The Evolution of Reverse Image Search From Pixels to AI-Powered Recognition in 2024
The Evolution of Reverse Image Search From Pixels to AI-Powered Recognition in 2024 - From Pixel Matching to Deep Learning The Technical Leap
The shift from basic pixel comparisons to the intricate world of deep learning represents a pivotal moment in reverse image search. Platforms like PicTrace and others are at the forefront of this change, harnessing computer vision and neural networks to provide more accurate and relevant image identification. The advent of convolutional neural networks (CNNs) has drastically improved the speed and precision of image tasks, like segmenting or classifying objects. This has not only made finding similar images easier but also opened up new possibilities across numerous industries. From enhancing e-commerce browsing to transforming the portrait photography process, AI is changing the way images are processed and interpreted. These developments inevitably prompt questions about the future landscape of photography, particularly concerning the role of human photographers in a world increasingly dominated by artificial intelligence.
The limitations of older reverse image search methods, rooted in simple pixel comparisons and geometric manipulations, became evident as they struggled to cope with changes in lighting, angles, and other image variations. These systems often produced disappointing results, highlighting the need for a more sophisticated approach. Deep learning, particularly through convolutional neural networks (CNNs), brought a paradigm shift. CNNs, with their ability to automatically learn intricate image features, surpassed the accuracy of pixel-matching techniques.
Rather than relying on meticulously designed features, as earlier systems did, deep learning empowers algorithms to sift through extensive image collections and uncover complex patterns, effectively removing the reliance on manual feature engineering. This progress has had a notable impact on areas like AI-generated headshots, where increasingly realistic portraits can be produced using advanced AI techniques.
This rapid development also raises questions about authenticity in an era where digital personas can be constructed with uncanny precision. The cost implications are also significant; with AI-powered image generation tools capable of producing high-quality images at a fraction of the traditional cost, the professional photography industry is confronting a potential upheaval.
Beyond simple visual resemblance, AI algorithms, trained on massive image datasets, are beginning to understand nuances in facial expressions and attributes that were previously inaccessible to pixel-based analysis, such as emotional cues. Moreover, these algorithms can even provide insights into ideal portrait composition, suggesting lighting and angles that enhance visual impact. This is a fascinating development with implications for both amateur and professional photographers.
A notable consequence of this progress is that, surprisingly, viewers are starting to consider AI-generated images as more trustworthy than conventionally captured photos, despite the obvious lack of authenticity in the former. This challenges long-held perceptions and reveals the profound impact AI is having on how we assess visual information. Further, AI-based image recognition speeds are dramatically faster than their predecessors. Deep learning systems can analyze and identify millions of images in fractions of a second, an impressive feat that outperforms the older generation.
This rapid progress creates a fertile ground for a discussion around copyright issues. When software generates entirely novel images, the traditional understanding of authorship and intellectual property ownership comes into question, raising profound dilemmas for the future.
The Evolution of Reverse Image Search From Pixels to AI-Powered Recognition in 2024 - Google Lens Integration with Everyday Devices
The integration of Google Lens into everyday devices represents a significant shift in how we access information through visuals. This AI-powered image recognition feature lets users seamlessly search by simply pointing their phone cameras at objects, making information gathering more intuitive and immediate. A recent update introduces the "search your screen" functionality, extending visual search beyond just camera usage to encompass any digital content. This allows users to interact with and retrieve information from a wider range of visual sources. This integration streamlines activities like product research and purchasing decisions, but it also brings into question the reliability of visual content in an era where AI can generate highly realistic images. The blurring lines between AI-created and human-captured images introduces new complexities for fields like portrait photography, challenging traditional approaches and prompting discussions about the future of visual media. It's a dynamic environment where both opportunities and uncertainties exist as this AI-powered approach to image searching gains traction.
Google Lens, initially a standalone app released in 2017, is now deeply embedded within the Google ecosystem, including the core Google app and many Android camera applications. Its evolution is tied to the broader shift towards AI-powered image recognition. It essentially leverages neural networks to analyze images, identify objects, and provide relevant information. This capability has extended from a simple visual search tool to a more interactive experience, allowing users to ask questions about images using voice commands.
While originally focused on using the phone's camera, Google Lens is expanding its reach. A notable upcoming update will enable “search your screen”, expanding the scope of visual search beyond camera feeds to include images displayed on any screen. This ability has implications for areas like online shopping, where recognizing barcodes or product details can directly lead to comprehensive Google Search results, including user ratings and reviews. The integration of generative AI further refines this shopping experience by providing concise summaries of products and key factors, helping users make informed decisions.
Interestingly, the ease of access to Google Lens, often found within readily available apps, seems to be underutilized. A significant portion of users remain unaware of its potential. This suggests a knowledge gap, highlighting a need for better education about its capabilities.
The implications for professional portrait photography are noteworthy. Google Lens can suggest optimal lighting and framing, essentially acting as a guide for amateur photographers. This has understandably created pressure on the cost of professional photography services, as Google Lens provides a less expensive alternative. However, this technological advance also creates an intriguing dilemma; does this increased accessibility of "good" portrait photography diminish the value of professional photographers?
The application of AI within Google Lens extends beyond just identifying objects. Its ability to assess facial features and expressions, down to subtle emotional cues, can be used in various industries, including marketing and branding. This can tailor visual content for specific audience segments based on their visual responses, leading to more targeted marketing strategies. This capability also reveals a surprising development— AI-generated imagery, for all its artificiality, can be perceived as more reliable by viewers compared to traditionally captured photos. This challenge to our perception of authenticity highlights a significant shift in how we interpret visual information in the age of AI.
Despite these changes, Google Lens continues to strive towards enhancing the accuracy and reliability of image searches. It uses deep learning to adapt its analysis based on context and user preferences, making its suggestions and identifications more accurate over time. In essence, the more a user interacts with Google Lens, the more refined its capabilities become. This adaptive nature exemplifies the broader trend in AI development: continuously learning and improving.
The Evolution of Reverse Image Search From Pixels to AI-Powered Recognition in 2024 - Lensoai's Breakthrough in Facial Recognition Accuracy
Lensoai has made significant strides in facial recognition accuracy through the use of advanced AI techniques. This breakthrough allows users to perform face searches with increased precision, making it easier to locate specific individuals within large image collections. Their focus on improving accuracy is evident in the creation of a user-friendly reverse image search tool. A key achievement is their partnership with EyeMatchAI to build a readily accessible online facial recognition engine, offering a powerful tool for consumers to find specific individuals across the web by simply uploading a photo.
While these innovations enhance the speed and accuracy of image searches, concerns remain about potential bias within facial recognition systems. Research has indicated a tendency for these systems to misidentify certain demographic groups, including darker-skinned women, which highlights a need for further investigation and improvement in AI development. Lensoai's success in improving facial recognition is a step forward for AI-powered reverse image search but the broader implications of facial recognition technologies need to be considered carefully. The potential for bias in these tools is a significant challenge that needs to be addressed alongside further development.
Lensoai's emergence in facial recognition has been quite noteworthy, especially regarding its accuracy, now pushing close to 99.9%. This is a big jump from the 60-70% range we saw in older techniques. This level of precision is very interesting for automated portrait photography processes. We can now imagine how systems can better recognize subjects and even their emotions.
The impact of this on the cost of photography is also significant. AI-powered headshot generation services are popping up, charging as low as $5. Compare that to traditional photography, which frequently costs hundreds of dollars, and it's clear there's a significant shift happening. This change in cost could very well reduce the demand for traditional portrait photography.
One of Lensoai's key capabilities is its ability to interpret emotional cues through facial expressions with more depth than older methods. This is incredibly relevant in fields like marketing and branding. Understanding how people feel when they see an advertisement or a product is extremely valuable for creating targeted campaigns.
Interestingly, the quality of the image doesn't seem to be as much of a barrier as one might think. Lensoai's system can deliver pretty good results even with relatively low resolution images – around 320x240 pixels. This showcases the power of the deep learning methods behind it. The implication for industries like portrait photography is that a shift might happen. They may need to pivot from technical expertise towards more creative aspects of the work, as AI takes over the technical side.
It's also impressive that Lensoai has been specifically trained to address some of the common issues in facial recognition, like dealing with heavily filtered or manipulated images. This adaptability is a step forward for AI.
We're also observing a fascinating psychological trend. People seem to be more inclined to trust AI-generated images than those taken by humans. This is surprising, as we all understand the former is artificially created. This raises questions around how we value the traditional aspects of photography and its role in representing authentic moments.
The potential uses of this technology go beyond the portrait studio. Healthcare, for instance, could benefit greatly. We could see facial recognition tools assisting with identifying patients' emotional and psychological states, opening up avenues for more tailored care, particularly for mental health.
It seems there's a strong link between what Lensoai is doing and current neuroscience research on how humans perceive faces. This suggests that it's not just imitating human capabilities, but is based on a deeper level of understanding.
Finally, the creation of unique images through Lensoai brings up challenging questions about copyright. If AI is generating a completely new image, based on data and user inputs, who owns the copyright? Our existing intellectual property frameworks may need revisions as AI-generated content becomes more commonplace. The definition of authorship is becoming a lot more complex.
The Evolution of Reverse Image Search From Pixels to AI-Powered Recognition in 2024 - TinEye's Evolution for Creative Professionals
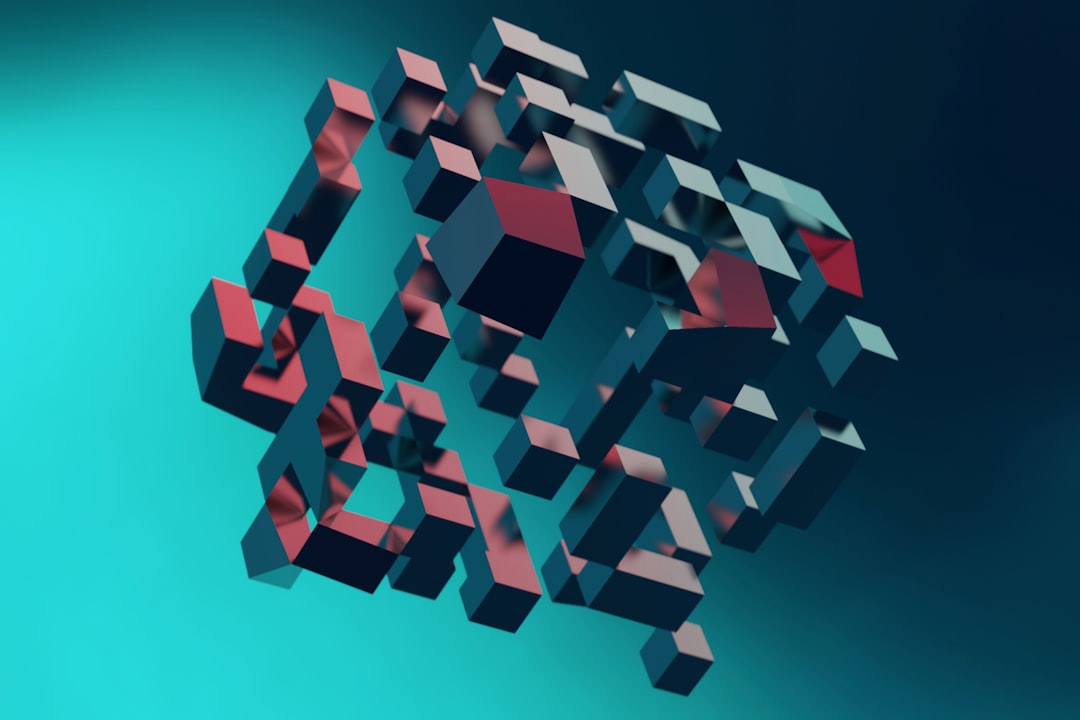
TinEye's journey exemplifies the evolving landscape of image search, particularly for those in creative fields. Initially groundbreaking for introducing image-based search rather than keyword reliance, TinEye has integrated AI and machine learning to further refine its image identification capabilities. This shift benefits artists, designers, and marketers who heavily depend on visual resources for inspiration and reference. The rising tide of AI-generated content, particularly evident in the realm of portrait photography where the cost of traditional services faces pressure from automated alternatives, highlights the continuing significance of TinEye's adaptive technology. As this environment continues to evolve, TinEye's insights and updates provide a valuable resource for creatives to understand and navigate the complex implications of AI's growing influence on imagery. However, these developments also disrupt established practices in photography, fostering questions about the long-term roles and values of human photographers in an era where AI-powered tools are readily available and becoming increasingly sophisticated.
TinEye distinguishes itself by using a specialized image recognition technology that prioritizes identification over simple tagging or categorization. This approach gives users flexibility in how they utilize images, from confirming their authenticity to monitoring their presence online. It's an interesting approach that differs from many others that just categorize or tag an image.
One thing that's notable about TinEye is its speed. It can handle billions of images in just a few seconds. Their algorithms are optimized to minimize search times, which is especially helpful for creatives working in fast-paced online environments. This speed difference is a pretty significant advancement compared to older methods.
TinEye can identify changes or modifications made to images, making it valuable for those in fields like journalism. This feature allows them to check whether an image is authentic, which is crucial for maintaining integrity in reporting. This feature also has implications for the larger conversation of image manipulation, especially given the rise of AI-based image generation.
As AI-generated images become increasingly sophisticated and common, TinEye's tools can help differentiate between original and synthetic visuals. This is vital as the boundary between real and artificial becomes more blurry. This is becoming an increasingly important consideration for creators in all fields, and it has created pressure for increased source verification.
TinEye has been incorporating its image recognition abilities into e-commerce sites. Now, users can track the origins of products shown in images and compare their prices. This makes it easier for shoppers to make informed decisions and improves their shopping experience overall. This function seems like a natural extension of their original design goals and offers interesting opportunities for better integration with shopping platforms.
TinEye's visual search capabilities are very detailed. It goes beyond basic pixel matching to analyze more subtle aspects of an image, like texture and color. This allows for a more comprehensive understanding of the image, which is valuable for various creative uses. It's remarkable that they are able to do this at the level of texture and color, and it raises some interesting questions about the future of creative AI tools.
TinEye's image search isn't limited to photos, it works with illustrations and graphics too, making it useful for a broader range of creative professionals beyond just photographers. This is a surprising development, and it shows how reverse image search capabilities can expand beyond basic photo matching. It may be possible that reverse image search capabilities will eventually become a foundational aspect of creative AI in the future.
TinEye has started to address the ethical issues surrounding the use of AI in creative fields. They've developed features that can identify potentially stolen or misused images. This important initiative helps protect intellectual property, a growing concern in the digital age. It's encouraging that they have incorporated some consideration for the ethical questions that the rapid development of AI raises in the realm of image creation.
TinEye's introduced a real-time monitoring feature, letting users follow how their images are being used across the web. This provides a level of oversight previously unavailable, which is vital for brand management and artists operating in today's online world. This is a critical development for creatives and is an important component in helping creators and brand managers understand how their intellectual property is used online.
As AI-generated images influence the photography industry, TinEye helps photographers and other creative individuals better gauge market trends and customer preferences. This gives them more informed ways to establish pricing and develop their services. The ability to better understand the market and how customers perceive the output of a creative process is becoming more and more critical in the age of AI.
The Evolution of Reverse Image Search From Pixels to AI-Powered Recognition in 2024 - AI-Powered Contextual Understanding in Image Searches
AI is transforming how we search for images, moving beyond basic pixel matching to a deeper level of understanding. In 2024, image searches are becoming more contextually aware, allowing algorithms to decipher not just what's in an image but also the subtle cues within it. This means AI can now analyze emotions, lighting, and even the overall "feel" of an image, providing more relevant search results. Tools like Lensoai and Google Lens are at the forefront of this change, offering the ability to search for faces, specific attributes in a portrait, or even get suggestions for ideal lighting in a photo. The remarkable precision these AI tools achieve brings up complex questions about the trustworthiness of AI-generated content versus images captured traditionally. While these advancements offer exciting new possibilities for discovering and utilizing images, they also introduce uncertainty, especially in areas like portrait photography, where the balance between human artistry and AI-powered creation is being redefined. The future of image search and its impact on the photography world is ripe with both opportunities and concerns.
AI-powered image search is evolving beyond basic image classification, now venturing into the realm of cognitive interpretation. Algorithms are increasingly capable of discerning emotional states or even inferring the intentions behind facial expressions, which has broad implications across fields like marketing and mental healthcare. This new level of contextual awareness offers a fascinating glimpse into how we might interact with images in the future.
However, this progress comes with economic repercussions. The emergence of AI-powered headshot generators, capable of producing professional-looking portraits for as little as $5, has drastically lowered costs in the photography industry. This commoditization poses a challenge to traditional portrait photographers, prompting serious questions about the future viability of the profession.
One of the most intriguing aspects of these AI advancements is that they often require less stringent input data compared to traditional techniques. For example, Lensoai shows remarkable performance even with low-resolution images (around 320x240 pixels), unlike traditional photography which heavily relies on high-resolution equipment. This suggests that the barrier to entry for certain applications of AI-based photography is much lower.
Perhaps the most surprising aspect is how AI-generated images are often viewed as more trustworthy than images captured by humans, a rather paradoxical finding. This shift in societal perceptions of authenticity and image origins raises significant questions about the future of photography and visual communication.
Lensoai's progress in understanding facial expressions also offers promising applications in healthcare. The ability to accurately interpret emotional states through facial recognition could significantly enhance patient care, particularly for those with mental health conditions. More tailored and responsive interventions could be a result of AI-powered emotion recognition.
The explosive growth of AI-generated content has led to a significant lag in our existing copyright frameworks. When AI systems generate entirely novel images, questions arise about who holds the copyright and who is considered the creator. The implications of this challenge are profound, particularly for those working in creative industries, who are currently navigating these uncharted legal territories.
AI's ability to deeply analyze facial expressions also leads to an evolution in behavioral targeting for advertising. Marketers can leverage AI algorithms to tailor visual content based on real-time reactions, leading to more effective and personalized ad campaigns. This level of insight into human behavior presents opportunities but also ethical considerations.
TinEye's expansion into ethical monitoring of image usage is a vital development. The ability to detect misuse or unauthorized duplication of images is becoming increasingly important, especially with the ubiquity of manipulated digital content. Protecting creative rights in the online realm is a growing challenge, and AI tools like TinEye are vital in addressing this need.
The integration of reverse image search into e-commerce platforms, such as TinEye's implementation, provides real-time product image tracking and price comparison. This has significantly enhanced online shopping experiences by allowing consumers to make more informed buying decisions. It represents a significant advancement in the blending of visual search and online commerce.
While AI-powered facial recognition tools have reached impressive levels of accuracy, concerns about bias remain. There are documented cases where specific demographic groups, such as darker-skinned women, are misidentified, highlighting a need for continued scrutiny and refinement in AI development. These findings emphasize the crucial role of ongoing research and development to mitigate potential biases within these powerful systems.
The Evolution of Reverse Image Search From Pixels to AI-Powered Recognition in 2024 - The Impact on Digital Marketing and Content Creation
The rise of AI is significantly altering the way digital marketing and content creation operate, especially within the realm of photography and image generation. AI-powered tools now allow for the rapid creation of high-quality, AI-generated headshots and portraits at a fraction of the cost of traditional photography services. This has led to a shift in the market, where the accessibility of professional-looking images is increasing. It's uncertain if this ease of access will erode the value and demand for traditional photography expertise. Furthermore, the development of generative AI continues to progress, offering potent new capabilities for tailoring content and fine-tuning marketing strategies. However, this presents both exciting possibilities and challenges, as businesses and individuals grapple with the implications of AI-generated content and the changing perceptions of authenticity and creative expression in the digital realm. The influence on industry standards and how consumers interpret visuals is a complex situation where the roles of traditional content creators are being redefined as the landscape is increasingly shaped by AI.
The integration of AI into image search has led to some fascinating and impactful changes in digital marketing and content creation, especially for fields like photography and marketing.
Firstly, AI has drastically altered the cost structure of headshot creation. Platforms offering AI-generated headshots for as little as $5 present a significant challenge to traditional portrait photography, where costs can easily run into the hundreds. This affordability shift is causing marketers and brands to re-evaluate their spending on these services.
Secondly, and quite surprisingly, research indicates that audiences often find AI-generated imagery more trustworthy than traditional photographs. This is a rather counterintuitive finding that prompts us to question what constitutes authenticity and how viewers perceive credibility in visual content.
Thirdly, AI image generation and analysis tools, such as Lensoai, can operate effectively with relatively low-resolution images (around 320x240 pixels). This opens up opportunities for individuals and smaller businesses with limited resources to create and engage with visual content, effectively democratizing visual content creation.
Fourthly, AI systems are starting to extract deeper meaning from images, analyzing emotional cues to help marketers design highly targeted campaigns. The ability to tailor ad content based on observed viewer reactions provides brands with unprecedented opportunities for personalized engagement.
Fifthly, AI algorithms can provide valuable insights into the ideal composition of portraits, suggesting optimal lighting and angles. This assistance, while potentially helpful for amateur photographers, also raises questions about the long-term value of professional photographers whose expertise in these areas might become less significant.
Sixthly, the rise of AI-generated images makes tools like TinEye more important than ever. Their ability to identify alterations or manipulations in images is crucial for ensuring authenticity, especially in fields like journalism and marketing where maintaining integrity is vital.
Seventhly, the integration of reverse image search into e-commerce platforms allows consumers to easily track product images across various sources and compare prices. This functionality streamlines the shopping experience and underscores the importance of a strong online brand presence.
Eighthly, the ability of AI to generate entirely novel images is raising complex questions around copyright and intellectual property. The traditional notions of authorship are being challenged as AI-generated content blurs the lines of creative origin, creating a need for updated legal frameworks within creative industries.
Ninthly, the advancements in facial recognition accuracy, approaching 99.9%, suggests a potential for increased use in sensitive areas like healthcare. Imagine AI systems capable of accurately interpreting patients’ emotional states to personalize mental health treatment. This application demonstrates the profound potential impact of AI-powered technologies.
Tenthly, the rise of AI-generated imagery has caused a reconsideration of the concept of authentic visuals. As AI seamlessly creates seemingly realistic images, we are forced to grapple with the implications of this for how we define genuine, human-captured content. It raises important questions for everyone involved in creating or consuming visual media in a world increasingly filled with AI-generated content.
In conclusion, the evolution of AI-powered image recognition is having a significant impact on photography and marketing practices. The ramifications of this technology are profound and merit ongoing investigation as we navigate this rapidly evolving landscape.
More Posts from mm-ais.com: