AI-Driven Inventory Prediction 7 Key Advancements Shaping Retail in 2025
AI-Driven Inventory Prediction 7 Key Advancements Shaping Retail in 2025 - Machine Learning Models Reduce Forecast Errors by 50% in Retail Inventory
Machine learning models are proving highly effective in improving retail inventory forecasting, with the potential to slash forecast errors by up to 50%. This improvement stems from the models' capacity to adapt to the increasingly dynamic and intricate nature of retail, an area where traditional forecasting methods often struggle. By leveraging advanced algorithms that process data and identify patterns in real-time, these AI-driven systems allow retailers to fine-tune inventory levels, minimizing both overstocking and stockouts. This, in turn, helps retailers avoid costly inefficiencies and enhances customer experience through consistent product availability. Looking ahead to 2025, it's clear that AI-powered inventory prediction is becoming increasingly vital for retailers to maintain a competitive edge and boost operational efficiency.
Machine learning algorithms have proven remarkably effective at reducing forecast errors in retail inventory. By analyzing massive datasets, these models can adapt in real-time to subtle shifts in demand, something traditional methods often struggle with. This agility translates to a substantial improvement in forecast accuracy, with retailers reporting reductions in forecast errors by as much as 50%. Notably, these models can also incorporate external factors like weather or local events, adding a layer of complexity to prediction previously unattainable. This capability, combined with the ability to learn and adapt to seasonal and promotional trends, sets machine learning apart from more static forecasting techniques. It's intriguing to consider how these models, through analysis of customer purchasing behavior, can uncover hidden patterns and inform more strategic inventory deployment and timely restocking. While the potential benefits of machine learning in inventory forecasting are evident, it's also important to acknowledge the potential for biases within the models and the need for ongoing monitoring and refinement to ensure their effectiveness.
The implications of this increased accuracy extend beyond just numbers. Many retailers report improvements in service levels, suggesting that a more accurate forecast directly translates to better customer satisfaction and retention. This benefit speaks to the practical application of these advanced models and the overall positive impact on customer experience. This growing reliance on machine learning is also fostering a shift away from traditional, retrospective analytics. Retailers are now focusing on predictive insights, leveraging past data with greater precision to anticipate disruptions in the supply chain and adapt their inventory strategies accordingly. While there are still challenges and uncertainties in implementing these complex models, it's undeniable that the increasing adoption of AI and machine learning is reshaping the field of inventory management within the retail sector, driving greater efficiency and resilience in a rapidly evolving landscape.
AI-Driven Inventory Prediction 7 Key Advancements Shaping Retail in 2025 - AI-Powered Virtual Shopping Assistants Boost Customer Service Efficiency
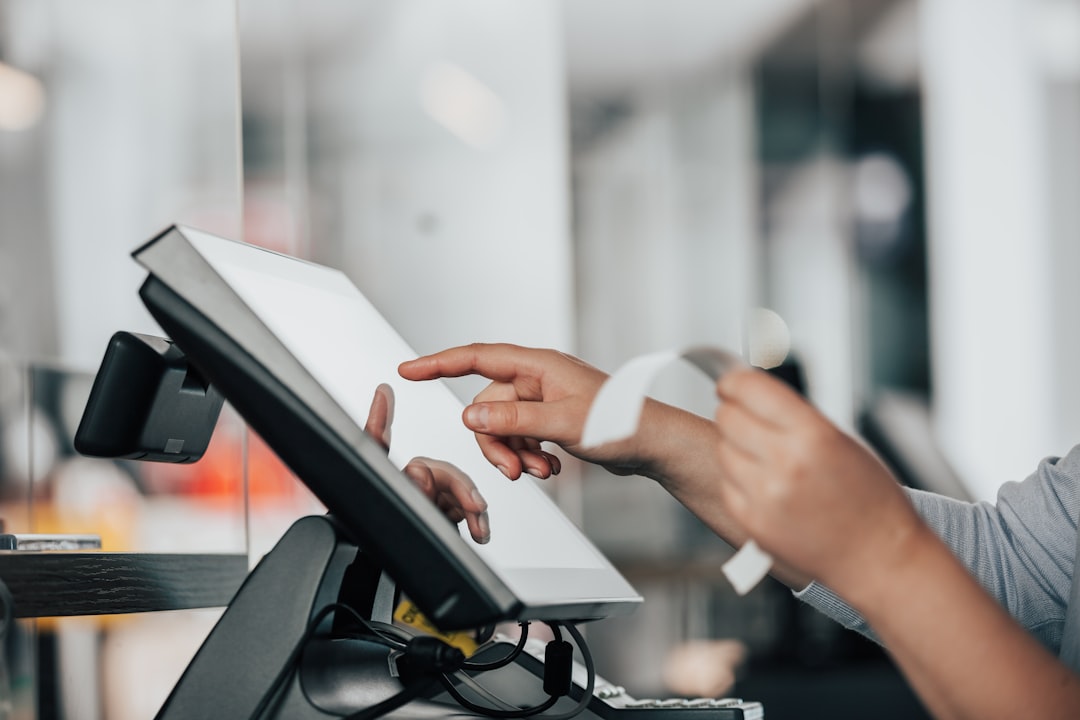
AI-powered virtual shopping assistants are emerging as a key way to improve customer service efficiency in retail. These virtual assistants, often powered by natural language processing, are capable of understanding and responding to customer questions in a conversational manner, much like a human associate. This allows retailers to address basic inquiries instantly, freeing up human employees to tackle more complex issues that demand personalized attention. We're seeing evidence that many shoppers prefer these virtual assistants for simpler tasks. This growing reliance on AI-powered assistants can help streamline operations and cut costs, all while creating more engaging shopping experiences. The ability to provide a personalized experience based on customer interactions is a significant shift in how brands engage with customers. This creates more opportunities to foster stronger connections and brand loyalty through these AI-driven interfaces, transforming the way retailers interact with consumers and influencing the future of the retail industry.
The integration of AI-powered virtual shopping assistants is significantly impacting the retail landscape, particularly in customer service. These assistants, like Amazon's Rufus, launched this year, are capable of handling a wide range of customer interactions, including answering questions directly within shopping apps. This has been instrumental during large events like Amazon's Prime Day, showcasing the potential for scaling customer support.
One intriguing aspect of these virtual assistants is their ability to understand and respond to customers in various languages, effectively breaking down language barriers and enhancing the experience for a global audience. Moreover, their ability to analyze customer sentiment allows for a more personalized and empathetic interaction, potentially increasing customer satisfaction. Interestingly, a large percentage of customers (74%) already favor AI chatbots for basic issues, indicating a growing acceptance and preference for this technology.
Furthermore, virtual assistants are not just passively responding to inquiries; they actively leverage data to enhance operations. They can integrate with social media platforms, providing real-time insights into current trends and customer preferences. This allows retailers to make more informed inventory decisions and quickly adapt to changes in demand. In fact, AI-powered assistants can handle a significant portion (up to 80%) of routine inquiries, freeing up human staff for more complex issues and leading to more efficient service delivery.
Beyond simple interactions, AI assistants are playing a role in enhancing sales. By leveraging recommendation algorithms, they can guide customers to products tailored to their preferences, leading to a noticeable increase in average order values. Additionally, they can conduct A/B testing on different customer interactions, helping retailers optimize their service strategies through data-driven insights. Their continuous learning ability allows them to evolve and adapt with customer preferences, becoming more effective over time.
While these advancements offer a more efficient and personalized customer experience, it's important to acknowledge the emergence of concerns related to data privacy. The need to handle user data responsibly and transparently is critical to maintaining customer trust in this evolving retail landscape. The future holds exciting possibilities as these assistants continue to integrate augmented reality features, offering customers immersive shopping experiences and the potential to significantly reduce returns. However, as these systems grow increasingly complex, they need to be able to manage even more nuanced and multi-part queries, showcasing a higher level of comprehension and adaptability in real-time. It will be interesting to observe how this technology continues to evolve, especially in the context of building trust and offering seamless customer experiences in the coming years.
AI-Driven Inventory Prediction 7 Key Advancements Shaping Retail in 2025 - Generative AI Adoption Accelerates Among Fortune 500 Retail Executives
Fortune 500 retail leaders are increasingly embracing generative AI, with a remarkable 90% actively experimenting and scaling its use. This rapid adoption mirrors a broader trend in retail, where the overall use of AI has skyrocketed to 72% this year, compared to about 50% just six years ago. Generative AI is proving valuable in refining operations, spanning tasks from optimizing inventory to improving customer experiences. This points to a significant change in how retailers manage their supply chains and engage with shoppers. Retail executives are particularly keen on leveraging generative AI in supply chain management and creating a better customer experience. This focus suggests a potential for major improvements across the retail industry. However, this swift move towards generative AI also prompts concerns about the long-term feasibility of these endeavors given the ever-changing expectations of consumers and the intricate nature of the technology.
Recent research suggests a significant surge in the adoption of generative AI among top retail leaders. A survey earlier this year found that a vast majority, around 90%, of Fortune 500 retail executives have started experimenting with these AI systems and are prioritizing certain use cases for scaling. This reflects a broader trend: AI adoption across companies has jumped to 72% in 2024, up from roughly 50% just six years ago. It seems like generative AI isn't just a buzzword—retailers are finding real-world applications.
It's interesting that these AI systems are impacting both customer-facing areas and back-office functions. Generative AI is helping streamline operations by enhancing things like demand forecasting and inventory management through sophisticated analytics. Some US and European retailers saw online sales climb by 35% within weeks of using AI to fine-tune marketing strategies during key shopping periods. This highlights the potential for these technologies to improve business outcomes.
The pace of adoption is quite rapid. Nearly half of the companies surveyed are moving fast on generative AI projects, and those with the most AI experience are staying on that fast track. This rapid uptake suggests a real sense of urgency and potential payoff. This enthusiasm for generative AI has even spilled over to other AI areas, showing a wider interest in the technology's capabilities.
It's no surprise that supply chain management and customer experience are key focuses for retailers using generative AI. Retail executives see clear potential in these areas. In fact, advisory experts have outlined several best practices for retail leaders to follow when adopting this technology, a sign that implementation isn't always straightforward. It also seems that generative AI's introduction into retail is prompting a ripple effect, leading to other areas of AI implementation within the same businesses. It's shaping how consumer-focused businesses operate.
However, while the potential is evident, questions about how to implement generative AI effectively remain. Only a relatively small number of businesses have fully integrated generative AI solutions, implying that the challenge lies in effectively scaling these systems and managing potential issues like data security and ethical use of AI. This is something to watch closely as the field develops further.
AI-Driven Inventory Prediction 7 Key Advancements Shaping Retail in 2025 - AI-Driven Inventory Management Leads to 25x Profit Growth for Retailers
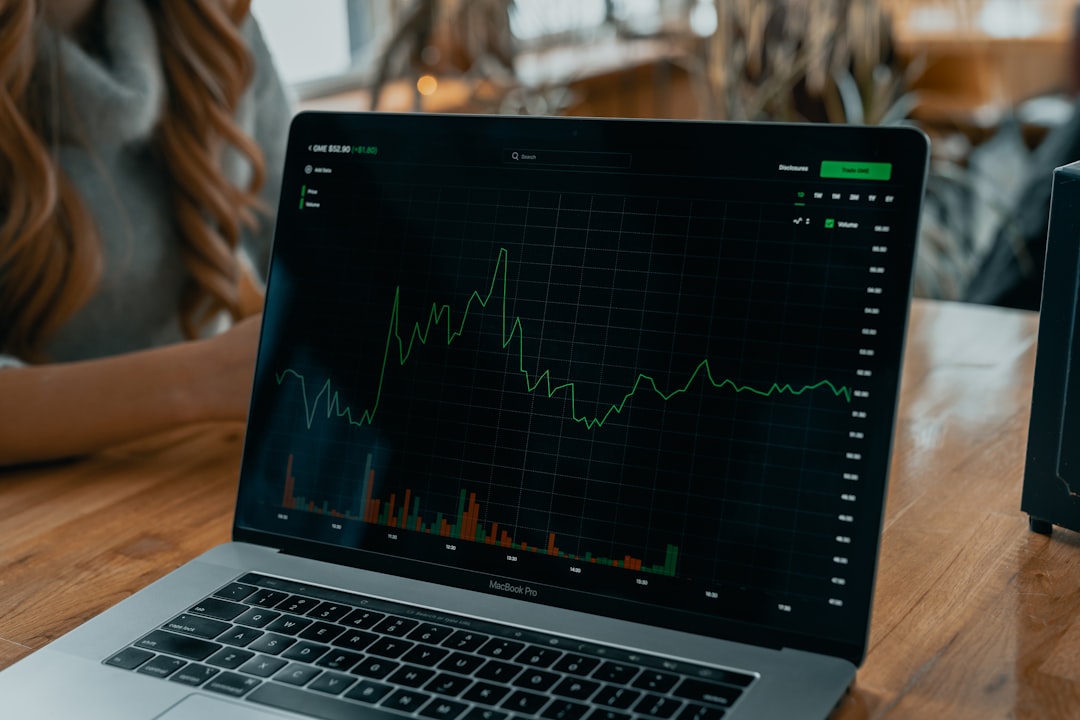
In the retail landscape, AI-powered inventory management is proving exceptionally effective, with some retailers experiencing remarkable profit increases—up to 25 times their previous levels. These systems use sophisticated algorithms to fine-tune inventory levels by analyzing sales data, customer behavior, and broader market trends to predict future demand with greater accuracy. The result is a significant reduction in both overstocking and stockouts, minimizing costly waste and improving operational efficiency. Furthermore, more accurate inventory management translates to higher customer satisfaction due to improved product availability. The growing reliance on AI within retail, along with the increasing size of this global market, suggests AI-driven inventory strategies will continue to evolve and transform the industry. Though promising, retailers need to be mindful of the complexities inherent in implementing AI systems and ensure that potential biases in these models are properly addressed.
AI's integration into inventory management has shown remarkable potential for retailers, with some achieving a 25-fold increase in profitability. This surge in profits seems to stem from the ability of AI to optimize inventory levels, leading to less waste and better alignment between what's stocked and what customers actually want.
Research suggests that AI-driven inventory systems can reduce wasted inventory by up to 30%. By analyzing sales history, customer preferences, and market trends, AI can predict demand with a high degree of accuracy—some systems claim up to 90% accuracy. This allows businesses to capitalize on increased demand without overstocking and incurring unnecessary costs. This heightened predictive capacity has a knock-on effect, leading to faster inventory turnover—some retailers have reported increases of around 40%. This agility can help retailers react swiftly to changes in customer preferences and maintain a relevant selection of goods.
Additionally, automation within these systems can lead to significant labor cost savings. Many retailers have experienced a reduction in labor costs by about 20%. This allows companies to redeploy human resources to more strategic tasks. It's particularly interesting that some AI systems are capable of tailoring purchasing decisions based on regional customer data. This shift toward localized inventory strategies can potentially boost regional sales by up to 50%, moving away from the traditional "one-size-fits-all" approach to inventory management.
These AI systems also foster a more responsive supply chain. Using real-time data from e-commerce platforms, they can adjust to shifts in purchasing patterns within hours instead of weeks. Furthermore, this responsiveness extends to the management of individual product variations, allowing for better planning for seasonal or limited-time promotional items. These improvements translate into logistics savings, with retailers seeing reductions of up to 15% in their logistics expenses.
The market's growing interest in AI-powered inventory management is reflected in projections that value this sector at around $10 billion by 2025. This rapid growth indicates a broader acceptance of AI's potential to transform retail operations and enhance financial performance. While it's promising, we need to keep a close eye on how these systems evolve, ensuring they remain effective, unbiased, and that the use of consumer data remains ethically sound and transparent.
AI-Driven Inventory Prediction 7 Key Advancements Shaping Retail in 2025 - Multi-Step AI Forecasting Process Revolutionizes Stock Level Accuracy
Retail inventory management is undergoing a transformation with the emergence of multi-step AI forecasting. This approach goes beyond simple, short-term predictions by leveraging advanced algorithms to forecast inventory needs over extended periods. While predicting future demand across multiple steps is inherently complex due to market shifts and unforeseen events, AI-driven models are increasingly capable of handling this complexity. These models utilize a combination of data inputs, including historical sales, current trends, and external factors, to provide more nuanced predictions. Furthermore, they can identify complex, non-linear relationships within the sales data that traditional methods often miss, improving the accuracy of these longer-term projections.
Although multi-step AI forecasting offers substantial improvements in inventory accuracy, it's not without its challenges. Retailers should carefully monitor the models for biases and ensure ongoing refinement to maintain their accuracy. The inherent complexity of these models can lead to unintended consequences if not carefully managed. As retail continues to become more data-driven, the capability to forecast accurately over longer timeframes will become increasingly valuable, allowing businesses to anticipate changes in consumer behavior and navigate a more volatile retail landscape. The adoption of this type of forecasting is likely to continue reshaping the field of inventory management, enhancing both operational efficiency and ultimately, customer experience.
The evolution of AI in retail inventory prediction is taking a fascinating turn with the rise of multi-step AI forecasting processes. Instead of the simpler, more generalized approaches of the past, these newer models are tackling inventory management with a more intricate and adaptable methodology. One interesting development is the way these AI systems handle data. Unlike traditional methods that treat all data points the same, multi-step AI processes assign different levels of importance or "weight" based on how relevant they are to the specific stock levels being predicted. This means recent sales spikes are given more consideration than older sales trends, resulting in more fine-tuned predictions.
Furthermore, these AI models are incorporating a wider array of data into their calculations. They pull data from diverse sources, like economic indicators, local events, and even social media trends. This "feature engineering" component allows the AI to create a broader, more holistic picture of the potential demand fluctuations which leads to more robust predictions. This differs from simpler models that rely on more limited data inputs.
Another crucial development is the introduction of causal inference techniques. These models strive to understand how various factors interact and influence one another, moving beyond simply identifying correlations between different variables. This deeper level of analysis, which isn't always present in traditional approaches, permits more nuanced decisions about inventory levels.
It's particularly noteworthy how these AI models adapt to new information in real-time. Instead of waiting for days for updates, these sophisticated systems can incorporate and adjust based on the latest data in seconds. The speed at which these adjustments are made can give businesses a considerable advantage in an environment with shifting market conditions.
Moreover, some models use a technique called "ensemble learning" which combines predictions from multiple different AI models to improve accuracy. This approach reduces the possibility that any single model may be overfitted to a specific dataset, increasing overall resilience in the face of changing conditions.
Some of these AI models have incorporated the ability to explore multiple future possibilities. This "scenario planning" approach enables retailers to see potential risks or rewards of various inventory strategies. This becomes especially helpful when preparing for unexpected disruptions like supply chain problems or large spikes in demand.
The level of detail captured in these newer systems is quite impressive. The AI is able to analyze how customers behave at a granular level and identify nuanced micro-trends like individual buying habits. This level of customer insight can assist in creating more specialized inventory strategies that better align with changing consumer preferences.
Many of these AI models also include "feedback loops" where they can refine their predictions over time. As the model's predictions are compared with actual sales figures, it adjusts parameters to enhance the precision of its future forecasts, essentially creating a continuous self-improving cycle.
In addition to refined prediction capabilities, some models utilize advanced simulation techniques, like Monte Carlo simulations, which produce a wide range of possible inventory scenarios, giving a more realistic understanding of potential futures.
Finally, beyond simply predicting stock levels, these newer AI systems are designed to interact with supply chain management tools. This integration streamlines the flow of data between forecasting and inventory deployment which translates into a smoother and more efficient logistical process compared to earlier approaches.
While the retail industry continues to evolve at a rapid pace, it appears that multi-step AI forecasting will become a crucial component of inventory management. It will be interesting to see how the continuous development of these AI models impacts forecasting accuracy and retailers' ability to navigate a complex and dynamic market.
AI-Driven Inventory Prediction 7 Key Advancements Shaping Retail in 2025 - Retail Sector Becomes Second-Largest AI Spender Globally in 2025
By 2025, the retail industry is projected to become the second largest investor in artificial intelligence worldwide, indicating a substantial change in how retailers utilize technology. This trend is reinforced by a large portion of retailers using AI reporting lower operating costs, demonstrating its effectiveness in enhancing efficiency. While AI adoption is advancing rapidly, concerns about job displacement and ethical issues surrounding data use and privacy remain present. As retailers leverage AI for diverse tasks such as managing inventory and improving customer experiences, successfully navigating the complexities of balancing the benefits of automation with the potential impact on jobs will remain a critical issue. The expected increase in AI investment highlights its potential to reshape the entire retail landscape.
By 2025, the retail sector is expected to become the second-largest investor in AI globally, trailing only the financial services sector. It's projected that spending will surpass $27 billion, highlighting the growing understanding of AI's potential to improve operations and boost profits. This shift seems to suggest that retail leaders are realizing that AI isn't just a fad but has the potential to fundamentally change how they operate.
Some retailers using AI-powered inventory prediction systems are seeing a remarkable impact on their bottom line—profit increases of up to 25 times compared to traditional methods. This kind of dramatic impact showcases how AI can help retailers get a better grasp of what consumers are buying, which is pretty important for any business.
We're seeing a trend towards using more advanced algorithms that can analyze huge amounts of data all at once, which can lead to inventory prediction accuracy as high as 90%. This kind of precision lets retailers adapt to changes in consumer preferences in almost real-time, which is really quite significant in today's rapidly changing world.
It's interesting that incorporating AI systems can also bring about noticeable reductions in labor costs. Some retailers have reported decreases in staff needs by around 20% due to automation within inventory management processes. This seems to be allowing companies to redirect human resources to more strategic, high-level tasks, instead of spending lots of time on routine inventory tasks.
Multi-step AI forecasting approaches are changing the game in terms of prediction length. Some AI models can now predict inventory needs up to a year in advance, a major jump from traditional methods that often only predict short-term trends. It's unclear if this new level of accuracy will be sustainable in the long run, especially given the volatility in the market.
Research is showing that AI systems can reduce inventory waste by up to 30% by providing data that better aligns stock levels with consumer demand. This isn't just about preventing financial losses, it suggests a more efficient use of resources. One has to wonder if this means we could see a reduction in the environmental impact of retail as a result.
AI-powered inventory management can integrate data that traditional methods typically don't include. These systems are now able to incorporate outside influences, like broader economic trends and even social media sentiment, helping them predict trends before they show up in sales data. The challenge will be making sure that this sort of data integration doesn't lead to biased results or unforeseen outcomes.
Implementing ensemble learning within AI inventory management is helping to improve forecast accuracy. By combining the outputs of multiple AI models, retailers can avoid relying on the results from a single model. This is an interesting approach for improving resilience in the face of shifts in the market and customer behavior.
Some advanced AI models have incorporated a process called scenario planning, which helps analyze a wider range of potential outcomes. This lets retailers develop plans that address various risks and scenarios. This is important in the retail world, where change seems to be the only constant.
The huge amount of data that AI systems analyze allows them to understand customer behavior at a very detailed level. This detail permits retailers to use hyper-targeted inventory strategies that are very closely aligned with the buying patterns of specific customer groups. The question becomes, how can we make sure that this granular level of personalization doesn't inadvertently lead to biased outcomes or practices that some might find unfair or exploitative?
AI-Driven Inventory Prediction 7 Key Advancements Shaping Retail in 2025 - AI Market Size in Retail Projected to Reach $405 Billion by Year-End
The retail sector's embrace of artificial intelligence is expected to result in a substantial market, potentially reaching a value of $405 billion by the end of this year. This projected growth signifies a major trend, with retailers increasingly utilizing AI to optimize their operations. The benefits of AI adoption, especially in areas like inventory management, are becoming more evident, including improved revenue and streamlined operational costs. This success in integrating AI is driving its expansion, but raises concerns about how consumer data is being used and the potential impact on employment within the retail workforce. As AI's role in retail expands, retailers face the challenge of realizing AI's potential advantages while managing the complex issues that its adoption presents. The rapid pace of change in this space requires retailers to be proactive in anticipating and navigating the implications of this evolving technology.
Estimates suggest the AI market within retail will reach a remarkable $405 billion by the end of 2024. This substantial figure underscores the escalating integration of artificial intelligence into retail operations, driven by a growing need for efficiency and optimization. It's fascinating how these AI-driven systems are transforming traditional approaches, particularly in areas like inventory management. For instance, AI-powered methods can significantly cut down on storage costs, with some estimates suggesting a potential decrease of 30% due to more precise control of stock levels.
It's also notable that a large portion of Fortune 500 retail executives (90%) are now experimenting with generative AI. This trend implies a broader shift towards harnessing AI for more complex tasks, moving beyond simpler administrative roles. One of the more impressive capabilities of AI is its ability to analyze real-time data from e-commerce platforms, allowing retailers to adjust inventory levels in seconds, as opposed to the days or weeks required by older methods.
The development of multi-step AI forecasting is particularly interesting. These models can predict inventory needs up to a year in advance, which is quite a leap from previous approaches that were largely confined to short-term forecasts. This is especially important given the ever-shifting nature of consumer demands and market conditions. The impact of precise AI-driven inventory management on profitability can be significant, with some retailers reporting a remarkable 25-fold increase. It's intriguing to observe how AI can translate into such a tangible financial benefit.
Beyond just internal sales data, AI models are capable of incorporating external factors into their predictions. This could include economic indicators, regional events, or even social media sentiment. It's quite interesting how a wider array of inputs can help create a more nuanced understanding of how demand can change. Another aspect that seems to be gaining traction is the ability for AI to analyze consumer sentiment on social media. While this capability raises some concerns regarding data privacy and potential biases, it also presents a unique opportunity for retailers to gain a deeper understanding of customer desires and adapt accordingly, pushing the boundaries of what we traditionally consider “sales data.”
It's worth noting that AI-powered systems can also lead to significant labor cost reductions. Reports suggest that AI adoption has led to about a 20% reduction in the need for human labor in certain aspects of inventory management. While this might spark concerns about job displacement, it's also interesting to consider the potential for human employees to be freed up for more strategic or value-added tasks within the organization. A notable technique gaining popularity in AI-driven inventory management is ensemble learning. By combining predictions from multiple AI models, retailers can improve the robustness and overall effectiveness of their inventory strategies. This approach helps ensure the AI isn't overly reliant on any one particular model or dataset, improving the ability to adapt to changes in consumer behavior and market trends. It remains to be seen how these emerging trends will continue to reshape the retail landscape in the future, but it is exciting to imagine the possibilities.
More Posts from mm-ais.com: