Emerging Trends in AI-Driven Inventory Management Software for Manufacturing in 2024
Emerging Trends in AI-Driven Inventory Management Software for Manufacturing in 2024 - Predictive Analytics for Demand Forecasting in Manufacturing
Predictive analytics is transforming how manufacturers forecast demand, utilizing vast datasets to gain deeper insights into consumer choices and market fluctuations. AI-powered algorithms are at the forefront of these advancements, allowing manufacturers to fine-tune their forecasting processes and adapt more readily to shifting demand patterns. A key technique within predictive analytics is time series forecasting, which analyzes past data to anticipate future market movements, thereby contributing to better inventory management. The ability to accurately anticipate demand is particularly crucial in today's environment where supply chain interruptions are a frequent occurrence. This capability empowers businesses to work more effectively with their suppliers and to navigate market changes more efficiently, ultimately contributing to smoother operations. While promising, relying solely on predictive analytics demands awareness of their potential drawbacks, including biases embedded in the data and inherent limitations in forecasting accuracy. A balanced approach is vital, recognizing the value of these technologies while acknowledging their imperfections.
Predictive analytics, through the application of sophisticated algorithms, can significantly boost the accuracy of demand forecasts in manufacturing, potentially achieving a 50% improvement over traditional methods. This increased precision allows companies to become more agile in adapting to shifting market conditions and helps prevent excessive stock build-up.
Integrating real-time data streams, like those provided by IoT sensors in advanced manufacturing setups, allows predictive models to evolve dynamically. This responsiveness is crucial as shop floor conditions change, resulting in more accurate forecasting.
The power of advanced algorithms lies in their ability to identify intricate patterns within vast datasets – patterns that human analysts might miss. This enhanced insight translates to a more proactive approach when making decisions about resource allocation.
Relying solely on historical sales data for predictive analytics may not always provide an accurate picture of future demand. Including external factors – like economic indicators or social media trends – has demonstrably improved forecast accuracy. It seems the world outside the factory gates matters.
Machine learning models applied to demand forecasting have shown remarkable potential in reducing stockouts by 20% or more. This impact on inventory levels directly translates to a better customer experience – higher customer satisfaction from always having needed products, which can subsequently fuel sales growth.
Machine learning's incorporation within predictive analytics offers a more nuanced understanding of seasonal demand fluctuations. This allows manufacturers to refine their production schedules, aligning them perfectly with anticipated peaks in demand – like holidays.
Traditional demand forecasting, if over-relied upon, can trigger a problematic effect known as the "bullwhip effect," where minor demand variations at the retail level cascade into major fluctuations up the supply chain. Predictive analytics offers a useful tool in mitigating these disruptive waves.
Predictive analytics provides manufacturers with a more granular approach to managing inventory by utilizing customer segmentation data. By tailoring inventory levels to different demographic groups, manufacturers can optimize their stock based on the unique buying habits found across various regions or customer types.
However, deploying predictive analytics in demand forecasting is not without its challenges. Data quality remains a significant hurdle. Manufacturers need to prioritize accurate and consistent data to prevent skewed predictions and disruptions to inventory levels. This emphasis on data integrity is critical for the success of the endeavor.
Maintaining the effectiveness of predictive analytics requires constant refinement. These tools can become outdated if not regularly updated with new data and analytical approaches. Consequently, manufacturers must view investments in analytics as ongoing, acknowledging that continuous improvement is necessary for sustained success.
Emerging Trends in AI-Driven Inventory Management Software for Manufacturing in 2024 - Real-Time Inventory Tracking with IoT Integration
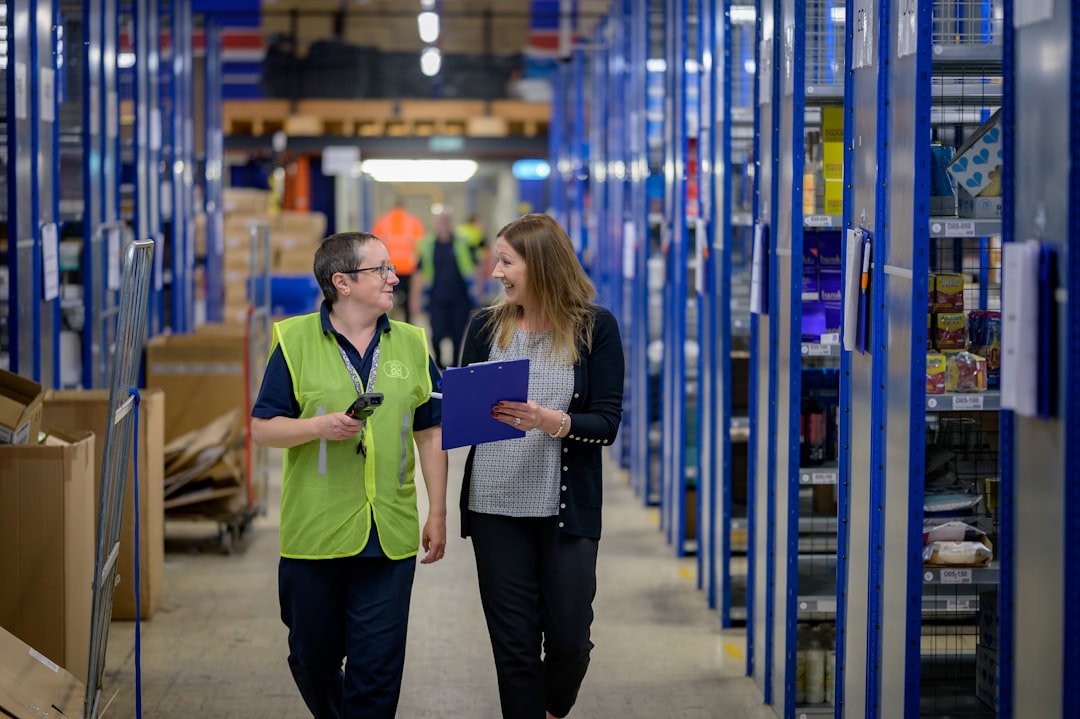
The integration of the Internet of Things (IoT) is revolutionizing real-time inventory tracking in 2024, significantly impacting how manufacturers manage their stock. This integration empowers businesses to gain instant visibility into inventory levels and locations, boosting efficiency and reducing errors. Technologies like Radio Frequency Identification (RFID) and other IoT sensors are playing a crucial role in enhancing accuracy across inventory processes. This granular level of data helps streamline the process of replenishing stock, minimizing delays and ensuring products are available when needed. The adoption of cloud-based solutions is also central to this trend, enabling seamless data sharing and collaborative decision-making. This facilitates faster and more informed responses to changing demand, contributing to better inventory management practices. However, it's crucial to maintain a critical perspective and ensure that the reliance on automated systems doesn't eclipse strategic human oversight. A balanced approach, carefully considering the strengths and weaknesses of automation, is vital for avoiding potential pitfalls and maximizing the positive impacts of IoT integration on inventory management within manufacturing.
Integrating the Internet of Things (IoT) into inventory management is rapidly changing how manufacturers track and manage their stock. By embedding sensors within the manufacturing environment, we can gain near-instantaneous visibility into the location and quantity of inventory. This constant stream of information empowers automated systems to react to changes in stock levels, potentially eliminating human errors and delays often found in traditional tracking methods. For example, sensors can trigger automatic reorder requests when stock falls below a certain threshold, optimizing the supply chain and reducing the risk of stockouts. While this hyper-automation seems promising, there's the ever present question of how it will impact human involvement in these formerly manual processes.
The use of technologies like Radio Frequency Identification (RFID) in conjunction with IoT is allowing for a dramatic decrease in the cost of holding inventory. By precisely tracking inventory in real-time, companies can optimize their storage and handling, potentially reducing holding costs by up to 30%. It's clear that this level of precision could lead to a shift in how we manage warehousing and inventory storage in the future.
IoT adoption has demonstrably boosted inventory accuracy rates. Some companies report accuracy rates over 95%, a considerable improvement over more traditional methods. This enhanced accuracy translates directly to improved operational efficiency and fewer discrepancies in inventory records. However, it's important to note the challenges inherent in these systems: with the increase in connected devices comes a vast influx of data. It’s estimated that manufacturing operations can generate up to a terabyte of data every day with these systems. Successfully leveraging all this data for valuable insights is a task that presents its own set of challenges.
Beyond just tracking inventory, IoT data can be used to identify bottlenecks and inefficiencies within the manufacturing process itself. By analyzing the flow of materials and identifying areas where delays are occurring, companies can streamline their workflows and potentially reduce manufacturing costs by up to 20%. In addition, this increased awareness can be very beneficial to those who work in the manufacturing space.
IoT sensors can also be invaluable for manufacturers handling sensitive goods. By monitoring environmental factors like temperature and humidity, manufacturers can ensure that products remain within the optimal conditions for storage. This capability is vital for industries dealing with perishable goods or materials susceptible to damage from environmental changes, minimizing waste due to spoilage or deterioration.
Integrating IoT data into predictive maintenance models shows promise. For instance, by analyzing inventory turnover rates and equipment usage data, manufacturers may be able to better anticipate when to replenish stocks based on expected wear and tear. While still nascent, this approach offers a fascinating possibility for preventative maintenance and potentially less downtime.
One of the most impactful benefits of adopting IoT for inventory management is a noticeable improvement in customer experience. Manufacturers have reported reductions in order fulfillment times by up to 50% with improved accuracy. Clearly, this enhanced responsiveness results in higher customer satisfaction and a more efficient supply chain, which has significant implications for companies.
Further improvements in supply chain efficiency can be seen in reduced order cycle times. Companies that have embraced IoT-based tracking have reported order cycle reductions of close to 30%. This is a notable increase in efficiency compared to more traditional methods, showing how this technology can reshape operations.
Despite the advantages offered by IoT technologies, it's striking that a substantial portion of manufacturers continue to rely on manual inventory tracking. An estimated 40% of manufacturers still stick to the older ways of doing things, implying there's a significant opportunity for further adoption of these technologies in the industry. Understanding the reasons for this resistance and finding ways to address concerns around cost, data management, and training are key factors in expanding the adoption of more intelligent inventory solutions.
The integration of IoT within the realm of inventory management is clearly a field in motion. While we are beginning to understand the immense potential of these technologies to transform how manufacturers oversee their inventory, there are challenges that still need to be addressed and hurdles that need to be overcome. As the future unfolds, it will be fascinating to watch the pace at which these technologies evolve and integrate themselves within the modern manufacturing environment.
Emerging Trends in AI-Driven Inventory Management Software for Manufacturing in 2024 - Machine Learning Algorithms for Optimizing Stock Levels
Machine learning algorithms are playing a growing role in optimizing inventory levels, especially within manufacturing environments. These algorithms delve into historical sales data and customer purchasing patterns to drive more dynamic inventory replenishment, ensuring that stock levels better match current demand. They help avoid the pitfalls of both overstocking and stockouts by calculating factors like economic order quantities and establishing minimum and safety stock thresholds. This, in turn, supports smoother manufacturing processes. While promising, manufacturers need to acknowledge that machine learning models can be susceptible to biases within the data they utilize and require consistent refinement to remain accurate and relevant in the face of rapidly changing market conditions. Ultimately, incorporating machine learning into inventory management signifies a move toward more adaptable and productive manufacturing processes.
Machine learning algorithms are increasingly being used to refine inventory management by optimizing stock levels. These algorithms can analyze historical data with a precision that potentially eliminates the need for the traditional 10-30% safety stock buffer many companies carry. This allows for leaner inventories without compromising service levels, a significant benefit for manufacturers.
Surprisingly, these algorithms aren't limited to historical data. They can also incorporate external information such as social media trends to anticipate demand spikes. For instance, if a product becomes a trending topic, the algorithm might suggest increasing inventory to meet the potential surge in demand. This highlights how consumer behavior and evolving market sentiment can influence optimal inventory levels.
Algorithms like reinforcement learning offer the capability to adapt stock levels dynamically based on real-time sales and market conditions. These systems "learn" over time and make adjustments, much like adaptive control systems found in other engineering fields. This adaptability is particularly valuable in volatile markets.
Some machine learning techniques, such as clustering algorithms, allow manufacturers to segment inventory items and develop tailored stock levels based on their performance rather than a one-size-fits-all approach. This individualized approach can significantly reduce inefficiencies related to overstocking slower-moving goods or understocking fast-moving ones.
Researchers have found that weather patterns can influence stock level optimization. For example, forecasts of cold weather can predict a surge in demand for winter clothing. Incorporating weather data into algorithms improves prediction accuracy and helps manufacturers prepare for these demand fluctuations.
While the promise of these methods is exciting, it's important to be cautious. Machine learning models can exhibit biases if their training data is not diverse and representative. If historical patterns used for training don't accurately reflect changing market conditions, the algorithms might reinforce undesirable stock misallocations.
Properly implemented stock optimization algorithms can demonstrably reduce excess inventory by at least 15%, freeing up capital that can be reinvested in other operations or technologies. This benefit can contribute to improved profitability and operational flexibility.
These algorithms can outperform traditional replenishment methods in terms of accuracy. Based on learning from historical data, the systems can predict restock needs with around 90% accuracy. However, it's important to remember that this performance heavily depends on the quality and completeness of the available historical data.
More recent advancements involve the use of generative models that simulate various stock level scenarios. This capability lets manufacturers explore the potential impact of multiple variables on inventory, leading to more insightful decision-making regarding stock management.
Despite the evident potential, many manufacturers are still reluctant to fully embrace machine learning for stock optimization. Concerns around integration complexity and the need for specialized personnel to manage sophisticated analytics platforms remain a barrier to wider adoption. Overcoming these challenges is critical to realizing the full potential of this technology.
Emerging Trends in AI-Driven Inventory Management Software for Manufacturing in 2024 - AI-Powered Supplier Management and Risk Assessment
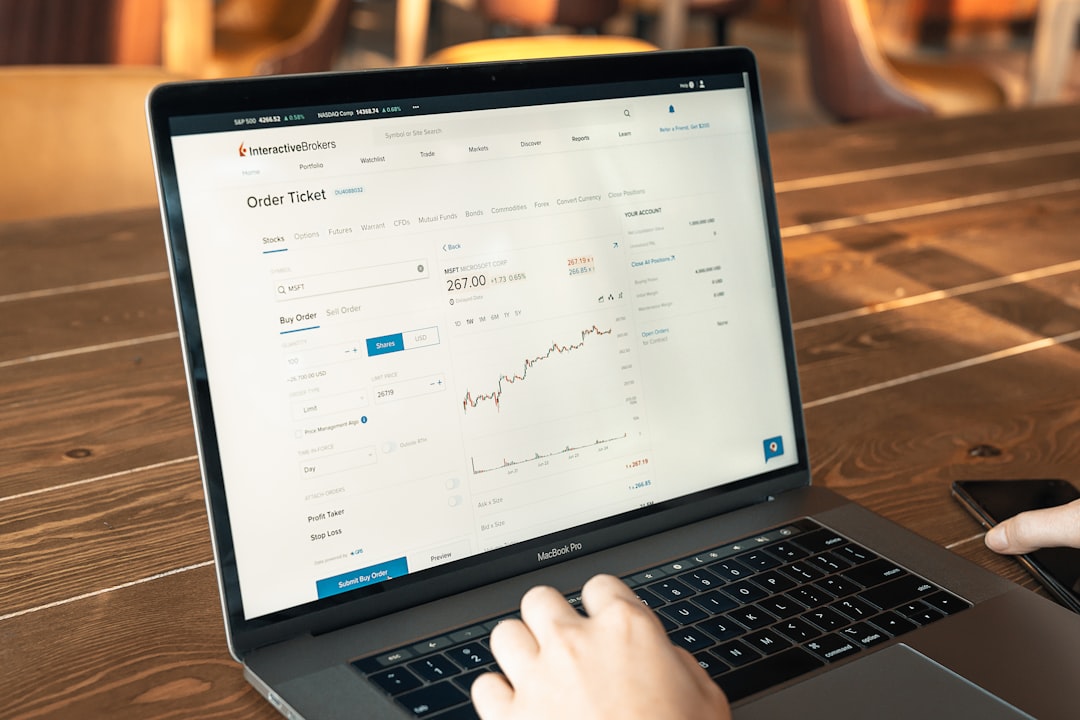
AI is increasingly influencing how manufacturers manage their suppliers and assess related risks in 2024. These AI-powered tools help businesses evaluate supplier performance, improve sourcing processes, and anticipate supply chain disruptions more effectively. The use of generative AI and large language models is particularly noteworthy, as they allow for analysis of vast amounts of information, including unstructured data. This capability empowers companies to better understand and respond to potential risks lurking within their supplier network.
It's important to recognize that while these technologies are promising, there's a potential for over-reliance on AI's capabilities. Relying too heavily on automation could inadvertently lead to a diminished focus on the more qualitative aspects of supplier relationships, where human understanding and experience play an important role. Achieving optimal outcomes in this area requires a measured approach, skillfully integrating AI's analytical prowess with human experience and insight. A balanced approach that leverages AI’s strengths while also acknowledging its limits will undoubtedly be crucial to the success of managing risk and building robust supply chains in this era of rapid change.
In the evolving landscape of manufacturing, AI is increasingly influencing how we manage suppliers and assess related risks. AI-driven tools now assess supplier risk by examining a wide range of factors, from financial health to past delivery records. This holistic approach helps companies anticipate potential disruptions and strengthens the overall supply chain's robustness. It's interesting how these AI systems can sift through vast amounts of data to provide a comprehensive view of a supplier's capabilities and vulnerabilities, something humans alone would struggle with.
We're seeing the use of natural language processing (NLP) applied to supplier reviews across various online platforms. By analyzing the sentiment expressed in these reviews, manufacturers can get a real-time sense of supplier performance. Not only does this provide a window into past actions, but it also helps in predicting future reliability, an aspect that's traditionally been very difficult to quantify. I'm intrigued by how this can help move beyond just hard metrics and consider the intangible 'feel' surrounding a particular supplier.
Traditional supplier risk assessments are often static, but AI is changing this paradigm. Risk profiles are now dynamically updated as new information emerges, ensuring that they're always up to date. This adaptability is a crucial element in an era marked by volatile markets and unpredictable events. Imagine being able to understand a supplier's shifting reliability in real-time.
AI can simulate various supply chain scenarios, including those impacted by geopolitical events or industry-wide trends. These simulations can help businesses understand how these external forces could affect relationships with their suppliers, fostering informed decision-making. The ability to model different scenarios and understand the possible outcomes certainly adds another layer of preparedness for navigating the complexities of global manufacturing.
Keeping tabs on supplier compliance with regulations is vital, but it can be tedious. AI-powered solutions are automating this process, relieving the pressure on human teams. Some studies suggest that automation could lead to a significant reduction in compliance-related penalties due to increased vigilance. This is an example of where automation can contribute to efficiency and minimize risk, albeit at the cost of removing some aspects of human judgment.
With the help of machine learning, it's now possible to benchmark a supplier against industry standards and compare them with competitors. This approach allows manufacturers to pinpoint those suppliers that aren't performing up to par, leading to potential improvements across the entire supply chain. It's fascinating how AI is helping us level the playing field and create a more balanced and competitive environment.
Forensic data analytics within supplier assessments offers the chance to uncover hidden fraud or discrepancies in supplier data that may escape traditional audit methods. This adds another layer of integrity to supplier relationships, but also raises questions about data privacy and the potential for unintended biases within these systems.
Research into the application of machine learning to predict supplier failures is promising. By identifying patterns in past performance data, these models can predict potential issues before they become critical. This anticipatory capability allows manufacturers to proactively identify and switch to alternative suppliers, which would be invaluable for reducing disruption. While the potential for a 75% accuracy rate in such predictions seems high, I'm keen to understand the limitations and potential biases inherent in such predictive models.
The automation of supplier selection is another area where AI is making a mark. Algorithms can weigh factors like cost, compliance history, and past performance to automate the selection process, reducing the amount of time human teams need to spend on manual evaluations. However, a concern arises here – how do we ensure that automation doesn't stifle creative decision-making in a critical part of a supply chain?
Finally, we're seeing a shift towards a more quantitative approach to assessing supplier risk. AI-generated metrics don't just measure risk, but also the potential financial consequences of supplier failures. This quantitative perspective offers a clearer understanding of the trade-offs involved in each supplier relationship, and is vital for the future of risk management within manufacturing.
It's clear that AI is ushering in a new era of supplier management. While the benefits of increased automation and insight are undeniable, we must remain mindful of the limitations of these systems. Understanding and mitigating potential biases, and the delicate balance between AI and human decision-making, will be crucial for ensuring a successful and robust application of these powerful tools within manufacturing.
Emerging Trends in AI-Driven Inventory Management Software for Manufacturing in 2024 - Automated Replenishment Systems Using Natural Language Processing
Automated replenishment systems are increasingly leveraging Natural Language Processing (NLP) to improve how humans interact with inventory management software. NLP enables these systems to understand both spoken and written instructions, allowing for more intuitive and efficient communication. This can streamline operations by minimizing the chance of misinterpretations that can slow down processes. Furthermore, these systems can adapt to fluctuations in demand, supplier lead times, and other dynamic aspects of manufacturing, with the goal of fine-tuning stock levels. This dynamic approach aims to reduce the likelihood of holding excess inventory or experiencing stockouts, a constant challenge for manufacturers. However, it is important to acknowledge the risks associated with relying solely on automated systems. Over-reliance can obscure the value of human experience and judgment, and the data used to inform decisions may contain biases that could negatively affect inventory management. As manufacturers adopt NLP-driven automated replenishment, striking a balance between human oversight and automated decision-making is critical to maximizing the benefits of these advancements.
Automated replenishment systems are increasingly leveraging natural language processing (NLP) to enhance inventory management in 2024. This approach goes beyond traditional methods by enabling systems to understand and react to the nuances of human language. For example, they can analyze customer reviews and social media conversations to gauge product popularity and anticipate demand fluctuations. This is a more dynamic way to adjust stock levels compared to older techniques that rely solely on past sales figures. NLP also streamlines interactions with suppliers by automatically processing communications, spotting potential issues or delays in the supply chain before they cause major disruptions.
It's fascinating how these systems can decipher the context of language. They can discern genuine complaints from mere user expectations, leading to more informed forecasting decisions based on customer satisfaction. This level of understanding is especially useful when companies operate across multiple regions and need to consider different languages and cultures. These systems aren't constrained by fixed data entries – they adapt in real-time. If NLP algorithms pick up a sudden demand spike, they can trigger automatic reorders without human intervention. This continuous responsiveness is vital in today's fast-paced markets.
NLP's ability to sift through vast amounts of unstructured text data also allows these systems to identify emerging trends before they become widespread. This proactive approach enables manufacturers to adjust their stock levels accordingly and reduces the risk of stockouts. Moreover, the insights gained from these systems can be presented in easily understandable language, making them accessible to everyone involved, from engineers to managers.
Beyond customer feedback, NLP can also analyze maintenance logs and service communications to spot potential equipment failures. This predictive maintenance insight helps reduce disruptions due to breakdowns, leading to smoother operations. Similarly, NLP can help in staying on top of regulatory and compliance requirements for suppliers, potentially minimizing the risk of violations.
Furthermore, NLP's capacity for rapid data analysis makes it a valuable tool in handling unexpected events. For instance, during a crisis like a pandemic, NLP can analyze news articles and social media posts to understand the impact on supply chains, allowing for swift adjustments in inventory and sourcing strategies. While there are still complexities and potential biases to consider with NLP, it's clear that these automated replenishment systems are evolving to offer valuable capabilities for managing inventory in increasingly unpredictable times.
Emerging Trends in AI-Driven Inventory Management Software for Manufacturing in 2024 - Blockchain Technology for Enhanced Supply Chain Transparency
Blockchain technology is gaining traction as a way to make supply chains more transparent, particularly within the manufacturing sector. It operates by providing a decentralized, secure, and permanent record-keeping system that tracks the journey of goods from their source to the final customer. This enhanced traceability helps reduce administrative costs and strengthens trust between the various players in the supply chain. The pandemic served as a harsh reminder of how important strong supply chains are, leading many businesses to explore blockchain as a solution to improve visibility and adaptability. When combined with other technologies like the Internet of Things (IoT) and advanced analytics, blockchain can further streamline supply chain operations. This allows manufacturers to gain real-time insights and make faster, better-informed decisions. But, even with all its promise, blockchain's implementation comes with its own set of issues. Concerns regarding compatibility with other systems, the volume of data that needs to be managed, and the need to balance technological advancements with human oversight are still important considerations.
Blockchain technology has emerged as a potential game-changer for improving transparency within supply chains. Its core strength lies in establishing an immutable record-keeping system—a shared ledger where each transaction is permanently recorded and cryptographically linked to the previous one. This "once written, never changed" aspect makes it incredibly difficult to tamper with data, fostering trust among the various participants in a supply chain.
One of the most intriguing applications of this technology is in boosting the traceability of goods. This increased visibility across the supply chain reduces administrative overhead and improves confidence among stakeholders, especially when dealing with complex, multi-party transactions. The events surrounding the pandemic highlighted the need for resilient supply chains. The challenges brought on by COVID-19 prompted many organizations to look for new approaches to build in supply chain flexibility and the ability to manage unforeseen disruptions, leading to a rise in interest in exploring the benefits of blockchain.
It seems that combining blockchain with other technologies like the Internet of Things (IoT) and advanced analytics will play a key role in transforming how we manage supply chains. IoT-enabled sensors could feed real-time data directly onto the blockchain, providing near instantaneous tracking and visibility into the movement of goods. This, coupled with analytical tools that can extract valuable insights from the ever-growing blockchain dataset, could lead to improved decision-making across supply chains.
Blockchain also offers a compelling solution to a persistent problem in supply chains: proving product origin and verifying orders. Manufacturers can use this capability to demonstrate the provenance of their goods with verifiable and auditable data, allowing consumers or other entities within the supply chain to confirm the ordering history with ease. This enhanced transparency can also contribute to better price discovery, giving all participants a clearer view of the costs associated with getting products to market.
In addition, it’s been proposed that a “Triple Retry” framework be applied in circular supply chains. The goal of this concept is to build a framework that enhances the core design features around trust, traceability, and transparency. This concept is still in the research and development stage, but it highlights an area where blockchain’s strengths might have broader implications.
The application of blockchain directly addresses critical challenges within supply chains. Fraud, which has been a long-standing problem across numerous industries, can potentially be diminished through the increased transparency enabled by the technology. Moreover, many of the operational inefficiencies seen in traditional supply chain systems can be reduced by automating and streamlining processes.
Challenges remain however. Integrating blockchain technology with existing systems is a complex task that requires technical expertise. Also, questions around scalability and data privacy continue to be areas that need further research. Nonetheless, the inherent transparency and immutability features of blockchain make it a powerful tool for manufacturing and other industries looking to improve trust, efficiency and visibility in their supply chains.
Even as AI and machine learning-driven inventory management tools advance, the role of these types of technologies is likely to continue to be crucial for manufacturers. Digitalization is reshaping how operations are conducted, and advanced technologies like AI and blockchain are becoming more integrated. Despite integration challenges, the ability to improve resilience and operational excellence through these tools is a driving force behind increased investment in this area.
More Posts from :