The Evolution of Cost Behavior Analysis in Managerial Accounting Trends and Techniques for 2025
The Evolution of Cost Behavior Analysis in Managerial Accounting Trends and Techniques for 2025 - Advanced Data Analytics in Cost Behavior Prediction
The field of cost behavior prediction is undergoing a significant shift, fueled by the integration of advanced data analytics. Techniques like machine learning and predictive modeling are increasingly being used within managerial accounting to dissect how costs change in response to different levels of business activity. This shift enables businesses to make more astute decisions in today's complex economic environment. By leveraging these capabilities, organizations can develop a much deeper understanding of how operational performance directly affects financial outcomes, potentially leading to greater efficiency. The ability to analyze vast quantities of data through big data analytics allows for a move away from the limitations of traditional cost analysis methods, paving the road to more accurate forecasting and innovative approaches to managing costs going forward. It's important to note, though, that as these sophisticated tools become more prominent, it’s crucial to critically examine their effectiveness and any limitations they may introduce in fully grasping the nuances of cost behavior. This careful approach is essential to ensuring the reliable application of these advanced technologies.
The field of cost behavior prediction is undergoing a significant shift, moving beyond relying solely on historical patterns. We're seeing a growing emphasis on real-time data streams, allowing businesses to adapt their cost projections on the fly. This dynamic approach offers a more agile response to changing business environments compared to the traditional reliance on past trends.
Machine learning algorithms are proving to be especially adept at unearthing intricate relationships within massive datasets. This capability makes them particularly well-suited to forecasting variable costs, which can exhibit complex fluctuations based on production levels. While traditional regression techniques still have their place, machine learning offers a promising route to enhanced prediction accuracy in these situations.
The ability to analyze unstructured data is another exciting development. Natural language processing tools can extract meaningful information from sources like maintenance records or customer reviews, uncovering hidden factors that influence costs. This is a crucial advantage since traditional quantitative methods may miss these qualitative aspects.
Big data and the Internet of Things (IoT) are merging in interesting ways within cost behavior analysis. Predictive analytics platforms can now integrate a wide range of data sources, including sensor data from factory floors. This allows for incredibly detailed insights into cost behavior tied directly to operational conditions.
It's interesting to note that, despite the immense potential of data, there's also a potential downside: 'data fatigue.' As organizations accumulate vast quantities of data, there's a risk of becoming overwhelmed, struggling to sift through the noise to extract useful knowledge. This underlines the need for carefully targeted analytical strategies.
In situations with limited historical data, techniques like synthetic data generation can prove useful. These methods can simulate different cost scenarios, offering valuable support for companies lacking extensive historical records. This capability is crucial in enabling modeling of future costs in dynamic or nascent industries.
Going beyond simply predicting cost changes, advanced analytics can also incorporate principles of behavioral economics. This means understanding how human decision-making biases might affect cost allocation and spending within organizations. This broader perspective can potentially lead to more realistic and nuanced predictions.
The rise of cloud computing has created opportunities for cross-departmental collaboration in analytics. This shift facilitates wider sharing of cost behavior analysis insights and integration into decision-making across the organization. This collaboration fosters a more unified and impactful approach to using these findings.
The implementation of advanced analytics in cost forecasting has shown significant promise in improving accuracy. There are instances where it has led to up to a 50% increase in forecast precision. This is particularly valuable in reducing the risk of over- or under-estimating project costs.
Finally, we're seeing a stronger focus on the importance of data visualization. Effectively communicating the relationships between cost drivers and operational performance is crucial for engaging stakeholders in meaningful strategic discussions. Clear, visual representations can help quickly convey complex information and promote a more informed understanding of cost behavior.
The Evolution of Cost Behavior Analysis in Managerial Accounting Trends and Techniques for 2025 - Integration of Machine Learning for Pattern Recognition
The integration of machine learning for pattern recognition is transforming how managerial accounting approaches cost behavior analysis. Machine learning's ability to unearth complex patterns hidden within large datasets allows for more precise predictions, particularly for variable costs that fluctuate with business activity. This not only improves the accuracy and speed of financial reporting but also offers timely insights that are critical for making quick decisions in today's fast-paced environment. The promise of automation through machine learning is appealing, but it's crucial to acknowledge potential drawbacks. Organizations must be vigilant in ensuring that the use of these powerful tools doesn't lead to a simplification of the complex forces that drive costs. Finding the right balance between automation's benefits and a deep understanding of fundamental economic principles is essential for maximizing the value of this technology in managerial accounting.
The integration of machine learning (ML) into cost behavior analysis is opening up new avenues for pattern recognition that traditional methods often miss. For example, ML can uncover non-linear relationships within data, which are vital for accurate cost behavior analysis but frequently overlooked by simpler regression techniques. This capability is particularly important when we're dealing with complex cost dynamics.
Furthermore, ML models can be tailored to specific industries, learning from the unique patterns of cost behavior that arise from factors like regulatory changes. This adaptability makes them more effective at forecasting across diverse environments, offering a more refined approach than general-purpose models.
One exciting development is the use of ensemble learning, where multiple algorithms work together to improve accuracy. This method has shown potential for significantly enhancing the robustness of cost projections by minimizing the problem of overfitting that can happen in simpler models. This raises questions about the best ways to design and deploy ML for robust cost analysis.
ML's capacity to process data in real-time is a significant advantage. It allows organizations to adapt cost estimates nearly instantaneously in response to sudden market shifts or supply chain disruptions. This dynamic capability isn't easily replicated using traditional techniques which can struggle to react to quick changes.
Integrating ML with current accounting software can automate repetitive tasks and enhance workflow efficiency. However, this automation comes with its own set of challenges. It raises concerns about potential errors if algorithms are not updated and audited regularly, creating the need to balance the benefits of automation against the need for human verification.
Intriguingly, some research suggests that ML-based cost behavior analysis often needs less historical data than traditional methods. This makes it a valuable tool for organizations in rapidly evolving sectors or ones with limited data. It seems a very useful attribute when considering certain industries.
While ML excels at recognizing patterns, not all algorithms are equally adept when working with financial data. The performance of these techniques can falter if the underlying assumptions about the data are flawed. This highlights the importance of human oversight and expertise in the implementation of ML models.
Anomaly detection is another area where ML is proving useful. It can identify unusual cost behavior and flag potential issues that require immediate attention. However, if these anomalies are indicative of larger problems within the organization, this emphasis on pattern recognition can sometimes lead to overlooking the fundamental causes. This implies the importance of human investigation in tandem with automated pattern recognition for a complete and well rounded perspective.
The evolution of ML in cost prediction has raised questions about model transparency. Many complex ML models act as "black boxes," making it hard for users to understand how they reach their conclusions. This lack of transparency can be problematic for accountability and decision-making, especially within a management context where explanations for decisions are crucial. Perhaps we need to focus on developing explainable AI for cost behavior analysis going forward.
Finally, adopting ML techniques successfully requires a significant cultural change within organizations. Employees need training and reskilling to fully leverage these tools, which can often be a major hurdle to implementation. Successfully integrating ML in a firm may require changes in organizational structure and practices, to support the new skills and expectations.
As we move toward 2025, the evolving landscape of cost behavior analysis is clearly integrating ML for pattern recognition. However, it is a field still needing careful exploration and a balanced approach, with its strengths and limitations being carefully considered.
The Evolution of Cost Behavior Analysis in Managerial Accounting Trends and Techniques for 2025 - Real-Time Cost Monitoring through IoT Devices
The integration of the Internet of Things (IoT) into cost behavior analysis is creating new avenues for real-time cost monitoring. This capability allows businesses to gain a more dynamic understanding of how costs change, moving beyond solely relying on historical data. IoT devices, like sensors and GPS trackers, provide a continuous flow of information on operational performance. This real-time data stream makes it possible to see the direct link between operational conditions and cost fluctuations, giving rise to potentially better, more adaptable cost forecasting methods.
The potential of using artificial intelligence alongside IoT for anomaly detection is also significant. This pairing can help organizations quickly spot unexpected changes in costs and operational processes, giving them a chance to intervene promptly. The possibility of identifying and preventing future cost issues could improve efficiency and reduce disruptions.
However, the use of real-time cost monitoring through IoT also presents some complexities. Managing and analyzing such large amounts of data can be challenging, and there's a risk of organizations becoming too reliant on automated systems, potentially losing sight of the nuanced causes of cost behavior. It's crucial that businesses critically evaluate how they integrate and utilize this technology to avoid simply substituting one set of potential issues for another. Ultimately, the effective use of IoT for cost monitoring will likely depend on a delicate balancing act between the potential for more accurate, dynamic cost analysis and a robust understanding of the underlying economic forces driving cost behavior.
The Internet of Things (IoT) offers a powerful new way to capture cost-related data across many operations in real-time, providing a level of detail that traditional accounting methods simply can't match. This detailed view allows businesses to directly link specific actions and situations to cost changes, uncovering relationships that were previously hidden.
IoT's impact on cost reduction has been shown in areas like manufacturing and shipping, where it's demonstrated potential savings of up to 20%. This ability to immediately address inefficiencies that might otherwise slip by until financial reports are generated is quite valuable.
It's important to remember that effectively using IoT for cost monitoring isn't just about technology. Businesses often underestimate the need to train their workforce on how to utilize these systems, resulting in the data not being put to its best strategic use.
While we might think that more data always leads to better decisions, IoT can actually lead to an overload of data. If organizations don't have advanced filtering and analytics capabilities in place, this flood of real-time information can make it harder to identify the most important insights.
Interestingly, IoT-based cost monitoring isn't only for large companies. Smaller businesses are finding ways to leverage this technology affordably. Tailored IoT solutions can deliver the type of insights previously available only to bigger firms with more resources.
IoT can also monitor external elements, such as market trends or customer feelings, which can have an impact on cost management. This broader view makes things more complex, but it also offers a rich source for forecasting cost behavior in the future.
The advantages of IoT come with certain risks. The interconnected nature of IoT devices opens them to security vulnerabilities, making companies susceptible to data breaches that could lead to financial loss and create issues in how costs are managed.
IoT's rapid data collection and processing speeds allow organizations to shift from reacting to costs to proactively managing them. Businesses can anticipate fluctuations instead of just responding to them, completely changing how they approach budgeting and forecasting.
Quite often, using IoT to monitor costs exposes previously unknown inefficiencies, such as unused assets or redundant processes. This type of insight can lead to strategic reallocations and significant cost savings.
While the benefits of using IoT for cost monitoring are clear, the process of implementing it can be challenging. It usually requires collaboration among various stakeholders and a substantial investment in both technology and people. Having a well-defined implementation plan is crucial for success.
The Evolution of Cost Behavior Analysis in Managerial Accounting Trends and Techniques for 2025 - Blockchain Technology for Enhanced Cost Transparency
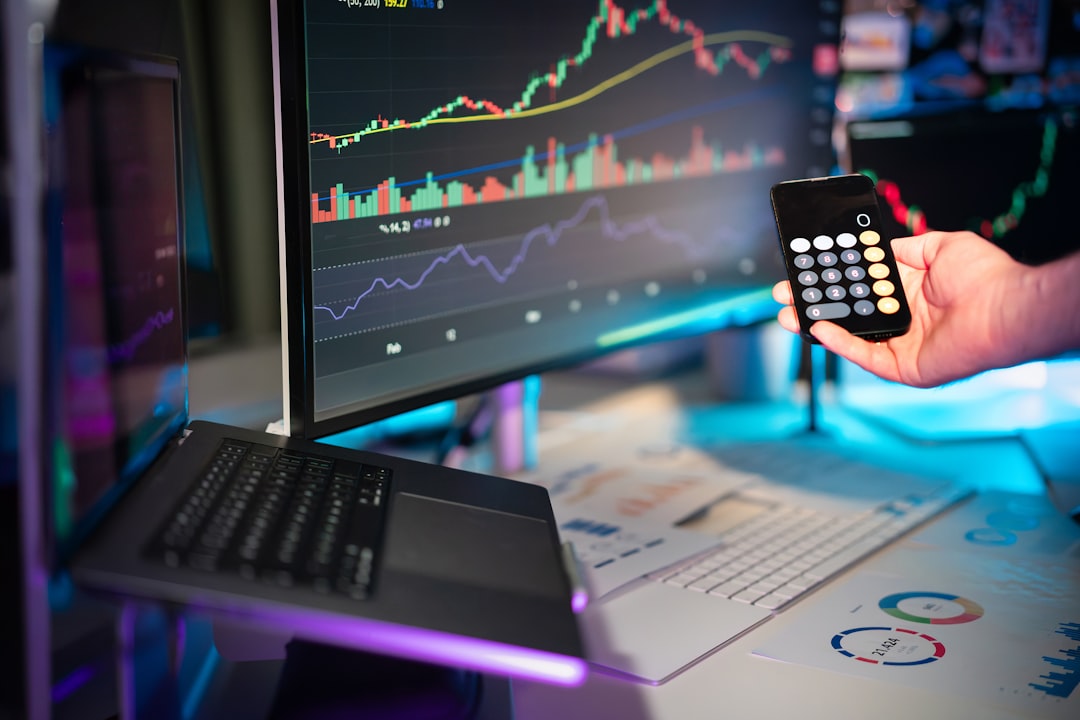
Blockchain technology offers a promising avenue for improving cost transparency in managerial accounting. By creating a shared, immutable record of transactions, blockchain can enhance the reliability and security of financial data used in cost analysis. This decentralized approach can streamline the auditing process and allow for real-time tracking of costs across various stages of the value chain, fostering better-informed decision-making.
Despite the potential benefits, widespread adoption of blockchain in cost management faces obstacles. Existing accounting systems and business models may need substantial adjustments to integrate blockchain seamlessly. Additionally, concerns about the complexity of implementing blockchain and the need for specialized skills may slow its broader acceptance. Nonetheless, the potential for blockchain to reshape cost transparency and elevate the trustworthiness of cost data in the future warrants continued exploration and discussion among accounting professionals as we approach 2025.
Blockchain technology is being explored as a potential game-changer for enhancing cost transparency in managerial accounting, particularly as we look towards 2025. It's fascinating how the inherent features of blockchain, like its decentralized nature and immutable ledger, could potentially revolutionize how we track and manage costs.
One of the intriguing aspects of blockchain is its ability to create an immutable record of every financial transaction related to costs. This 'write-once' characteristic means that once a cost entry is recorded, it cannot be altered, creating a highly reliable audit trail. This immutability, while seemingly simple, could have significant implications for accountability in financial reporting, possibly reducing the risk of manipulation or errors.
Blockchain's decentralized structure also stands out. Unlike traditional centralized databases, which rely on a single entity for managing data, blockchain spreads the responsibility across a network of computers. This distributed ledger concept makes the cost data more resistant to hacks and data corruption, ensuring that the information remains accessible even if one part of the network fails. The potential ramifications for internal controls and cost oversight could be profound, particularly as it could encourage more distributed monitoring of cost behavior across departments.
Smart contracts, another exciting aspect of blockchain, are essentially automated agreements written in code. When integrated with cost-related processes, they could automate tasks like expense allocation and payment processing. While this can streamline operations, it also raises questions about potential errors if the code itself is flawed or not properly maintained. There's a tension between the automation benefits and the need for robust error checks and regular reviews of smart contract implementations.
Furthermore, the decentralized nature of blockchain enables real-time visibility into cost allocation. This transparency could help businesses quickly identify areas of inefficiency, potentially leading to better cost management and improved decision-making. However, this transparency also raises questions about who has access to the cost data and how to manage the potential overload of information in certain business situations.
Blockchain's inherent transparency also presents the possibility of reducing the risk of fraudulent activities. Since every transaction is visible to all participants on the network, it becomes significantly harder to manipulate cost data for personal gain. This increased security could foster trust and integrity in cost management practices.
Another noteworthy aspect of blockchain is its ability to reduce transaction costs by minimizing or eliminating intermediaries. This streamlined process leads to faster settlement times and potentially reduces administrative overhead, which can translate into tangible savings for businesses.
Moreover, blockchain's chronological record of transactions provides enhanced traceability of cost-related activities. This granular level of detail is particularly helpful in regulated industries where detailed documentation of cost behavior is necessary.
The real-time reporting capabilities of blockchain are also enticing. Instead of waiting for periodic financial reports, businesses can access current cost data at any time. This immediacy is critical in today's fast-paced environments where timely information is essential for making rapid and informed decisions.
Interestingly, the integration of blockchain with the Internet of Things (IoT) has the potential to further enhance cost transparency. Imagine a scenario where IoT sensors automatically record cost-related data, which is then verified and stored on a blockchain. This synergy could create a system capable of capturing and analyzing a vast amount of operational data, opening possibilities for predictive cost analytics based on immediate conditions.
While still in its early stages, the prospect of global standardization in cost reporting through blockchain is also intriguing. As the technology matures, the potential to simplify cross-border transactions and enhance transparency in international financial dealings could benefit global enterprises, but it also requires consideration for potentially complex legal and regulatory hurdles.
Blockchain technology, as it relates to cost behavior analysis, offers a fascinating potential for a new generation of accounting practices. However, its adoption faces significant hurdles. Developing robust standards, addressing concerns about data security and access, and adapting current organizational practices are just a few of the obstacles that need to be navigated. The future of this technology in cost management is undoubtedly still evolving, and it will be exciting to observe how these challenges are addressed as we progress towards 2025 and beyond.
The Evolution of Cost Behavior Analysis in Managerial Accounting Trends and Techniques for 2025 - Sustainability Metrics in Cost Behavior Analysis
The growing recognition of sustainability's importance for long-term business success has brought sustainability metrics into sharper focus within cost behavior analysis. While a significant number of CEOs see sustainability as vital, many lack the tools to properly measure its impact on their businesses. Methods like full-cost accounting and models for sustainability assessments are being adopted to illuminate the social and environmental consequences of a company's actions. This evolution goes beyond simply meeting regulations, demanding a more in-depth comprehension of how sustainability efforts influence cost structures and influence management decisions. By integrating these metrics into the analysis of cost behavior, businesses can better align financial responsibility with ecological concerns, a trend likely to continue as we approach 2025. This is a crucial development, as it forces a reevaluation of how organizations consider their role in broader society. However, it's critical to be aware of the potential complexities and limitations of these new methodologies, particularly when applying them across industries and accounting systems.
A growing number of people are realizing that incorporating sustainability metrics into the way we look at how costs behave is crucial. These metrics help us understand how environmental and social factors, often grouped under ESG, can impact things like supply chain costs and how we price things. It's become clear that these non-financial aspects can have a substantial impact on the overall cost structure of a company.
It's also been interesting to see that using things like life cycle assessment (LCA) as a sustainability metric can show us that the upfront costs of using sustainable materials might not give us the complete picture of the long-term financial benefits. In some cases, using these materials can actually lower operational costs over time because they can improve efficiency.
A study done a few years ago showed that companies which carefully tracked sustainability metrics alongside traditional cost metrics saw a significant improvement in the accuracy of their cost forecasts. This suggests that by including a wider range of performance metrics, businesses can create much more reliable financial projections.
In industries like manufacturing, adopting practices aligned with a circular economy can lead to substantial reductions in the costs of raw materials. This shift in how costs behave is driven by sustainability metrics that encourage things like waste reduction and using resources more efficiently, which ultimately changes how we think about traditional cost structures.
It's quite interesting that when companies openly report their sustainability metrics, investors tend to feel more confident in them. This can lead to a lower cost of equity for those companies. This highlights how sustainability metrics can affect financing costs in a way that adds another layer of complexity to the traditional way we analyze cost behavior.
Businesses that use sustainability metrics are reporting that their employees are more engaged. This heightened engagement can lead to lower labor costs because there's less turnover. This illustrates how an organizational culture focused on sustainability can influence cost dynamics in a meaningful way.
It's been surprising to discover that including sustainability metrics can act as a sort of safeguard against supply chain disruptions. Businesses that carefully consider the sustainability of their suppliers have experienced less volatility in the costs of materials, which allows for more stable cost projections in environments with rapidly changing markets.
The use of predictive algorithms paired with sustainability metrics has shown real promise in industries that require a lot of energy. We've seen that by optimizing operations to meet sustainability goals, these companies have been able to reduce their costs.
Interestingly, as of today, businesses that have broadly adopted sustainability metrics have seen an improvement in their profit margins. This is a direct result of things like waste reduction and using energy more effectively. This really highlights the financial benefits that can come from committing to sustainable practices.
Lastly, it's worth noting that companies that regularly audit their sustainability metrics during their cost analysis are better able to identify operational inefficiencies. These inefficiencies can then translate into significant annual savings. By focusing on these audits, organizations are able to continually improve their cost management strategies by aligning operations with sustainability goals.
The Evolution of Cost Behavior Analysis in Managerial Accounting Trends and Techniques for 2025 - Cognitive Computing for Complex Cost Structures
Cognitive computing offers a new way to understand and manage complex cost structures within managerial accounting. It blends cognitive science, data science, and computing to analyze how costs respond to different operational conditions, including factors like cost stickiness. By mimicking human thinking and learning, cognitive computing enables a deeper understanding of cost behavior, moving beyond traditional approaches. This includes integrating concepts like cognitive and physical time into Activity-Based Costing (ABC). Importantly, cognitive computing also enhances real-time analysis of data, leading to improved decision-making and resource allocation. The ability to rapidly adapt to the ever-changing business environment becomes increasingly vital as we move towards 2025, and cognitive computing appears well-suited to address these challenges. While the field of cognitive computing in cost behavior analysis is still evolving, its potential to enhance cost management decision-making makes it worth exploring for businesses looking to improve their agility and competitiveness.
Cognitive computing offers a novel approach to understanding intricate cost structures by recognizing the complex relationships between various cost elements that traditional models often miss. For instance, it can uncover real-time connections between fixed and variable costs in dynamic business settings. These systems are designed with flexible algorithms that can adapt to new data and adjust predictions as business conditions evolve. This flexibility provides a far more responsive way to manage costs than the static models relying only on historical data. It's interesting to consider how cognitive computing can also draw insights from the field of behavioral economics. By incorporating behavioral biases into cost analysis, it potentially leads to a more accurate understanding of how subjective factors might skew cost allocation decisions, promoting more objective planning.
Additionally, these systems can process qualitative information through natural language processing. This means extracting valuable insights from sources like employee feedback or market sentiment, uncovering subtle drivers of cost behavior that quantitative analysis might miss. Unlike traditional approaches, cognitive computing doesn't limit itself to structured data. It effectively utilizes a wide range of unstructured information—think emails, chats, or social media trends—to glean valuable insights that impact cost management decisions. These systems allow for real-time scenario simulations. Rather than sticking to linear projections, businesses can simulate potential cost outcomes across different operational and market scenarios. This feature is especially useful in dynamic environments. Cognitive algorithms are also remarkably good at detecting anomalies in cost patterns. This capacity can identify inefficiencies or potential risks much earlier than traditional auditing approaches, leading to earlier intervention.
These technologies foster collaboration across different departments by providing shared insights on cost behavior. This capability helps break down data silos and promotes a more collective sense of responsibility for the organization's financial health. The data-driven nature of cognitive computing enables a proactive approach to cost management. Instead of simply reacting to cost overruns, organizations can use cognitive systems to implement preventative measures well before financial reporting cycles. It is worth mentioning that these systems are designed to continually learn and improve their accuracy over time. They refine their understanding of cost behavior as they process new data. This capability is a significant advantage over traditional systems that often require extensive retraining or adjustments for each new dataset. As the field evolves, it will be interesting to see if cognitive computing truly lives up to its potential for improving cost analysis and informing strategic financial decisions in the years to come.
More Posts from :