Job Order Cost Accounting in 2024 Adapting to AI-Driven Manufacturing Processes
Job Order Cost Accounting in 2024 Adapting to AI-Driven Manufacturing Processes - Real-time cost tracking with AI-enhanced sensors in 2024
In 2024, AI-powered sensors are fundamentally changing how we track costs in real-time, particularly within job order cost accounting. This shift allows companies to gain immediate insights into their spending, empowering them to make faster and more informed decisions. The ability to connect devices and sensors through the Internet of Things (IoT) is crucial in providing this granular data, offering a stark contrast to the less precise estimations used in traditional methods. The move towards relying on AI and machine learning isn't just a trend; it's becoming a necessity for businesses aiming to adapt quickly to changing market conditions. The level of detail provided on costs and profitability is now critical for competitiveness. While this automation improves the accuracy of financial reports and frees up accountants for higher-level tasks, it's important to acknowledge that the increasing reliance on AI might necessitate a careful consideration of human expertise and judgment within financial processes. There's a risk of overlooking the nuances that only human experience can bring to accounting, despite the many benefits of AI.
By 2024, we're seeing a surge in the use of AI-enhanced sensors for real-time cost tracking, pushing the accuracy bar beyond 99%. This means manufacturers can quickly adjust processes on the fly as variables shift during production. It's fascinating how these sensors can pick up even the smallest changes in temperature or pressure, which are directly linked to material costs. This allows engineers to make dynamic adjustments to their processes with unprecedented precision.
These advanced AI algorithms aren't just collecting data – they're actively analyzing it to find cost-saving opportunities. They can uncover inefficiencies that traditional methods simply miss, potentially leading to significant financial gains. Preliminary data suggests that real-time cost tracking with AI can cut production waste by as much as 30%. This is achieved by issuing alerts when there's overconsumption or deviations from normal operating ranges, enabling prompt corrective actions.
Interestingly, many companies implementing these AI systems are also reporting drops in labor costs. This is because automated monitoring lessens the need for constant human oversight, freeing up employees to focus on more complex tasks. It's becoming increasingly common for AI sensors to generate predictive analytics, anticipating future costs based on current operations. This advanced capability provides firms with a real edge when it comes to budgeting and project planning.
Furthermore, these systems are often surprisingly easy to incorporate into existing infrastructure. They can directly communicate with existing Enterprise Resource Planning (ERP) systems, meaning implementation can be smoother and less disruptive. In fact, these systems often enhance the overall functionality of the ERP system. It's worth noting that the reliance on AI sensors for cost tracking seems to be fostering a more decentralized approach to operations. Real-time data equips local teams with the ability to make immediate adjustments.
Some forward-thinking companies are even combining these sensors with blockchain technology. This experiment aims to increase transparency in cost reporting and ensure process accuracy, building confidence and trust throughout the supply chain. While manufacturing is at the forefront of this trend, it's exciting to see its applications expanding to other areas like logistics and construction. There, real-time data can significantly improve efficiency and accuracy in resource allocation. It will be fascinating to see how these trends further evolve.
Job Order Cost Accounting in 2024 Adapting to AI-Driven Manufacturing Processes - Predictive maintenance impact on job costing calculations
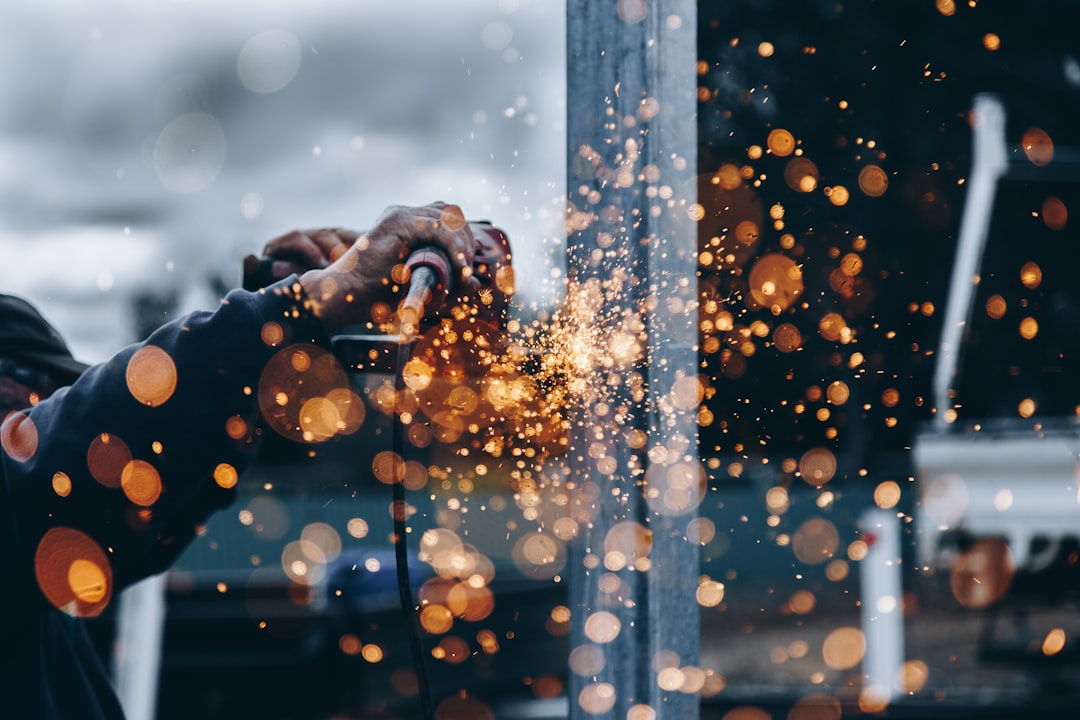
In the context of job order cost accounting, particularly as it adapts to AI-driven manufacturing processes, predictive maintenance is gaining importance in shaping how job costs are calculated. Using historical data and sophisticated analytics, predictive maintenance helps reduce unexpected production stoppages, ultimately impacting overall production costs and improving cost control. This integration allows for the automated generation of maintenance requests, leading to streamlined operations and ensuring maintenance is only performed when truly needed. As predictive maintenance becomes more commonplace in manufacturing, its insights are increasingly valuable in refining how job costs are calculated, providing a more informed approach to resource allocation and evaluating project profitability. Although this technology promises improved accuracy, it does introduce new considerations, particularly the delicate balance needed between automated processes and the essential human element in financial management.
Predictive maintenance can change how we allocate labor costs in job costing. Since we might need fewer technicians for routine inspections, we could potentially move people to more complex tasks that require specialized skills. This reallocation of people could impact the cost calculations in a project.
Historically, equipment failures have caused big swings in job costing estimates. Predictive maintenance tools can help lessen the frequency and severity of these issues. This stability could make budgeting and forecasting more reliable for projects.
Using predictive maintenance, we can gather data on how equipment performs over its lifespan. This allows us to make future job costing estimates based on real data rather than just past projects. We can refine our understanding of cost over time with this approach.
A surprising benefit of predictive maintenance is that it can extend equipment lifespans. This translates to lower spending on new machines, which could significantly affect the job order costs, particularly in capital-intensive projects.
The real-time data from predictive maintenance systems supports a more agile approach to cost management. When unexpected maintenance issues come up, project managers can quickly adjust the budget. This helps prevent cost overruns, which are always a risk.
Companies that use predictive maintenance report a decrease in unplanned downtime, anywhere from 20-40%. This has a direct effect on job costing because we get a more accurate picture of how much labor and equipment we're using.
We can use predictive analytics to anticipate not only when equipment will break down but also when material costs might change. This information is crucial when refining cost estimates for a project and for making overall financial decisions.
Using predictive maintenance often leads to better relationships with suppliers. Because timely maintenance reduces delays and inconsistencies, there's a sense of trust that can lead to more favorable pricing and terms for job costing.
It's interesting to see that organizations using predictive maintenance are developing a culture of proactive management. Teams are more involved in performance monitoring and continuous improvement, directly influencing the quality and costs of individual jobs.
It's easy to focus on the technological aspects of predictive maintenance, but we also need to consider the emotional and psychological impacts. When employees see real benefits from reduced breakdowns and more predictable workloads, it's likely that morale improves. This could translate into higher productivity and reduced costs on individual projects.
Job Order Cost Accounting in 2024 Adapting to AI-Driven Manufacturing Processes - Machine learning algorithms refine material usage estimates
Within the evolving realm of job order cost accounting, machine learning algorithms are becoming crucial for refining how we estimate material usage. These algorithms leverage the massive amounts of data collected in modern, connected factories, creating insights that make cost estimates more precise. They provide real-time views of factors that impact production, which lets manufacturers adjust their processes quickly to minimize material waste. This granular approach to material usage not only improves budget management but also enhances decision-making when it comes to allocating resources. In essence, it changes how manufacturing project costs are determined. While the advantages are clear, implementing these AI-driven tools does bring up questions about finding the right balance between automated processes and the important role human judgment plays in financial matters.
Machine learning algorithms are becoming increasingly integrated into manufacturing, particularly within the context of Industry 4.0, to improve how we predict material usage. They can analyze massive datasets, including data from smart sensors, to refine our understanding of material consumption, going beyond relying solely on historical data or manual estimations. This ability to analyze large datasets in real-time is particularly useful as it allows the algorithms to adapt to changing production conditions and refine their estimates accordingly. For example, algorithms can learn and adapt to shifts in production methods or material specifications. Interestingly, the use of ensemble learning—where multiple machine learning models work together—can not only improve the accuracy of the predictions but also reveal previously hidden sources of material waste.
In a surprising development, these algorithms have the potential to uncover seasonal or cyclical patterns within material consumption that might be missed by even the most experienced professionals. This offers a window into inventory management and production planning, mitigating risks of both overproduction and stock shortages. Research suggests that combining machine learning with historical job order costing data can significantly reduce the variability of material cost estimations. Especially for complex projects, this refined approach can enhance project cost predictions. Furthermore, these algorithms are showing the capacity to predict material price fluctuations, based on an analysis of supply chain variables, allowing businesses to optimize their material purchasing strategies. This predictive element allows companies to buy at advantageous times, leading to better cost control.
The ability to simulate various manufacturing scenarios is another powerful tool offered by machine learning. It empowers engineers to test 'what-if' scenarios with relative ease, allowing for optimized material usage before a project goes into full production. This 'what-if' capability is incredibly valuable, minimizing time wasted on trial and error and directly reducing potential material waste. In some instances, these algorithms have identified predicted material needs that differ significantly from earlier estimates, often driven by evolving project scope or design changes. This ability to flag these inconsistencies is crucial for timely adjustments, preventing cost overruns. Studies suggest that utilizing machine learning algorithms for material estimations has resulted in a considerable reduction in excess inventory. This is a valuable outcome as excess inventory represents tied-up capital that could be utilized for other purposes.
Perhaps more importantly, the implementation of machine learning algorithms has the potential to shift the overall culture of cost management within organizations. As the accuracy and visibility of material usage data increase, teams are prompted to embrace a more analytical and data-driven approach to their work. This shift in perspective and approach can translate to measurable improvements in overall operational performance. While this technology holds significant promise, it's important to remember that human expertise and judgment are still needed to ensure that the technology remains a supportive tool rather than a replacement for human oversight and insight.
Job Order Cost Accounting in 2024 Adapting to AI-Driven Manufacturing Processes - AI-driven labor allocation shifts traditional cost structures
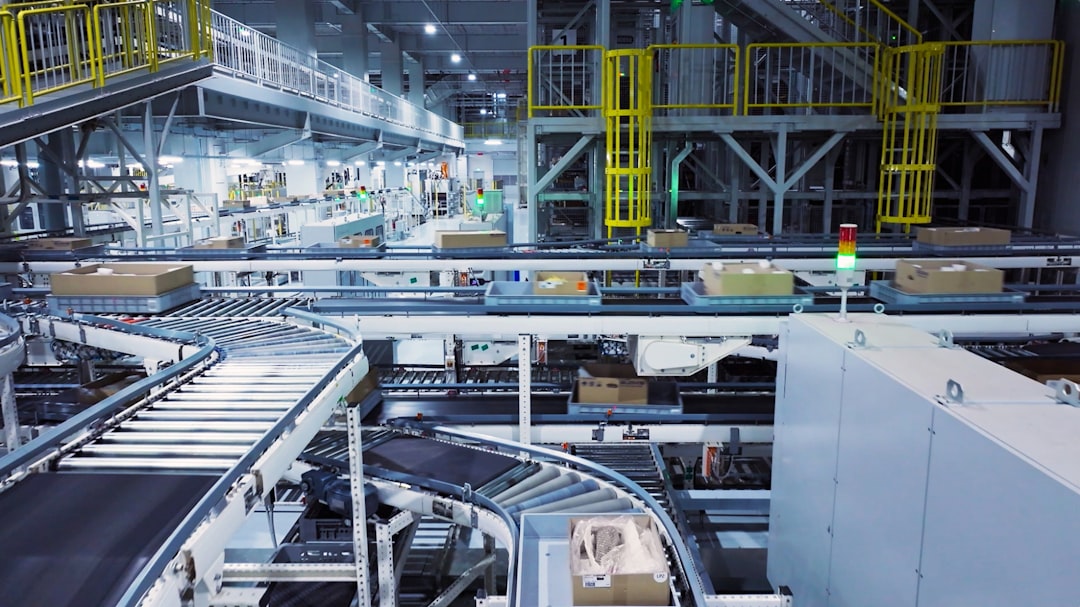
AI's growing role in labor allocation is significantly altering the way manufacturing costs are structured. The expanding use of automation in various tasks is changing the workforce landscape, which, in turn, influences traditional budgeting methods. AI's ability to optimize labor allocation improves efficiency, but also forces companies to re-examine long-held cost assumptions, particularly those related to labor-intensive parts of operations. While this promises cost reductions and improved cost management precision, it also sparks questions about the future of work and the skills humans need in more automated settings. The tension between the efficiency gains offered by technology and the necessity of human expertise is central to navigating the changes AI brings to job order cost accounting. It is clear that companies will need to adapt, and how they manage that shift will likely shape the future of manufacturing cost management.
The adoption of AI is fundamentally changing how labor is allocated, leading to noticeable shifts in traditional cost structures. In some areas, we're seeing reductions in labor costs, sometimes as high as 20%, largely because AI-powered systems are automating routine tasks and decreasing the need for constant human oversight. This frees up skilled workers to tackle more complex problems that still require human expertise.
Beyond simply optimizing for current projects, these AI systems are showing remarkable potential in forecasting future labor needs. They use predictive analytics linked to production schedules and market trends, which gives businesses the ability to react more quickly to changing demand. This proactive approach to staffing could be particularly beneficial in industries with unpredictable order volumes.
It's interesting to observe that many companies utilizing AI-driven labor allocation are reporting faster project completion times, sometimes up to 25% faster. This improvement seems to be linked to the systems' ability to more effectively match worker skills with project requirements compared to older methods. It's as if the AI has a deeper understanding of where each person's talents best fit.
Along with quicker project completion, we are seeing a rise in a more refined skill-matching process. AI-powered systems seem to be better at pairing individuals with jobs based on their specific competencies. This tailored approach isn't just improving productivity, but it seems to be boosting job satisfaction as well.
This whole process is having an unexpected impact on employee retention. Companies using AI for labor allocation are seeing a decrease in employee turnover. The primary reason appears to be that a better fit between employee and project is fostering a more positive work experience, particularly in markets with high competition for skilled workers.
AI systems have the capability to develop dynamic real-time labor dashboards. These dashboards track worker productivity and availability, allowing managers to instantly adjust staffing to meet project needs and ensure the workforce is used as efficiently as possible. The speed and flexibility of this approach are a significant upgrade from earlier systems.
The shift to AI-driven labor allocation is changing how businesses approach employee training. We're seeing a greater focus on developing skills where human expertise remains vital. This strategic investment in specific training helps ensure a smooth transition as some tasks are automated and others require highly skilled humans.
It's intriguing that the use of AI for labor allocation can contribute to a more even distribution of workloads. By taking into account an employee's individual capacities and project needs, AI can reduce the chance of burnout from overloaded assignments. Finding that balance between work and personal capacity might be one of AI's less-heralded strengths in workforce management.
One of the more practical benefits of AI-driven labor allocation is the enhanced ability of organizations to adapt to rapid project changes. Teams can reallocate labor within a matter of hours, where it might have taken days in the past. This ability to rapidly respond to shifts in project needs allows for improved agility and responsiveness to customer demands.
Finally, the trend of using AI in labor allocation seems to be promoting a more decentralized approach to management, empowering local teams to control their own labor needs in real-time. This kind of increased local control and adaptability could be a significant shift in organizational structure in the years ahead. While there are many challenges with introducing AI into any existing process, the impact on labor allocation is just starting to become visible.
Job Order Cost Accounting in 2024 Adapting to AI-Driven Manufacturing Processes - Blockchain integration ensures transparent cost data across supply chains
Blockchain integration is reshaping how cost data is handled across supply chains, introducing a transparent and verifiable record of transactions. By using a decentralized digital ledger, everyone involved in the chain has access to the same cost information, which builds trust and collaboration. This transparency also improves the tracking and management of products, lowering the risk of counterfeit items entering the market. Furthermore, this system helps reduce reliance on paperwork, which in turn decreases human errors and increases overall reliability in financial reporting.
Despite the promise of blockchain, there are still hurdles to overcome. Concerns around who has access to the data and the need for universally accepted protocols can create hesitancy for businesses considering adoption. However, as companies increasingly embrace AI and automated processes, blockchain technology might play a vital role in making operations more efficient and ensuring accountability throughout the supply chain.
Blockchain integration within supply chains offers a potentially powerful tool for creating a shared, transparent view of cost data. By utilizing a decentralized ledger, it's possible to build a single, immutable record of all cost-related transactions across the entire supply chain. This shared view can help eliminate the discrepancies that often arise from the various accounting systems used by different entities. It seems that blockchain’s ability to generate an audit trail for every transaction helps build trust among partners because they have a way to verify the authenticity of cost information, which has historically been susceptible to errors and even manipulations.
Interestingly, blockchain's potential goes beyond simple record-keeping. Some experts believe that it can also automate compliance checks related to cost reporting through the use of smart contracts. Essentially, smart contracts can automatically trigger predefined actions when certain cost-related thresholds are met or violated. While this level of automation might seem daunting, it could reduce human error in the reporting process and perhaps create efficiencies. It's worth pondering whether this level of automation would truly increase efficiency, or if it might simply shift the bottleneck to managing the complex rules embedded within smart contracts.
The decentralized nature of blockchain presents another advantage: even if a specific part of the supply chain encounters disruptions, the rest of the network can still access the necessary cost data. This could be beneficial for maintaining continuity during audits or contract negotiations. In theory, this could minimize the impact of supply chain disruptions and perhaps even help manufacturers create more resilient supply chains.
Blockchain can bring real-time visibility into cost changes, offering a substantial improvement over traditional systems that often operate on a delayed basis. This near-instantaneous access to cost information could be particularly helpful when dealing with unexpected cost fluctuations. This capability also presents interesting opportunities for organizations to utilize dynamic pricing strategies and even refine their risk management approaches, however, it remains to be seen how widespread its adoption becomes and how resilient it is in the face of rapidly shifting market forces.
Moreover, by connecting different companies on a shared network, blockchain might lead to opportunities for performance benchmarking. In this scenario, companies could compare their cost data against their peers. While this might sound appealing, it also brings up questions about the potential for information sharing or unintended consequences related to market dynamics.
Blockchain also offers a potential path towards speeding up the resolution of cost disputes between supply chain partners. Each transaction is recorded on a tamper-proof ledger, making it easy for parties to settle disputes quickly. This increased speed could streamline operations and lead to more efficient workflows. However, there is an open question regarding the actual practical adoption of blockchain in real-world situations and the legal challenges that could arise from utilizing it in this way.
From a sourcing perspective, blockchain could offer a powerful tool for verifying the authenticity of cost claims from suppliers. It could provide a means for tracing the origin of materials and ensure suppliers are not providing misleading pricing information. It remains to be seen if this approach would be widely adopted, as there are existing concerns around privacy and confidentiality in revealing such granular supplier data.
While enhancing transparency, blockchain might also increase the intensity of competition among suppliers. With the ability to see the actual costs associated with various products, buyers might negotiate better pricing. It's likely that this competition would be a driver of innovation and potentially improve the overall efficiency of supply chains, but it's crucial to consider how those changes might affect individual suppliers in the long term.
Interestingly, blockchain can facilitate a new level of collaboration among supply chain partners. With a shared understanding of cost data, they can potentially find new opportunities to optimize processes, pinpoint bottlenecks, and even collaborate on new joint ventures. It is exciting to consider the potential for such partnerships and it could create a new era of collaborative management within supply chains, however, there are risks related to information security and trust among partners that need to be carefully addressed.
In conclusion, blockchain's capabilities for cost transparency are changing the way companies approach cost management. By providing access to real-time data, it's likely that organizations can move away from reactive management to a more proactive approach, where they anticipate and adjust to future cost trends. While it presents exciting possibilities, it remains to be seen how successfully blockchain can be integrated into various sectors and overcome the existing challenges related to implementation and data privacy.
Job Order Cost Accounting in 2024 Adapting to AI-Driven Manufacturing Processes - Quantum computing accelerates complex cost simulations for manufacturing
Quantum computing offers a new avenue for accelerating complex cost simulations in manufacturing. Its unique capabilities allow for the exploration of intricate optimization problems and the execution of simulations much faster than traditional methods. This is becoming increasingly important as manufacturing embraces AI and seeks to improve its operational efficiency and refine business models. The substantial investments pouring into quantum computing technology highlight a belief in its potential to revolutionize various facets of manufacturing, including supply chain management and the development of new, AI-enhanced systems. While still in its early stages, the synergy between quantum computing and AI presents exciting prospects for disrupting existing cost accounting methods within the manufacturing industry. It’s plausible that this combination could usher in a new era of sophisticated and accurate cost modeling and planning. However, it is important to note the limitations of quantum computing currently and to critically assess the claims regarding its practical applications in cost simulation, as it may take time before it's widespread and ready for prime-time use.
Quantum computing is starting to show promise in speeding up complex cost simulations for manufacturing, a development that's definitely worth paying attention to for those of us interested in this field. Let's consider some interesting aspects of how this technology might influence manufacturing costs:
First, quantum computers use something called superposition, which means they can explore many different possibilities at once. This unique ability makes them really good at evaluating a wide range of cost scenarios and constraints simultaneously, significantly outperforming traditional computers for complex simulations.
Second, tasks that would take days or even weeks to complete on a regular computer might take only a few seconds using quantum algorithms. For manufacturing, this potential for speed could result in much quicker cost analyses and more agile operational decisions. Being able to respond quickly to market shifts could be a big advantage.
Third, figuring out how to minimize material costs can be a real challenge. Quantum computing might be able to optimize material use by solving incredibly complex problems involving factors like supply chains and inventory management, and doing it all in real-time. This could result in less waste and a more efficient use of resources.
One particular quantum computing method, called quantum annealing, seems particularly well-suited for finding the lowest cost solutions in a manufacturing process. Companies are exploring how to use quantum annealers to discover the best way to allocate resources, which is something that's often very difficult to simulate effectively using conventional methods.
Another aspect worth considering is the development of more advanced predictive models. By incorporating massive datasets with complex relationships into their calculations, quantum computing can lead to more accurate cost predictions. This improved forecasting ability could be very useful for budgeting and planning production.
It's also interesting to think about the combined power of quantum computing and machine learning. If we can combine these two technologies, we might develop groundbreaking algorithms that are much faster and more accurate in analyzing complex data. This could potentially improve cost estimations and trend analysis related to job order costing.
Furthermore, quantum computing can improve the simulation of product development research and development phases. This better simulation can lead to more precise budgeting, helping reduce uncertainty around the costs of labor and materials during innovation.
Quantum computers can also create intricate scenario analyses, including how costs might fluctuate due to economic factors. This kind of modeling could enable manufacturers to develop robust strategies to deal with potential problems that are simply impossible to simulate with conventional methods.
It's important to consider the impact on resource allocation. Because quantum computing is really good at solving complex allocation problems, companies might be able to make more informed decisions about how they use their workforce and machines. This will directly affect labor costs and overall efficiency.
Lastly, quantum computers might also give us a better ability to create long-term cost projections. By considering things like inflation, market trends, and operational inefficiencies, they can build more effective models of future costs, which can significantly impact job costing over time.
These aspects illustrate the exciting advances and transformative potential of quantum computing for improving cost simulations in manufacturing. It will be interesting to see how traditional cost accounting models adapt as these technologies continue to evolve.
More Posts from :