Marketo University Unveils 7 New Interactive Learning Paths for Marketing Automation in 2024
Marketo University Unveils 7 New Interactive Learning Paths for Marketing Automation in 2024 - Campaign Workflows Path Brings Drag and Drop Interface for Advanced Email Sequences
Marketo University's new Campaign Workflows Path is designed to make building elaborate email sequences easier. It offers a drag-and-drop interface, which is supposed to make designing and managing complex workflows more approachable. This approach, which relies on Smart Campaigns and a robust automation system, allows marketers to implement custom actions and dynamic triggers. While this is meant to improve user experience, particularly for marketers hoping to streamline their operations, it remains to be seen if it truly simplifies campaign management for everyone. The push for more intuitive tools is part of a broader trend in marketing automation that emphasizes ease of use. Whether it translates into a real gain in efficiency for the average marketer is yet to be determined.
One of the new learning paths focuses on campaign workflows and introduces a drag-and-drop interface specifically designed for building sophisticated email sequences. It seems like the idea is to make creating these complex email journeys more intuitive. This approach is supposedly rooted in some cognitive theory about reducing mental strain, which in turn helps people learn and perform better.
It's claimed that drag-and-drop interfaces generally speed up tasks, maybe by about 30% compared to the traditional click-around methods. If this claim holds up, we could see faster campaign deployments and quicker testing cycles, which would be useful for getting email strategies off the ground.
There's also this predictive analytics feature built-in. It apparently suggests the best email sequence based on what's been seen in previous interactions with users. While interesting, it raises questions about how robust the machine learning algorithms behind it are and if they can truly optimize engagement.
The new workflow also allows for A/B testing directly within the sequence. This, in turn, allows real-time adjustments based on what recipients do. That could be useful since studies suggest that tailoring content leads to better email open rates.
It also looks like the workflow tool supports multi-channel marketing. That's in line with the trend of recognizing the importance of consistent messaging across channels. Whether it genuinely helps with customer retention and engagement is still to be seen.
Additionally, the interface offers customizable templates, which are good for quickly making copies of successful campaigns. This potentially saves a lot of time. It's interesting to see how well the effectiveness of the templates aligns with that 20% effectiveness gain.
In terms of team collaboration, it looks like they designed it so that multiple people can work on the workflows at the same time. This follows the idea that knowledge and creativity come from working together. However, the real-world practicality and implications of having many people working on the same workflow need to be assessed.
There's also a stated emphasis on accessibility. It seems that it's designed so that everyone can use the drag-and-drop functions, regardless of ability. That's a good step and falls under the design principle of making products for all people.
The tool includes analytics that provide immediate feedback on each workflow, enabling changes based on what's seen. This kind of data-driven approach is important in marketing. The claim of a 5-10% success rate increase is interesting but needs more verification.
The final aspect discussed is automation within the workflows. This enables automated sequences based on events, which leads to efficiency and better response time. The 14.5% improvement in ROI claim from automation is worth exploring, however it is difficult to know in what context the ROI was measured.
Marketo University Unveils 7 New Interactive Learning Paths for Marketing Automation in 2024 - Data Management Learning Track Shows Real Time Database Syncing Methods
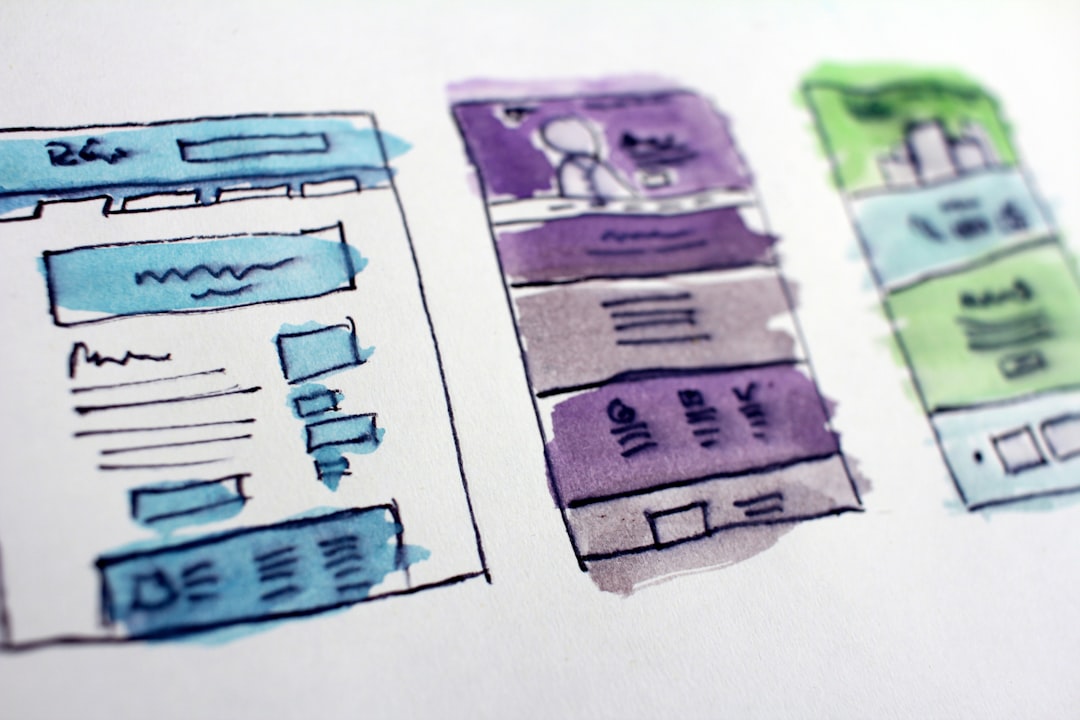
One of the new learning tracks from Marketo University focuses on data management, specifically how to keep databases synchronized in real time. This is important because marketing data, especially customer interactions, changes frequently. The track covers methods for keeping data synced across different systems, such as Marketo and data warehouses. It explores ways to set up a reliable flow of information, potentially using things like Kafka clusters, which is a technology that can handle lots of data quickly. The challenge, as the track likely emphasizes, is that managing the flow of data becomes more complex and difficult as the amount of data increases.
This track aims to provide the skills needed to make sure that Marketo and other systems, like CRMs, have the same information about customers and prospects. The idea behind this is that having all of the data in the same place leads to better marketing campaigns and possibly a better experience for customers. However, there is a trade-off: the more data there is and the more systems that need to be kept in sync, the more difficult it is to maintain the data management system.
A big part of the track likely examines how bidirectional syncing can be useful, particularly in the context of marketing and sales alignment. By having Marketo and other systems like Microsoft Dynamics CRM share data in both directions, it allows for a constant exchange of lead and contact information. The curriculum likely also emphasizes the importance of regular maintenance because these syncing processes, especially as they become more complex, need to be reviewed and updated periodically. It's likely that the track discusses how to anticipate and respond to problems in syncing.
One of the new learning paths within Marketo University focuses on how to manage data in real time. It dives into how to keep databases synchronized in real-time, which is essential for ensuring that marketers are using the freshest data when making decisions. This is particularly important in marketing, where a few minutes of delay can affect how you target and interact with prospects.
However, there are potential issues with real-time syncing, such as the delay it can experience as it moves data between servers. During peak times, this can cause a backlog in processing and make data appear out of date. Depending on how the database is designed, real-time syncing can mean that data is only eventually consistent or, in some cases, very reliably consistent. It's important to understand what consistency model is being used when designing these systems.
Real-time syncing also creates some challenges. For example, if two different programs try to update the same data point at the same time, you run into what's known as a database conflict. There needs to be a clear way to resolve these kinds of conflicts to avoid corruption. Another challenge is keeping this syncing process running well as data volumes grow. As more data is generated and synced, the entire process can get bogged down and require considerable computing power. This can be costly and make the system more sensitive to failures.
If you were to switch over to a real-time data management system, you might have to completely overhaul the underlying database architecture. Relational databases are often not the most efficient when handling very large volumes of constantly changing data. Newer databases designed for dynamic data and large datasets might be more suitable. Furthermore, connecting existing software to a new real-time data system can be difficult. You might need to custom-build code (API integrations) that translates the data from the older system to the newer one, requiring both marketing and engineering expertise.
Of course, having access to the most current data enables some powerful analytical techniques. For example, real-time analytics tools can give you instant insights that are critical for making fast decisions. But marketers and analysts have to adjust to this rapid influx of data and the tools required to make sense of it. Too much data can overwhelm marketers and potentially even annoy users if the constant updates are not personalized or relevant.
One final concern to consider with real-time data syncing is security. Because data is moving constantly between different systems, security holes can expose it to more risks. Security policies need to be well-defined to minimize the risk of breaches as data streams move between various points. In conclusion, while real-time data syncing is a useful tool in the marketer's arsenal, it's crucial to acknowledge these challenges in designing a robust system.
Marketo University Unveils 7 New Interactive Learning Paths for Marketing Automation in 2024 - Lead Scoring Path Introduces Machine Learning Based Contact Rating System
Among the new learning paths offered by Marketo University, the Lead Scoring Path stands out by introducing a machine learning-driven contact rating system. This represents a departure from traditional lead scoring methods that relied on manually assigning points based on actions like form submissions or email opens. Instead, this new approach utilizes predictive analytics to analyze a contact's behavior and purchasing history, creating a more dynamic and potentially accurate rating. This shift aims to improve the quality of leads passed to the sales team by better identifying those most likely to convert.
However, relying on machine learning introduces complexities. The effectiveness of this new rating system hinges on the sophistication and accuracy of the algorithms that power it. Marketing teams have to consider whether the benefit of a more nuanced lead score outweighs the potential increased difficulty of managing yet another layer of automated systems within their existing marketing workflows. There's a risk of over-reliance on a system that may not always be accurate, and the need to balance the power of automation with the ability to understand and fine-tune its outcomes remains critical. The real impact of this machine learning integration on improving lead scoring will depend on how well it tackles these inherent challenges.
One of the new learning paths focuses on lead scoring and introduces a machine learning-based system for rating contacts. This system uses algorithms that constantly adapt based on how people interact, potentially allowing for real-time improvements in how leads are ranked. This dynamic approach could lead to better ways of prioritizing leads and optimizing marketing efforts.
It's interesting that using machine learning models for lead scoring often leads to better predictions. Research suggests they can boost accuracy by as much as 30%. However, how well it works depends heavily on the quality and amount of data used to train the models. This means there's a limit to what these models can learn if the training data isn't representative or extensive enough.
One interesting feature is that this system can find patterns in how users behave. This can reveal insights into engagement that traditional scoring methods might miss. It could even help find hidden segments within the lead database that weren't apparent before. This aspect of the system makes me curious about how it could help uncover previously unknown groups of users.
However, the use of machine learning in lead scoring can have some drawbacks. These systems can sometimes pick up biases from historical data, potentially leading to unfair preferences or prioritizing of certain types of leads over others. This is something researchers and developers need to be aware of and adjust for so that the system doesn't inadvertently perpetuate existing inequalities.
The new rating system is a big deal because it shows a shift toward making marketing decisions based on data. It's likely that organizations that use these methods will see a better return on their investments, with some reports claiming an increase of up to 15% in overall campaign success. However, this claim is likely based on specific cases, and broader results remain to be seen.
There's potential for gains in speed and efficiency as well. Machine learning algorithms can sift through enormous datasets much faster than traditional methods, possibly cutting lead scoring time from hours to minutes. This accelerated scoring process could be a game changer, especially for marketing plans that need quick execution. However, the extent of the speed gain depends on the complexity of the datasets being processed and the underlying hardware and infrastructure.
The technical side of machine learning for lead scoring relies heavily on complex approaches, like neural networks or decision trees. Marketers need a solid understanding of these models to be able to use the results correctly and make good choices based on the information generated. This means it's important to understand how the system reaches conclusions and not just accept the results blindly.
It's also crucial to remember that while machine learning can offer more flexible and responsive lead scoring, marketers still need to stay involved in the process. Relying too much on automated systems without oversight could lead to poorly informed choices based on flawed data interpretations. A balanced approach of humans and machines would be beneficial to help identify and eliminate potential errors in the model.
This new contact rating system might also provide valuable insights across various marketing channels. Since machine learning models gather data from multiple platforms, they could potentially find important trends and allow marketers to optimize strategies based on the observed patterns. However, ensuring seamless integration between channels is essential for fully maximizing the insights gained.
Lastly, this system might be able to anticipate future lead behavior, such as the likelihood of a purchase or continuing engagement with marketing efforts. That would enable marketers to develop custom marketing strategies. This level of personalization could dramatically change how customer journeys are designed. However, it's also crucial to maintain rigorous standards for data governance and privacy to ensure that this personalized marketing doesn't become intrusive or overly invasive. It will be interesting to see how privacy standards evolve along with the increasing sophistication of machine learning based marketing.
Marketo University Unveils 7 New Interactive Learning Paths for Marketing Automation in 2024 - Analytics Dashboard Course Adds Mobile Performance Tracking Module
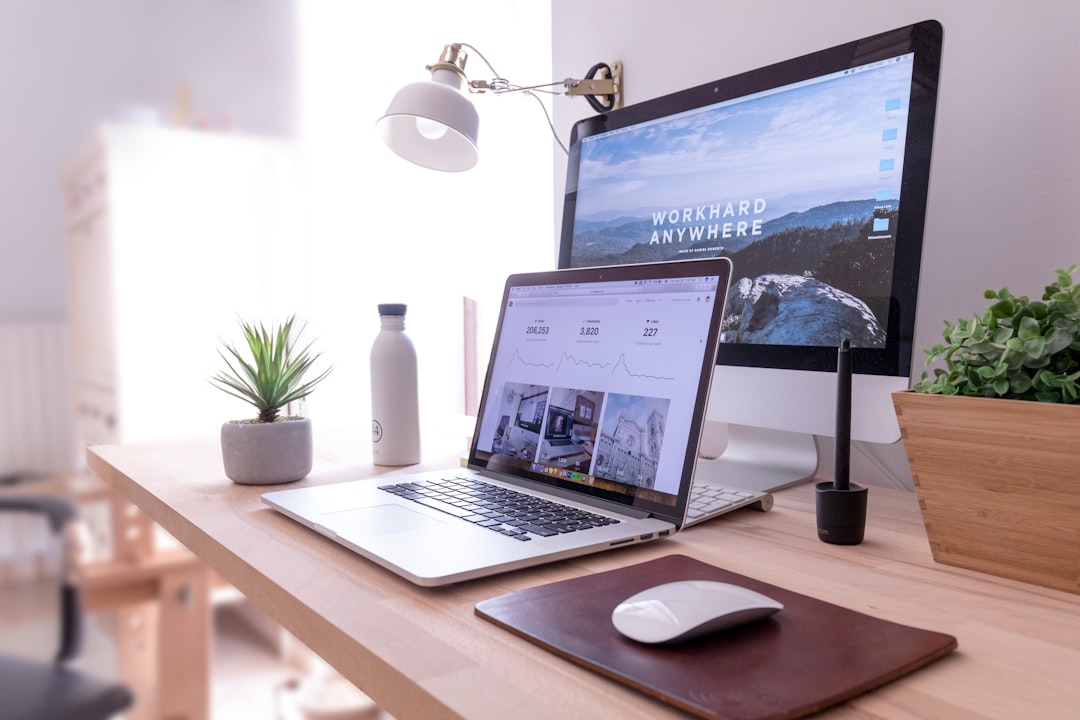
Marketo University's Analytics Dashboard Course has gotten a new module focused on tracking how marketing campaigns perform on mobile devices. This addition signals a growing emphasis on understanding how people interact with marketing materials on their phones and tablets. The module is supposed to give users a clearer picture of how well their mobile campaigns are doing, offering a more holistic view of marketing effectiveness across all devices. However, this is just one part of a larger puzzle. There are a lot of different aspects to mobile analytics that need to be accounted for in today's world. Given the importance businesses place on mobile-first strategies, it's not clear if this new feature adequately handles all the intricacies of mobile analytics in a complex marketing landscape. It's also unclear if the insights gained are comprehensive or easily interpreted. While the inclusion of a module dedicated to mobile is a step in the right direction, its effectiveness will rely on how well marketers use the information provided to truly understand and improve their strategies across all channels.
The Analytics Dashboard Course has been updated with a new module focused on mobile performance tracking. This is a significant addition, especially given the increasing reliance on mobile devices for internet access. We're likely to see mobile traffic make up a larger portion of overall web traffic in the coming years, so understanding how campaigns perform on mobile is becoming increasingly important. The idea behind the module is to give marketers real-time visibility into how users are engaging with content on their phones and tablets. This shift towards mobile-centric analytics is in line with the wider trend of users expecting more personalized and context-aware marketing experiences.
This new module, by offering mobile-specific analytics, potentially enables more effective campaign optimization. There's research suggesting that mobile users react more positively to timely, context-specific marketing, potentially leading to a significant improvement in engagement rates. However, it's important to acknowledge that mobile browsing sessions are generally shorter compared to desktop sessions, creating a need for rapid analysis and the ability to adapt strategies quickly.
The module seems to be built around a foundation of mobile analytics, giving marketers a better understanding of the user's behaviour on mobile devices. This means we're talking about granular information on the specifics of how people are interacting with content on their phones, helping identify which specific features or actions within campaigns have the highest impact. This type of information might be particularly relevant when considering mobile app features like push notifications and in-app messaging, both of which have been shown to be effective at boosting engagement.
It's also interesting to think about how this module could be used to gather insights that might not be apparent from desktop analytics alone. For instance, mobile users tend to be more diverse in their demographics and often exhibit unique behaviour compared to desktop users. The ability to better understand these mobile user characteristics can likely be very useful for marketers tailoring campaigns for various segments.
Of course, with the growing use of mobile and the increased collection of data, questions about privacy inevitably arise. Regulations like GDPR and CCPA underscore the need for a robust approach to data governance. It will be important to see if the module's approach adequately accounts for these growing concerns regarding data privacy.
There's also potential for A/B testing within the mobile context. This capability could allow for rapid evaluation of different versions of campaigns, leading to faster iteration and possibly a noticeable improvement in performance. One potential area of interest is how machine learning could be integrated into the module. The ability to identify high-value customer segments based on mobile behavior would be valuable. Similarly, location-based marketing, which has shown promise for driving foot traffic, can now potentially be assessed within the context of mobile tracking.
One potential challenge with the module is the need to reconcile inconsistencies across different mobile operating systems. iOS and Android platforms have differences in their tracking mechanisms, potentially creating discrepancies in data that marketers need to account for to make effective decisions. This is a hurdle that's likely to be encountered more broadly in marketing moving forward as mobile environments continue to evolve. Overall, this new module represents a valuable addition to the course for marketers seeking to better understand and refine their mobile-focused strategies, but it remains to be seen how widely it is adopted and how effective it is for the average marketer.
Marketo University Unveils 7 New Interactive Learning Paths for Marketing Automation in 2024 - Landing Page Design Path Features New A/B Testing Framework
Marketo University's new Landing Page Design Path introduces a built-in A/B testing framework, aiming to help marketers improve their landing pages. It's surprising that, based on current data, only about half of marketers currently use A/B testing for their landing pages, hinting at a large untapped potential for boosting conversions. This new framework allows for direct comparison of different landing page versions within Marketo, making the testing process more convenient and supporting data-driven decisions. The path emphasizes the importance of optimizing elements like headlines and calls to action, suggesting that improvements in these areas can have a significant impact on campaign performance. The success of this new framework hinges on marketers' embrace of the new tools and their commitment to using them effectively to refine their landing page designs. There's always a risk that users don't fully grasp the significance of these tools, potentially impacting the expected improvements.
Marketo University's new Landing Page Design Path incorporates a fresh A/B testing framework, aiming to streamline the process of optimizing landing pages. This framework supposedly makes it easier to test different versions of landing pages at the same time, which could lead to faster decisions. The promise of real-time analytics through integrated tools allows marketers to shift from a post-campaign review to a more proactive approach. This could improve adjustments to campaigns based on visitor actions and, theoretically, lead to higher conversion rates.
However, there are limitations to consider. Studies show that effective use of A/B testing on landing pages can increase conversion rates by as much as 300%, but not all tests deliver clear results. It's crucial to consider the statistical significance of the results to avoid misinterpreting the data and making inaccurate conclusions.
Interestingly, this framework isn't limited to basic text or image tests. It enables marketers to explore various layouts and calls to action as well, which provides a more comprehensive view of what grabs a user's attention. Another noteworthy aspect is the automated segmentation of user traffic based on visitor behavior and demographics. This might enable better personalization and, in theory, more targeted messaging which could improve conversion and engagement.
The learning curve for marketers who haven't used A/B testing before might be lessened because of the framework's user-friendly features and best practice suggestions. While this is a positive, there's also a chance that marketers might favor the ease of use over the depth of the analytics, potentially overlooking some important information.
This tool also provides comparisons with historical performance data, which is helpful for understanding the current landing page design's success relative to past efforts. While insightful, there's a risk of falling back on past success rather than exploring novel options.
There's also the option of using machine learning algorithms that could predict which design elements work best based on past visitor interactions. However, we should question how effectively these algorithms can adjust to the ever-changing market landscape.
Another feature is its ability to connect landing page performance to other campaign metrics, offering a broader perspective across all channels. While this gives a holistic view, it introduces difficulties in assigning specific credit to each element of a campaign.
Finally, the ability to test changes on live campaigns is notable. However, if the landing page is in a constant state of change, it might negatively impact the consistency of user experience. Overall, this new path within Marketo University offers some promising features for landing page optimization. But as with any new tool, it's necessary to critically examine both the advantages and drawbacks to understand its full potential impact.
Marketo University Unveils 7 New Interactive Learning Paths for Marketing Automation in 2024 - Integration Architecture Track Maps Third Party Connection Points
Marketo University's new "Integration Architecture Track Maps Third Party Connection Points" learning path focuses on how Marketo Engage connects with other marketing technology (martech) tools. It emphasizes the value of pre-built connections, allowing marketers to link Marketo to a range of existing or future platforms. This approach, in theory, makes it easier to keep data flowing smoothly between different systems.
However, this integration process isn't always straightforward. The path likely delves into the limitations of the Marketo API, particularly the restrictions on how many users and third-party integrations it can handle at once. If you exceed these limits, it can slow down the API calls. This means that carefully planning and monitoring these integrations is important, especially when a large number of third-party apps are involved.
The goal of this learning path is to equip marketers with the understanding and skills needed to take advantage of these connections without encountering issues. While it highlights the benefits of having a unified view of marketing data and processes, it also makes marketers aware of the potential downsides. As the complexity of integrations grows, so does the risk of running into difficulties, which ultimately impacts the effectiveness of the entire system. Essentially, managing all of these connections efficiently can be a tricky balancing act. It is unclear if this new learning path fully addresses all of the complex technical and logistical details, or if it will merely scratch the surface.
Marketo Engage, being a part of the Adobe Experience Cloud, is designed to integrate with a wide array of existing marketing and other business tools. This integration capability is a core feature, but it also adds a layer of complexity to the system. Integration architecture track maps are essentially visual representations of how these connections work. They are important because they help us understand how various third-party tools interact with Marketo and its core database. Even minor changes to one part of the system can have complex, cascading effects on other parts.
The track maps go beyond just mapping connections; they also shed light on the flow of data between systems. Understanding how information is exchanged between third-party apps and the Marketo system is essential for optimizing the speed and performance of the data transfer processes. This is particularly important in marketing, where quick data updates can greatly affect how you interact with potential customers. The data transfer process can become more complicated if you are relying on something like a Kafka cluster to handle massive quantities of data.
A key aspect of these integration maps is the inclusion of strategies for handling potential errors. It's important to have ways of stopping problems in their tracks before they cause a major malfunction. That's because if one integration fails, it can cause problems for other parts of the system that depend on it. The architecture tries to prevent this by adding safeguards, ensuring the system remains stable.
The more modern integration architectures seem to be focused on handling updates in real-time. This makes sure that Marketo has the newest data for making marketing decisions, rather than having to deal with a slight delay or lag. This real-time aspect is valuable, as even small delays can sometimes mean the difference between reaching someone at the right time or missing an opportunity. But it also introduces complexities. If, for example, two parts of the system try to update the same data at the same time, you might get a database conflict and that's problematic.
The way the integration architecture is designed can affect how easily you can update or improve the system. If it uses a modular approach, it becomes much easier to make changes to one part of the system without disrupting the rest of it. This is useful because it means you can incorporate new tech faster, improving how the system works over time.
Security is a growing concern in all aspects of computing. With the increasing reliance on third-party integrations, it's more important than ever to implement good security protocols at all points where the Marketo system interacts with external systems. This is important because breaches of data often happen at the point where different systems connect.
The more connections you add, the more complex the architecture becomes. One concern when designing a system like this is whether it can handle the increased demands as the number of integrations grows. Understanding the limits of the system under pressure is important so that you can predict performance and make adjustments as needed.
Testing is critical in software development and that's especially true in this context. The system needs to be thoroughly tested to make sure that all the integrations work as intended and don't interfere with each other. This may sound straightforward, but in practice, it can be complex and time consuming.
A lot of the value from having these track maps is that they give you a better understanding of the impact on the user experience. Slow or problematic integrations can make things difficult for people and make the marketing campaigns less effective. This ultimately impacts sales, conversions and overall marketing success.
In many of these complex architectures, you'll find features designed to gather information about how the integrations are being used. These feedback loops can help improve the system over time. This continual improvement is key to keeping a complex system running efficiently. This includes the continuous monitoring of the system to ensure the integrations are optimized for performance.
These architecture maps, while complex, are useful tools for designing and managing the interconnectedness of marketing automation systems and third-party apps. They provide a visual and conceptual structure, allowing marketers and developers to anticipate and manage the inevitable complexities of such sophisticated systems.
Marketo University Unveils 7 New Interactive Learning Paths for Marketing Automation in 2024 - API Development Path Explores Custom Application Building Tools
Marketo University's new API Development Path aims to teach marketers how to create custom applications using Marketo's application programming interfaces (APIs). This path emphasizes the vital role of APIs in marketing automation, detailing how they work with languages like Python and Java through the REST API. It also covers important aspects like securing data access, defining permissions, and building helpful documentation with tools like Swagger. While the idea is to help users develop solutions through API integrations, it's important to remember that setting these things up can be tricky. Marketers should ideally balance leveraging automation with a clear grasp of how these APIs work without over-depending on them. They need to manage the integration process carefully. It remains to be seen if this learning path properly covers the complexities of integrating custom applications into Marketo, but it is a step towards a more custom approach to marketing automation.
Marketo University's new API Development Path is an interesting addition to their learning resources, offering a glimpse into building custom applications that integrate with their platform. It appears to be focused on leveraging Marketo's REST API, which allows for interaction with the platform using various programming languages such as Python and Java. While it seems intuitive on the surface, it raises several questions and introduces potential complications.
One of the key things to consider is that Marketo's API has limitations. There are restrictions on things like how many calls you can make per hour, which can become an issue if you are building an app that's going to see heavy usage. Managing the performance of these custom apps in high-traffic situations is going to be key. It's interesting to consider the rising prominence of no-code and low-code development platforms within marketing. The idea is to allow people with little coding experience to create more complex marketing workflows. Whether this approach is ultimately beneficial or results in hastily built apps with security vulnerabilities is yet to be seen.
The API Development Path likely touches on issues related to version control, as custom applications often involve interacting with multiple versions of the API. Keeping track of these versions can become tricky since changes in one API version might break compatibility with existing parts of the system. This can cause unexpected headaches if not properly managed. The path also likely emphasizes the importance of schema management because the structure of data needs to be consistent between systems, otherwise the entire app is going to run into issues. It appears a good amount of development time is wasted due to bad data mapping.
There's also the matter of security. Building custom applications can open up the door to new vulnerabilities, especially if the APIs themselves aren't built in a secure way. Protecting sensitive data through strong authentication and authorization practices is critical. Custom app development also emphasizes the ability to fetch data from Marketo in real-time, which is essential for responsive marketing applications. However, real-time updates can introduce complexities, potentially creating performance bottlenecks if not handled properly.
The curriculum probably stresses the significance of user experience. Focusing on user feedback throughout the development process is vital to ensure that the applications people build are usable and actually get used. It's likely that the learning path emphasizes things like usability testing and design principles. It appears that automated testing is also becoming increasingly important in this area, where development tools can automatically flag potential bugs earlier in the development process.
A significant challenge likely emphasized is data mapping between the Marketo platform and the custom applications. Developers have to ensure that data is accurately exchanged between systems, otherwise the information won't be helpful for making marketing decisions. Scalability is also likely discussed. Developers have to consider how these applications are going to perform as the number of users grows, ensuring that they can handle the increased demand. This kind of scalability planning isn't always done in the rush to get an application released.
In conclusion, while Marketo University's API Development Path appears to be a helpful step toward creating innovative marketing applications, it's essential to understand the complexities and potential challenges. Building truly useful and secure custom apps for marketing requires thoughtful consideration of the technical details, along with a focus on user experience and maintainability. The extent to which it succeeds in conveying these subtleties will likely determine its ultimate value to marketers.
More Posts from :