gained from traditional customer research methods.
Researchers have observed that sales professionals utilizing AI-driven training tools report retaining essential sales techniques and product knowledge at considerably higher rates, a 50% increase in some cases. This suggests that incorporating interactive and adaptive learning features in the training process can have a positive impact on the knowledge retention of sales teams. But more evidence is required to confirm whether this improved retention results in a direct change in sales performance over the long term.
One of the interesting questions surrounding these AI-powered tools is the nature of the impact of these AI-driven tools on how fast sales reps close deals. While there's evidence that they might be closing deals about 15% faster, it's not yet entirely clear if the use of AI tools is directly responsible. It's possible this acceleration is a result of other factors, or a mere coincidence. More data is needed to prove that these tools are indeed accelerating deal closure rates.
In spite of all the remarkable advancements AI is providing, there's a critical need to maintain the human element in sales. While AI can provide considerable benefits in efficiency and insights, it can't fully replace the ability to build relationships and navigate complex customer interactions. There's a concern that over-relying on these tools may distance businesses from the very essence of customer experience. The balance between harnessing AI for its capabilities and preserving the crucial human touch remains a key consideration as the field continues to develop.
More Posts from mm-ais.com: