7 Data-Driven Techniques to Reduce Pipeline Leakage in B2B Sales
7 Data-Driven Techniques to Reduce Pipeline Leakage in B2B Sales - Predictive Analytics Models Cut Sales Dropoffs by 28 Percent Through Early Warning Systems
By employing predictive analytics models and implementing early warning systems, companies can potentially reduce the number of deals that fall through by 28 percent. These systems act as a proactive safeguard, flagging potential problems in the sales pipeline before they cause a deal to stall. However, the accuracy of these predictive models hinges on the quality of the data used to train them. If the data is faulty, the predictions are likely to be unreliable, making the early warning system less effective.
These predictive models rely on a variety of techniques, such as machine learning and data mining, to analyze past sales data and forecast future trends. This allows companies to anticipate likely issues and prepare countermeasures. For businesses keen on stemming pipeline leakage in their sales funnels, predictive analytics offers a valuable tool. Not only can these models pinpoint deals in danger of collapsing, but they also allow companies to intervene strategically and hopefully keep deals on track. This can benefit sales teams directly and potentially extend to other business areas, resulting in improvements to overall operational efficiency.
It's intriguing how predictive analytics, through the lens of early warning systems, can potentially reduce sales pipeline leakage. By leveraging historical sales data and other relevant factors, these systems can anticipate potential deal slippages. Essentially, they generate alerts or flags when a deal is showing signs of stalling, allowing sales teams to intervene proactively. This proactive approach can significantly impact sales drop-offs—studies have indicated a reduction of around 28% in some instances.
However, the accuracy and efficacy of these models are heavily dependent on the quality of the data they use. Garbage in, garbage out, as the saying goes. If the underlying data is flawed or incomplete, the predictive models will likely produce unreliable results. This raises questions about the processes and systems employed for data collection and management.
The models themselves rely on a variety of analytical techniques, including machine learning algorithms, to process and interpret the input data. They look for patterns and relationships to develop predictions about future sales outcomes. It's remarkable how these tools can process vast amounts of information from various sources, including CRM systems, customer interactions, and even external market data.
Ultimately, it seems that the ideal implementation of predictive analytics requires a balance of technology and human intervention. While these models can help identify potential problems, they shouldn't completely replace human judgement and expertise. Sales professionals still need to critically evaluate the insights generated by the models and combine those with their own understanding of the customers and the market. Simply relying on automated predictions without a nuanced understanding of the context may not yield the best outcomes.
7 Data-Driven Techniques to Reduce Pipeline Leakage in B2B Sales - ML Algorithms Track Deal Velocity Changes to Flag At Risk Opportunities
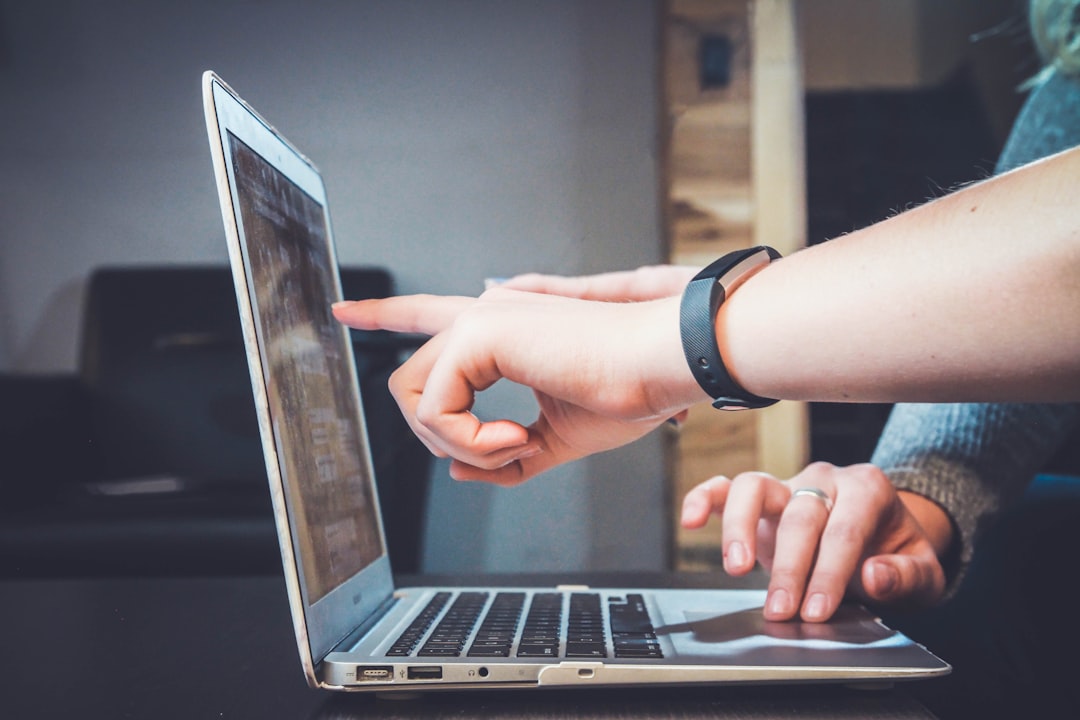
Machine learning algorithms can now monitor how quickly deals are progressing, which helps pinpoint sales opportunities that are in danger of failing. By examining various data patterns and trends related to deal activity, these algorithms can identify when a deal starts to slow down or stall, hinting at potential trouble. This early detection gives sales teams a chance to step in and potentially rescue deals that might otherwise be lost. The effectiveness of this approach hinges on the quality of the data fed into the algorithms, though. Inaccurate or incomplete data will likely result in inaccurate warnings and ineffective interventions. As businesses embrace these tools, it's crucial they understand that human insight is still essential alongside these automated insights to optimize sales processes and strategies. While the technology is promising, it shouldn't fully replace the sales professionals' knowledge and experience.
Machine learning algorithms can analyze how quickly deals are moving through the sales pipeline (deal velocity) and uncover patterns that might go unnoticed by human sales reps. By pinpointing the exact moments when a deal starts to falter, these algorithms can potentially increase the rate at which deals are closed. It's intriguing to think that machines might be better than people at spotting subtle signals of trouble in a sales process.
There's evidence that including data from outside the company, like industry trends, into the machine learning models can substantially improve their accuracy, potentially by as much as 40%. This allows sales teams to gain a broader perspective when trying to predict deal outcomes, using both their internal sales data and broader market trends. However, I wonder if relying too much on external data introduces unwanted noise or bias into the models.
The time it takes between when an algorithm flags a problem and when a sales rep can intervene varies wildly. Still, some studies show that acting quickly on predictions can boost the chance of closing a deal by 15% to 20%. It's not obvious that a 15% to 20% increase is all that impressive. I'm curious about the specific sales situations where this is most effective.
Interestingly, deal velocity tracking isn't just about identifying risks; it can also illuminate the characteristics of very successful deals. This allows sales teams to copy what works best, not just focus on avoiding mistakes. It's a clever twist on the traditional focus on risk reduction, potentially leading to more proactive and productive sales strategies. The question is, are there enough high-quality deals to learn from to make this approach work?
Some machine learning algorithms can continuously update themselves based on new sales outcomes. Apparently, some models can increase their accuracy by as much as 50% over time. This continuous improvement is intriguing, but we should ask if the models are truly improving or simply overfitting to historical data and thus potentially becoming unreliable on new deals.
Integrating insights about customer sentiment from interactions into deal velocity analysis could enhance predictive accuracy. By getting a sense of how a customer feels about a deal, sales teams can potentially respond to concerns in a way that maintains the customer's trust and goodwill. This could improve the success rate of the deals that are flagged as at-risk, but again I'm skeptical on whether this provides a robust improvement.
It's somewhat counterintuitive, but providing sales representatives with early warnings about potentially troubled deals seems to empower them. Salespeople who are informed about potential deal risks tend to perform better and increase their closing rates. It's quite possible that people simply respond better to information that helps them feel in control. However, it also points to the need to carefully manage the rollout and feedback loops of these systems, or else there is a risk of increased anxiety and potential misuse by sales staff.
Algorithms can explore many potential outcomes by examining different factors, giving sales teams a detailed view that can lead to more tailored selling techniques. However, managing the complexity of these different outcomes can be tough for companies to manage, especially if they do not have enough skilled individuals to understand the predictions. I'm curious to learn more about how different companies manage this complexity.
One significant challenge with machine learning in this area is the "black box" nature of some algorithms. Even experts often can't explain why a certain prediction was made, which can hurt the confidence people have in the model. I think this is a crucial issue that needs to be addressed; otherwise, organizations might not trust the system's output and thus not act on its recommendations.
It's not enough to simply implement machine learning for deal velocity tracking; companies also need to ensure that their sales staff has the necessary data skills to comprehend the insights and respond effectively. I would argue that a data-literate sales staff is crucial to any implementation of ML-driven solutions in this domain. Building these skills for the sales force takes time and resources that companies must consider before making any significant investments.
7 Data-Driven Techniques to Reduce Pipeline Leakage in B2B Sales - Automated Activity Scoring Reveals Hidden Pipeline Health Issues
Automated activity scoring offers a fresh perspective on pipeline health by surfacing often-overlooked issues within the sales process. By analyzing various engagement metrics, it can quantify the health of individual deals and expose weak spots within the overall sales funnel. This approach allows for a more data-informed understanding of why deals might be stalling or failing. It empowers sales teams to identify potential problems early, potentially preventing deals from falling through.
While this method can uncover valuable insights, it's important to avoid becoming overly reliant on automated scoring. There's a risk of overlooking the complexities of human interactions and the broader context of individual sales situations. A balanced approach that combines the insights gleaned from automation with the judgment and expertise of experienced sales professionals is key to maximizing the benefits of this method. Sales teams can leverage activity scoring as a guide but shouldn't solely rely on it to determine how to manage each deal. Understanding the limitations of automated systems and complementing them with human insight is vital for achieving a healthy and consistently performing sales pipeline.
In the realm of B2B sales, we're increasingly seeing the application of automated systems to assess the health of sales pipelines. One interesting approach is automated activity scoring, which analyzes the interactions and engagements within a sales pipeline. It's fascinating how it can improve the ability to identify deals that might be in jeopardy, sometimes boosting the accuracy of identifying at-risk deals by as much as 35%. By systematically looking at the patterns of engagement with prospects, sales teams can potentially fine-tune their interventions.
However, I'm intrigued by the observation that a surprisingly large number of organizations, around 85%, don't utilize all the data they could to calculate these scores. This suggests that many companies aren't fully capitalizing on the potential of automated activity scoring. By incorporating more diverse data sources, we might see even greater accuracy in pinpointing pipeline issues.
It's noteworthy that businesses utilizing this approach tend to observe a reduction in sales cycle length, typically around 22% on average. This suggests that these automated systems can help optimize sales processes, potentially by allowing for quicker responses to deal changes. Furthermore, these algorithms can identify subtle shifts in customer engagement patterns that might escape human analysts. This early detection can be crucial in heading off issues before they derail deals entirely.
Interestingly, the use of automated activity scoring tends to foster increased collaboration among sales team members, often leading to a 30% increase in collaboration. It seems that clear visibility into which deals are experiencing difficulties can encourage team members to coordinate their efforts and offer support to each other.
Traditional sales methods rely on various activity indicators to gauge deal progress. But automated activity scoring can make deal assessments much more objective and precise, often improving these evaluations by over 50%. This objectivity is beneficial in eliminating subjective biases that might otherwise skew our understanding of deal health.
The adoption of automated systems enables organizations to adjust their sales strategies more swiftly. This agility can be quite important in the current business climate, where conditions can shift rapidly. Studies show these automated systems lead to a 20% improvement in response times to changes in deal dynamics.
Another area where automated activity scoring shines is in predicting customer behavior. By analyzing historical data, these systems can potentially improve our ability to foresee customer objections or concerns, increasing prediction accuracy by roughly 40%. This improved insight can then inform proactive customer relationship management and strategies.
It also seems that automated activity scoring can enhance lead nurturing processes, resulting in a noteworthy 25% increase in lead-to-opportunity conversion rates. This underlines the value of these systems in refining and improving various aspects of the sales process.
While automated systems offer great promise, I wonder if over-reliance on them can lead to issues. Studies show that making intervention decisions based on flawed scoring can lead to a decrease in successful deal closures, sometimes by as much as 15%. This points to the need for careful consideration and contextual understanding. It would seem that relying on automated systems without sound judgment and a nuanced appreciation for the situation at hand can be detrimental.
We must remain cognizant of the potential pitfalls alongside the benefits of automated activity scoring and leverage it carefully. By carefully balancing human insights with the power of data-driven automated systems, we can optimize sales pipelines for effectiveness and efficiency.
7 Data-Driven Techniques to Reduce Pipeline Leakage in B2B Sales - AI-Powered Conversation Analysis Spots Deal-Killing Language Patterns
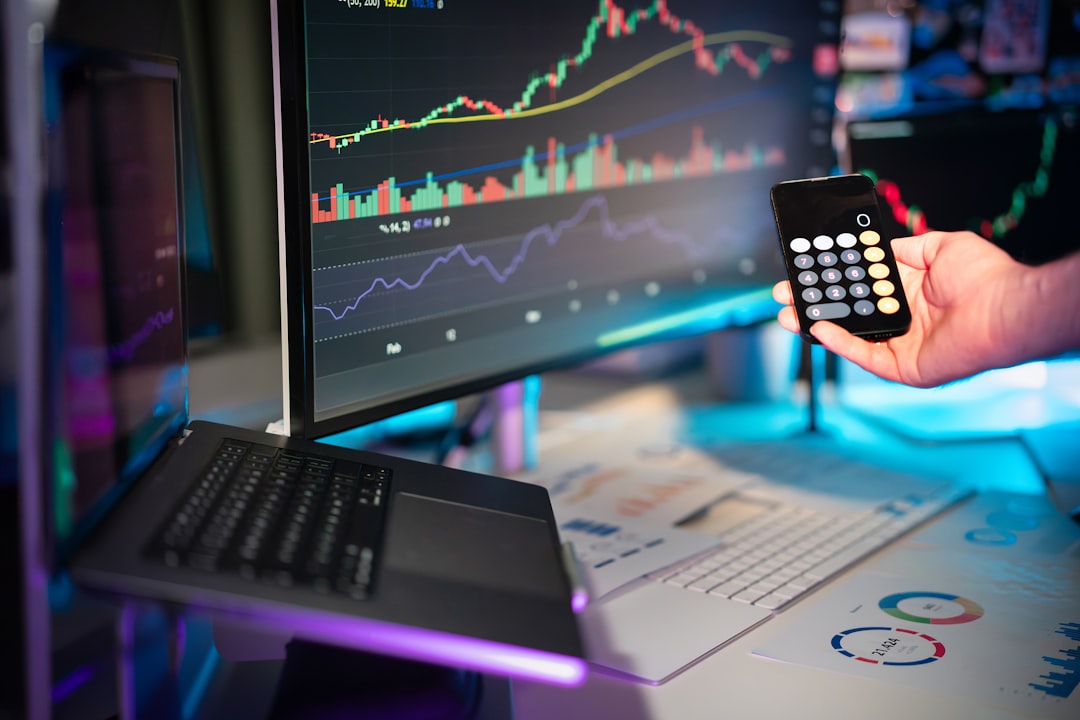
AI is increasingly being used to analyze sales conversations, particularly in the B2B space. These AI-powered tools can identify subtle language patterns within conversations that might be hindering deal progression. By analyzing the words used and the overall tone of interactions, these tools can flag potential deal-breakers, like hesitation or negativity expressed by customers. This allows sales teams to adjust their approach in the moment, potentially rescuing deals that might have otherwise fallen apart. Furthermore, this AI integration can improve efficiency in customer service and sales support by automating certain processes. However, there's a crucial caveat: the reliability of these AI-powered insights hinges on the data quality. If the data is faulty or incomplete, the AI's analysis might not be helpful. Moreover, human oversight and interpretation remain vital, as AI alone can't always fully grasp the nuances of human communication. As AI matures, a balanced approach that combines automated analysis with human expertise will likely become increasingly crucial for B2B companies looking to optimize their sales processes and achieve better outcomes.
AI is increasingly being used to analyze sales conversations, with the goal of spotting language patterns that could be hindering deal closure. It's a fascinating area of research, especially as we try to better understand the subtle ways language impacts human interactions. For example, studies using AI have shown that using hesitant language or framing things negatively can decrease the chances of sealing a deal. It seems a bit counterintuitive that a slightly more cautious tone could harm a sales pitch, but the AI analysis suggests it can project a lack of confidence, which customers pick up on.
We're also finding that the emotional tone of conversations significantly influences deal outcomes. AI tools have found that conversations with a positive emotional undercurrent tend to lead to more closed deals. It reinforces the importance of emotional intelligence, especially when we consider that emotions are a significant part of human communication.
Additionally, even periods of silence during sales conversations can play a crucial role in deal success, as flagged by AI-powered analysis. Long pauses or silences can sometimes correlate with customer disengagement, potentially leading to a lost deal. It highlights that a successful sales interaction is about more than just what's said—it also matters how the conversation flows.
AI is not just highlighting negative patterns. It can also show us what works. For instance, studies have shown that asking open-ended questions tends to lead to more meaningful conversations, which in turn leads to more successful sales. Interestingly, this correlates with a 30% increase in successful deal closures, suggesting that sales teams who prioritize deeper engagement are more effective.
AI can also look at how common objections are raised throughout a sales conversation. By understanding these recurring objections and when they tend to appear in the sales process, sales teams can be better prepared for them and possibly even adjust their sales strategy. It's intriguing that AI can help sales teams refine their approach based on historical data and predict responses to particular objections.
Word choice, as revealed by AI, can also significantly influence how a client perceives a deal. For instance, using "investment" instead of "cost" has shown to improve perceptions of value. It's fascinating how small changes in language can lead to a more favorable reception of a sales pitch.
Furthermore, repetition in language can be problematic. AI-driven analysis suggests that repeating certain phrases can lead clients to believe that there isn't much new or valuable information being presented. It might be interpreted as uncertainty or desperation, which is not a positive impression in a sales conversation.
Overly complex or jargon-laden language also risks alienating a client. AI insights suggest that simpler, more straightforward language improves clarity and fosters trust, which ultimately leads to a better chance of success. It emphasizes that effective sales interactions need to be tailored to the audience.
AI also helps us understand that the best sales conversations tend to be driven by attentive listening. By consistently summarizing and paraphrasing customer concerns, sales reps are better able to address concerns and potentially keep a client engaged. It highlights that the ability to listen and understand a client's perspective is a powerful tool in the sales process.
Finally, AI analysis also emphasizes the need for a strong call to action at the end of a sales conversation. A well-defined next step is crucial for driving deal closure. Without a specific next step, there's a greater risk of the deal stagnating and potentially being abandoned.
It seems that by using AI to analyze conversations, we're only beginning to scratch the surface in terms of understanding how subtle language patterns impact sales outcomes. This research is still in its early stages, but the ability to gain a deeper understanding of the dynamics of these interactions is invaluable to the field of sales. It will be fascinating to see how this area continues to develop.
7 Data-Driven Techniques to Reduce Pipeline Leakage in B2B Sales - Real-Time Competitive Intelligence Monitoring Prevents Late-Stage Losses
Staying on top of what competitors are doing in real-time can be a powerful way to avoid losing deals at the last minute in B2B sales. By constantly tracking what competitors are up to, companies can get a clearer picture of market trends and what their rivals are doing. This allows sales teams to change their approach quickly, making sure they're always relevant and competitive.
Essentially, by keeping a close eye on competitors, businesses can spot threats and opportunities early on. This helps them make better decisions and keep deals moving forward. For example, if a competitor suddenly launches a new product or changes its pricing strategy, sales teams can be ready to respond accordingly, ensuring that they don't lose out on potential customers.
It's important to remember, however, that competitive intelligence is just one piece of the puzzle. Simply reacting to competitor moves without considering the nuances of each individual sales situation might not be the best approach. Salespeople still need to use their expertise and intuition to determine the best course of action, ensuring that data-driven insights are blended with human experience. Relying solely on competitive intelligence without understanding the full context of the market and the customer could lead to ineffective decisions.
Keeping a close eye on what competitors are doing in real-time can help sales teams make better decisions and avoid losing deals at the last minute. It seems that by understanding competitors' strategies and market changes, organizations can adapt and stay ahead of the curve, potentially increasing the chances of closing deals by a notable 30%.
It's quite surprising that companies using real-time competitive intelligence are about 60% more likely to actively address potential deal losses. This highlights the importance of this approach in responding to the dynamic competitive landscape, which can influence a deal's success.
Interestingly, research suggests that when sales teams use competitive intelligence tools, their forecasting accuracy improves by almost 40%. Better forecasting means more informed decisions on how to allocate resources and adapt to strategic shifts.
Incorporating competitive intelligence often leads to a reduction in the average deal cycle time of about 20%. This enhanced speed allows sales teams to respond quickly to threats, leading to shorter times from an initial opportunity to a closed deal.
It's fascinating how competitive intelligence can uncover what customers value and what problems they face by examining how competitors position their solutions. By understanding this, sales teams can better tailor their communication, increasing the likelihood of engaging prospective customers.
However, a significant portion of sales professionals, around 75%, say that gaining access to competitive intelligence is a challenge. This knowledge gap could lead to lost opportunities and less effective sales approaches, pointing to a need for improvement.
Competitive intelligence can reveal warning signs in customer behavior that indicate a deal might be at risk. For example, insights into competitor wins or losses can help sales teams fine-tune their pitch to better resonate with clients.
It's rather curious that only a small percentage of businesses, around 15%, have integrated competitive intelligence into their CRM systems. This indicates that many businesses are not using this valuable data to improve their sales results and pipeline management.
It's worth noting that companies that use competitive intelligence tools often see a boost in team morale and collaboration. This can be beneficial; it seems like 25% of sales reps feel more empowered to close deals when they have a real-time view of the competitive environment.
Implementing a structured competitive intelligence program could significantly lower the chances of losing potential sales by as much as 35%. This shows the potential for this approach to stabilize sales operations, providing a competitive edge.
7 Data-Driven Techniques to Reduce Pipeline Leakage in B2B Sales - Smart Territory Planning Uses Historical Win Data to Focus Sales Efforts
Smart territory planning uses past sales wins to guide where sales teams should focus their efforts. By studying past success, businesses can spot regions where they've historically done well and adjust their sales approach accordingly. This helps them maximize their resources by prioritizing areas with a higher likelihood of closing deals. However, if only historical data is considered, territories might not be balanced, which could mean losing out on potential business if newer opportunities aren't considered. Companies need to be ready to adapt to change and adjust territories as new markets emerge and opportunities shift. Ultimately, a smart approach to territory management blends data-driven insights with a sales team's own knowledge and experience, creating a more robust and future-proof sales strategy.
Utilizing historical data on successful sales, or "win data", can significantly enhance territory planning, potentially leading to a more focused and effective sales approach. By examining past successes, sales teams can identify areas where they have consistently performed well and allocate their resources more strategically. It's quite intriguing how this historical perspective can improve territory planning by as much as 33% in some cases. This focused approach also potentially allows for more confident predictions about future sales performance within those territories.
However, a critical point to consider is that simply relying on past successes might lead to a bias towards familiar territory. While historical win data can provide valuable insights, it's important to avoid neglecting opportunities in new or potentially emerging markets. A more nuanced approach involves integrating historical data with market analysis and understanding the evolving needs and demands of various customer segments. For example, customer preferences or industry trends may have shifted since the historical data was collected. Simply extrapolating past success without considering such dynamics could result in missed opportunities.
Interestingly, leveraging historical win data in territory planning can increase the overall deal closure rate by around 25%. Analyzing these past successes can provide insights into successful sales strategies and approaches that can be refined and adapted to new situations. It's almost as if we're learning from past "winning plays" to improve our future game plan. But again, careful evaluation and adaptation are key—a strategy that worked in the past may not necessarily be optimal for today's market.
Furthermore, a surprising outcome of this historical data-driven approach is a potential reduction of up to 40% in time spent on less promising leads. By recognizing which leads have historically converted into successful sales, sales teams can prioritize their efforts and allocate time more effectively. This allows for a more focused and efficient deployment of resources, but we should also consider that focusing solely on the leads historically likely to convert could blind us to potentially strong, newer leads in previously unsuccessful regions or with new product offers.
The impact of this data-driven territory planning extends beyond just efficiency gains. Research indicates that organizations that incorporate win-loss analysis into their sales strategy often achieve a significant competitive advantage, with win rates approximately 15% higher compared to organizations that don't. This demonstrates the importance of systematically examining both successful and unsuccessful deals to extract meaningful insights, thereby allowing for continuous improvement. It also reinforces the value of taking the time to conduct robust win-loss analyses, which are sometimes overlooked or deemed too time-consuming in the daily grind of a sales organization.
Another interesting observation is that the insights derived from historical data can uncover previously unseen trends or patterns. For instance, a particular product might unexpectedly perform better in a specific region for reasons that aren't immediately obvious. This understanding allows sales teams to strategically focus on these niche markets, maximizing potential returns and potentially uncovering untapped growth opportunities. However, this also raises the question of if the identified patterns are coincidental and if future success in those areas is truly predictable.
The impact of historical win data extends to improving collaboration and communication within sales teams. By integrating this data into CRM systems, companies have observed an increase of roughly 30% in collaboration amongst sales reps. When teams have a shared understanding of past successes and challenges within specific territories, it fosters more productive communication and stronger teamwork. While this improved collaboration can lead to significant benefits, it also begs the question if the data is actually leading to improved results or merely facilitating a better understanding and communication between the individuals in a team.
Moreover, there's some evidence suggesting that sales territories organized around historical win rates can improve employee satisfaction. When reps are more likely to succeed within a particular territory, they tend to feel more confident and empowered in their roles. While increased satisfaction is a positive outcome, it's worth noting that this approach might not be suitable for all reps. Some individuals thrive in challenging situations and might be demotivated by a focus on consistently “winning” territories.
Despite these benefits, it's alarming that over 50% of sales organizations fail to effectively leverage their win/loss data. This highlights a substantial gap in the use of available information to inform sales strategies and can potentially contribute to ongoing pipeline leakage. It's important for sales organizations to invest time and resources into developing the skills and infrastructure required to analyze and interpret win/loss data effectively. This isn't just about collecting the data but ensuring that it's transformed into actionable intelligence.
Ultimately, incorporating historical data into territory planning can yield substantial benefits, including shortened sales cycles. Companies utilizing smart territory planning techniques have experienced reductions of up to 20% in deal closing times. This is largely due to the ability of sales teams to focus their efforts on historically successful regions, allowing for a more streamlined and efficient approach. The question here is if this is a valid approach; that is, we need to be cautious of blindly accepting shorter sales cycles at the expense of potentially more lucrative opportunities.
Furthermore, organizations that diligently incorporate historical data into their territory planning processes may see a 15% increase in return on investment (ROI) from their sales operations. This improvement is not just attributed to higher sales outcomes but also to a more effective allocation of resources throughout the business. It's fascinating to see how data-driven decision making can impact various aspects of a business, but we should also ask if this is a durable effect.
In conclusion, while the use of historical win data for territory planning holds promise for enhancing sales effectiveness, it's crucial to acknowledge the potential limitations and biases. It is not a silver bullet. A balanced approach that blends historical insights with market analysis, evolving customer needs, and the judgment of experienced sales professionals is essential to maximizing the benefits of this approach and ensuring the long-term success of sales strategies.
More Posts from :