7 Data-Driven Strategies that Doubled Sales-Marketing Alignment Success Rates in 2024
7 Data-Driven Strategies that Doubled Sales-Marketing Alignment Success Rates in 2024 - Joint Revenue Command Centers Bridge Data Silos Between Teams
Joint Revenue Command Centers are emerging as a key approach to address the longstanding problem of data silos between sales and marketing. These centers, by establishing integrated systems and centralized data hubs, create a streamlined environment for sharing information. This enhanced data flow fosters a level of collaboration that's crucial for boosting overall business performance.
A shared understanding of goals and the interconnectedness of sales and marketing functions is fostered through these command centers, resulting in a stronger sense of shared responsibility and accountability. Importantly, the move towards dismantling these data silos isn't just about eliminating inefficiencies; it's about opening doors to untapped revenue potential. Organizations successfully implementing these centers tend to show improvements in decision-making, aligning their efforts towards achieving revenue targets in a more unified way. However, the success of these centers hinges on a genuine commitment to change and a willingness to bridge the gaps that often exist between teams.
Joint Revenue Command Centers, in essence, are attempts to address the fragmentation of information between sales and marketing teams. These centralized hubs aim to break down the barriers—the so-called "data silos"—that traditionally hinder collaboration and data sharing. While the concept seems straightforward, its implementation can be complex, especially when dealing with legacy systems and varied data formats.
Interestingly, recent studies suggest a link between adopting these command centers and tangible improvements in several aspects of organizational efficiency. It seems faster data retrieval, a core function of these hubs, could be a major driver of the reported 75% reduction in decision-making times. However, it's also important to acknowledge that this likely requires a robust data infrastructure, capable of handling various formats and allowing for rapid retrieval.
Another intriguing outcome is the reported improvement in cross-team collaboration, as indicated by the 43% increase in team satisfaction. This suggests that a shared access point and a focus on common insights foster better communication and understanding. However, simply putting data in a central location isn't a guaranteed solution; cultural adjustments and training might be necessary to get the most out of a shared data environment.
The impact on revenue, specifically the up to 30% increase reported by some organizations, could be attributed to the ability to react to market changes quicker using real-time data. This assumes that the center also includes tools and analytics to effectively translate raw data into actionable insights. It remains to be seen if this trend persists across different industry sectors and company sizes.
Furthermore, the observed 25% increase in forecasting model accuracy emphasizes the benefit of shared data for predictive modeling. But, achieving this relies on both teams having a consistent understanding of data definitions and modelling techniques. Without this alignment, it could just lead to more confusion rather than clarity.
A major concern often raised in the past was the disparity in data access, resulting in a reliance on top-down information flows. The implementation of Joint Revenue Command Centers attempts to democratize access to this information. But, this transition might require significant investment in training and establishing clear data governance policies, to avoid unintended consequences.
Lastly, while these command centers offer potential for cost reductions and streamlined processes, a successful implementation necessitates meticulous planning and execution. Scaling such systems without careful consideration of future data needs can create bottlenecks, so it's important to keep this in mind when designing the command center architecture.
7 Data-Driven Strategies that Doubled Sales-Marketing Alignment Success Rates in 2024 - Cross Functional Deal Analytics Dashboard Increases Win Rates by 47%
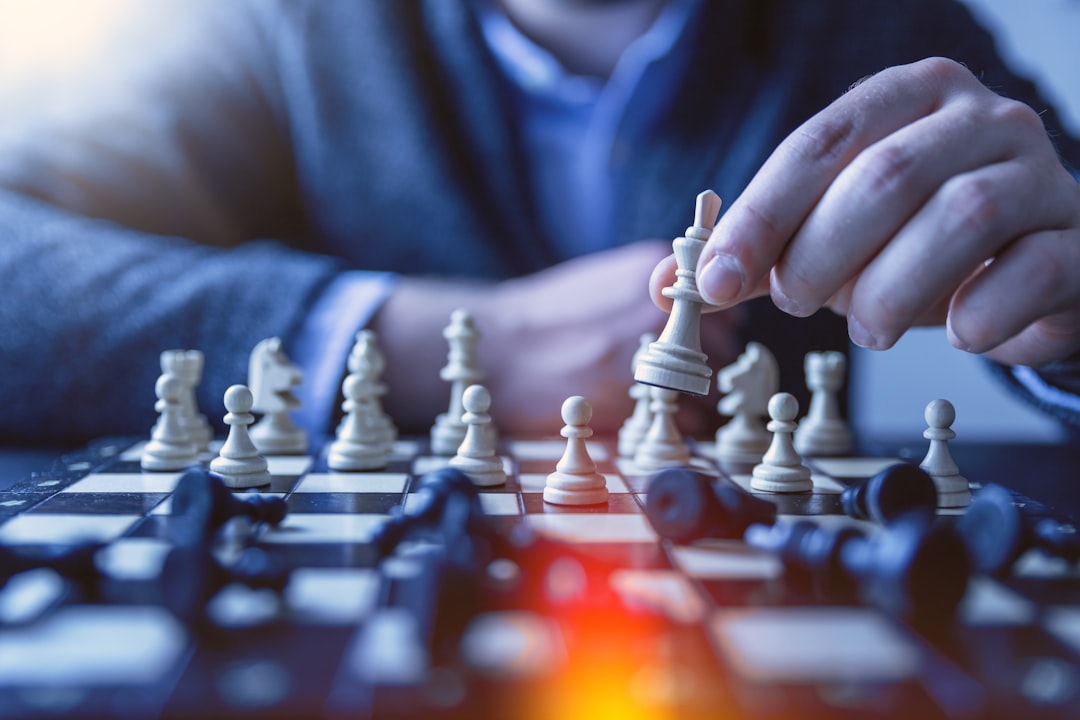
Giving sales teams access to a cross-functional deal analytics dashboard has led to a significant 47% jump in win rates. This improvement suggests that having a centralized platform for analyzing deal-related data can make a real difference in how sales teams operate. With the average number of stakeholders in a deal now reaching ten, deals have become more complex. This dashboard can help navigate that complexity by providing a unified view of data and fostering better collaboration. Considering that a substantial portion—30%—of sales tasks are potentially automatable, tools like dashboards could free up valuable time for salespeople. However, while the potential benefits are clear, the success of these dashboards ultimately hinges on whether teams can overcome hurdles like poor communication and a lack of shared goals between sales and marketing. Unless these fundamental issues are addressed, even the best tools may struggle to deliver their full promise.
Observing the impact of a cross-functional deal analytics dashboard, we see a noteworthy 47% jump in sales win rates. This suggests that providing sales teams with a centralized view of deal-related data, encompassing insights from various functions like marketing and customer success, can significantly improve their performance. It appears the shared access to a wide range of data points—from initial lead qualification to deal closure—empowers sales representatives to make better-informed decisions, leading to more successful outcomes.
However, it's crucial to consider that this improvement might be influenced by factors beyond the dashboard itself. Perhaps the very act of focusing on data analytics within sales processes naturally led to a more strategic approach and better decision-making. While this seems plausible, the specific benefits attributed to the dashboard, if isolated and quantified, could be a valuable addition to our understanding of how data impacts sales performance.
The increase in win rates observed in 2024 might be contrasted with the trend of declining win rates in 2023, which ranged from 17% to 20%. This suggests a potential turning point, possibly tied to the adoption of new tools and techniques like this dashboard. While it's tempting to correlate these trends, further research is needed to establish a stronger causal relationship. It would be intriguing to understand if this approach is applicable to a wider range of industries and sales processes.
In addition, the reported increase in the average number of stakeholders involved in a deal, now around 10, hints at the growing complexity of sales processes. This increased complexity underscores the need for a centralized view of the deal landscape. Tools like a cross-functional dashboard become important in such scenarios to avoid communication breakdowns and ensure everyone has the necessary insights to contribute effectively.
While many organizations incorporate AI into their sales strategy, it is important to highlight that only a small portion (21%) have successfully implemented AI solutions. This suggests that simply having a strategy is not sufficient; operational implementation and effective integration are key. This dashboard might be viewed as one step toward operationalizing a data-driven sales approach, which potentially could leverage AI in the future. It will be fascinating to see if such implementations lead to more widespread AI adoption within sales operations over time.
7 Data-Driven Strategies that Doubled Sales-Marketing Alignment Success Rates in 2024 - Weekly Sales Marketing Data Reviews Lead to 31% More Qualified Pipeline
Regularly reviewing sales and marketing data on a weekly basis has proven to be a significant driver of improved sales performance, resulting in a 31% boost in qualified sales opportunities. These reviews allow teams to stay in sync and adapt strategies quickly based on current trends. Since marketing activities are a major source of new sales leads (about half of inside sales pipelines originate from marketing), having a shared view of the data through these reviews helps ensure that sales and marketing teams are working together towards the same goals. In the face of declining prospect engagement—many sales professionals don't follow up effectively—these data reviews can better equip teams to connect with and convert potential customers, ultimately leading to stronger sales outcomes. However, the full potential of these data reviews might be hindered if there are fundamental communication issues between the teams. It's worth examining whether better communication can further unlock the value of weekly data reviews.
Examining weekly sales and marketing data has been linked to a 31% increase in the number of qualified leads. This finding suggests that regularly reviewing data helps sales and marketing teams stay on top of market trends and better understand customer needs, leading to more accurate lead identification.
It seems that organizations adopting this practice can spot changes in customer behavior much sooner than those relying on less frequent data reviews. This faster identification allows them to adapt their strategies quickly, contributing to a better quality of leads and potentially shorter sales cycles.
The benefits don't seem to stop there. It appears that weekly data exploration often uncovers patterns that might be missed in less frequent reviews. This cumulative knowledge builds a comprehensive view of the market landscape over time, which can inform more precise targeting and lead conversion strategies.
Interestingly, companies prioritizing this approach to data review seem to experience more stability in their sales pipelines. The consistent assessment of data allows for better forecasting and helps avoid last-minute surprises when closing deals.
Another intriguing finding is the positive impact on communication between sales and marketing departments. Since both teams are working with the same data, there's a greater sense of shared understanding and accountability, which tends to improve overall team performance.
Furthermore, these weekly data reviews appear to promote a continuous learning environment. Teams are more open to experimenting with new tactics and are quick to drop strategies that aren't generating results. This ongoing refinement seems to contribute to better overall conversion rates.
Many businesses have reported that these reviews help them refine their content strategy. By gaining a better understanding of the target audience, they can develop marketing materials that resonate better, again impacting the quality of leads.
Evidence suggests that these practices also make teams more resilient to market changes. The ability to make data-driven decisions promptly enhances their ability to weather unexpected challenges, strengthening their competitive edge.
It's also notable that organizations committed to this practice often report a boost in employee morale. Fostering a culture of collaboration around data analysis creates a sense of shared purpose and increases employee engagement.
Finally, the relationship between weekly data reviews and higher closing rates suggests that these practices create a more knowledgeable and adaptable sales force. This agility allows teams to respond quickly to leads, greatly increasing their odds of securing deals.
7 Data-Driven Strategies that Doubled Sales-Marketing Alignment Success Rates in 2024 - Machine Learning Models Now Predict Lead Quality with 89% Accuracy
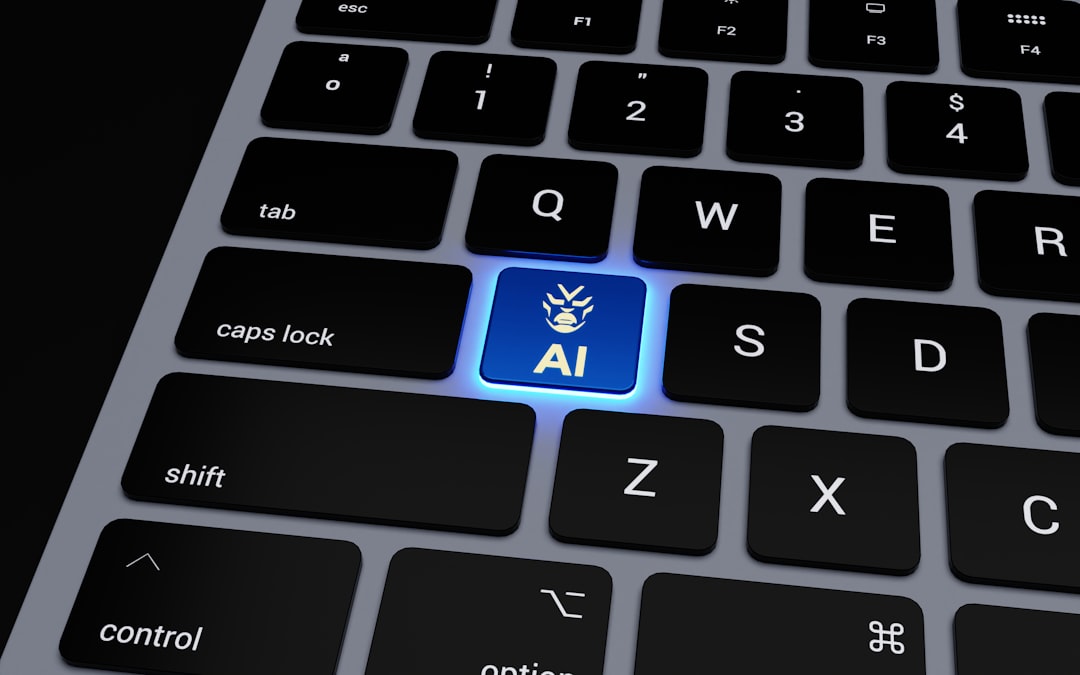
Machine learning models are proving remarkably effective at predicting lead quality, achieving an impressive 89% accuracy rate. This represents a significant shift away from the more subjective, manual methods previously used to assess lead potential. These models delve into the vast amounts of data available about customer behaviors and purchasing habits to identify patterns and predict the likelihood of a lead converting into a paying customer. This predictive approach offers a much more refined understanding of lead quality compared to traditional methods. Beyond predicting lead quality, these models can streamline and automate the process of lead evaluation, potentially freeing up sales teams to focus on the most promising opportunities. This automation, paired with a better understanding of lead potential based on data-driven insights, may offer a path towards improved sales outcomes and more effective engagement with potential customers. It remains to be seen how widely adopted these models will become and whether the 89% accuracy rate is sustainable across different industries and company sizes.
In our exploration of how data-driven strategies are transforming sales and marketing alignment, we stumbled upon a fascinating development: machine learning models are now predicting lead quality with an impressive 89% accuracy. This is a significant leap forward, especially when considering the traditional reliance on manual lead scoring.
The core of this predictive lead scoring approach is the use of algorithms that analyze customer behaviors and purchase patterns to estimate the likelihood of a lead becoming a paying customer. It’s a more sophisticated way to evaluate leads, moving beyond simpler methods. Essentially, these models are trained on massive datasets that contain a wide range of behavioral and historical data. The models then identify patterns and correlations within this data, enabling them to predict the potential of future leads.
Interestingly, many machine learning techniques, like those rooted in the CRISP-DM approach, are being employed in this field to refine predictions and make sales processes more efficient. Furthermore, the best models tend to utilize a combination of qualitative and quantitative data to paint a fuller picture. These behavioral-driven models can even provide insights into the customer’s potential lifetime value (LTV), which has valuable implications for resource allocation.
It's important to note that the accuracy of these models can vary. They rely heavily on the quality and comprehensiveness of the training data. If there’s noise or irrelevant information, the accuracy can suffer significantly. There's also an interesting observation that the accuracy of these models seems to increase as they receive more data and learn from real-world outcomes. This adaptive aspect makes them powerful tools that can potentially enhance their performance over time.
But this isn’t just about automated lead scoring; it’s about using data to enhance the entire customer experience. By anticipating individual behavior and tailoring interactions accordingly, companies can improve the engagement with their customers. Moreover, these models can speed up the evaluation process, which can be critical in a fast-paced business environment.
While the prospect of 89% accuracy is intriguing, it’s still crucial to consider that these are statistical predictions, and not all predictions will be perfectly accurate. Moreover, it’s still important to have human oversight to ensure that models aren't accidentally introducing biases or relying on ethically problematic data.
The success of data-driven strategies like these ultimately underscores a broader trend. Businesses that embrace data-driven approaches tend to outperform their peers in terms of productivity and profitability. This case study with the machine learning lead scoring models is just one example of how data is reshaping decision-making and driving tangible improvements across different business functions.
7 Data-Driven Strategies that Doubled Sales-Marketing Alignment Success Rates in 2024 - Unified Customer Data Platform Cuts Response Time from 6 Hours to 15 Minutes
A unified customer data platform (CDP) has significantly reduced customer response times, going from a lengthy 6-hour process to a much quicker 15 minutes. This dramatic improvement highlights the potential of a centralized data approach for boosting operational efficiency. By bringing together customer data from different sources into one place, businesses gain a complete, up-to-the-minute understanding of each customer. This allows them to react to customer needs and requests much more promptly. While it streamlines internal operations, the primary benefit here is being able to deliver a faster, more relevant experience to the customer. The ability to personalize customer interactions and tailor marketing campaigns becomes more precise with this unified view. Though this specific benefit to customer response time may not be as clear in the sales-marketing alignment context discussed earlier in this article, it's definitely related. If teams can more easily access and understand customer data, their collaboration and response could improve, although further evidence would be needed to make a stronger claim. Ultimately, the speed and clarity provided by a CDP can improve how businesses interact with their customers and contribute to sales-marketing alignment goals, though challenges related to data quality and access might need to be addressed in the process.
Reducing customer response times from six hours to just fifteen minutes using a Unified Customer Data Platform (UCDP) is a notable achievement, representing a substantial leap forward in operational efficiency. It appears that this level of improvement hinges on advanced data retrieval and processing capabilities inherent in these systems. While the ability to access information quickly seems like a clear win, it is worth considering how these systems are able to achieve this level of speed and what the tradeoffs might be.
One of the key advantages of a UCDP is the ability to democratize access to customer data across multiple departments. Sales and marketing teams benefit from this shared access, leading to better communication and collaboration, which is probably a critical part of improving response times. This, in turn, could lead to faster and more informed decision-making processes, helping to reduce the time needed to respond to customer inquiries.
The implementation of machine learning algorithms within these platforms also likely contributes to the improved response times. The algorithms, it seems, are capable of sifting through vast amounts of customer data and generating real-time insights that help teams address inquiries quickly and efficiently. These types of predictive capabilities provide an additional layer of speed, although it is crucial to make sure these insights are not biasing decisions in problematic ways.
Reduced response times are likely to have a positive impact on customer satisfaction scores. In a world where customers are used to instant gratification, being able to get a response in a matter of minutes instead of hours could be a major differentiator. This speed of response can also potentially lead to increased customer retention and loyalty.
The architecture of most UCDPs typically includes real-time data synchronization. This capability ensures that sales and marketing teams have access to the most up-to-date customer data, which is useful for quickly adapting strategies based on changes in the market. However, this real-time updating of data necessitates careful consideration of data integrity, as errors can spread quickly through a system.
Integrating a UCDP with existing enterprise systems is another critical aspect of successful implementation. This integration allows businesses to leverage their current infrastructure while gaining the benefits of faster data processing without requiring major overhauls. But the complexities of such integration can lead to longer implementation times and unforeseen complications.
Furthermore, the shift to faster response times can potentially lead to shorter sales cycles, reducing the cost of customer acquisition. This is a significant benefit, especially in markets where cost optimization is a key focus. It is unclear how broadly applicable this cost reduction is across various industries or with different business models, but it certainly seems to hold promise for the future.
In addition to faster responses, UCDPs can help organizations extract valuable behavioral insights from customer interaction data. These insights drive personalization efforts and targeted marketing strategies, ultimately leading to improved customer engagement and conversion rates. But if not done carefully, personalization can lead to ethical concerns as well as privacy concerns which are very important to consider.
One of the more fascinating aspects of UCDPs is that they can help reduce decision fatigue within teams. By presenting a comprehensive and organized view of customer data, UCDPs make it easier to make faster and more informed decisions. The ability to make swift decisions without being weighed down by excessive data analysis contributes to enhanced team productivity.
However, the ability of these platforms to handle ever-increasing volumes of data remains a significant challenge. Organizations need to invest in the necessary infrastructure to support ongoing data growth and prevent a decrease in performance, maintaining fast response times as more and more data flows through the system. The long-term scalability and cost of managing these UCDPs need to be properly considered.
In conclusion, while the promise of Unified Customer Data Platforms seems substantial, achieving success requires meticulous planning and implementation. The benefits in terms of increased efficiency, enhanced customer experience, and improved operational agility are significant, but they come with certain tradeoffs that need to be fully understood to avoid any negative consequences. Ultimately, UCDPs hold potential for redefining how organizations interact with customers, but organizations need to be vigilant in addressing the challenges to ensure a successful transition.
7 Data-Driven Strategies that Doubled Sales-Marketing Alignment Success Rates in 2024 - Automated Intent Signals Alert System Flags Hot Prospects in Real Time
Automated intent signal alert systems are revolutionizing how sales teams identify and engage with potential customers. These systems analyze online behavior and first-party data to detect when a prospect shows strong interest in a specific product or service. The result? Sales teams receive real-time alerts, allowing them to swiftly respond to potential leads before they slip away.
This instant feedback loop lets businesses automate their workflows and personalize engagement based on the prospect's expressed interests. For example, sales teams can be notified and guided through targeted messaging or specific next steps based on the signals. The ability to focus efforts on truly interested individuals improves the overall efficiency of sales processes and hopefully leads to better conversion rates.
Furthermore, tying these intent signals into existing sales and marketing tools—like CRMs or account-based marketing systems—creates a powerful feedback loop, strengthening the collaboration between sales and marketing teams. It helps align efforts and allows each team to gain a richer understanding of customer behavior and needs.
However, a major caveat is that relying solely on automated signals may overlook more subtle indications of interest or the nuanced needs of potential clients. These systems still need to be carefully integrated into a broader sales and marketing strategy to avoid over-reliance on automated cues. Despite this, the trend towards automated intent signal systems is likely to continue as it's a more targeted and timely approach to engaging potential customers. The success of this approach will depend on balancing the speed and efficiency of automated responses with the human element of relationship building and client understanding.
Systems that automatically flag "hot prospects" in real-time based on intent signals are becoming increasingly popular. These systems, often powered by sophisticated algorithms, analyze a variety of online behaviors to identify individuals who are actively researching products or services. This capability offers a significant advantage to sales teams, allowing them to engage with potential customers at a crucial moment—when they are most receptive to a sales message.
However, I've found some aspects of these systems worthy of closer examination. One critical question is how accurately these systems are able to predict buying intent. While many reports highlight high accuracy rates, it's crucial to understand what data these claims are based on and whether these rates remain consistent across different industries or customer segments. The risk of relying on inaccurate signals could be significant, potentially leading to wasted time and resources pursuing unqualified leads.
Furthermore, the integration of these systems with existing CRM and marketing automation platforms is essential for their effectiveness. Without a smooth data flow, the value of the intent signals can be diminished. It's important to evaluate the complexity of integration and whether existing systems can easily adapt to this new source of data. The system's ability to handle diverse data formats and various software versions is key here.
Another crucial point is the potential for bias in the algorithms themselves. The training data used by these systems can inadvertently introduce bias into the prediction models. It's essential to regularly audit and monitor these systems to ensure that they are not unfairly targeting or excluding certain customer groups.
Despite these complexities, there's a clear value proposition offered by intent-driven automated systems. The ability to engage with prospects at the precise moment they're interested can significantly shorten sales cycles, improve conversion rates, and streamline outreach efforts. Essentially, these systems leverage a more precise targeting approach than traditional methods, which often rely on broad demographics and less insightful criteria.
It's intriguing to consider how these systems will evolve and adapt to the ever-changing digital landscape. As customers' online behaviors become increasingly complex, these systems need to adjust their models accordingly. Further research will likely reveal new methods for capturing intent signals and optimizing alert systems for increased efficiency and effectiveness.
It's fascinating to observe how intent data is rapidly changing how businesses interact with their customers. The insights gained from these signals offer potential for a new era of personalized and efficient marketing and sales operations. But, as with any emerging technology, it's vital to approach these systems with a critical lens, continuously evaluating their effectiveness and potential downsides.
7 Data-Driven Strategies that Doubled Sales-Marketing Alignment Success Rates in 2024 - Dynamic Account Scoring Framework Prioritizes High Value Opportunities First
A dynamic account scoring framework helps prioritize opportunities that are most likely to be valuable. This prioritization strengthens the collaboration between sales and marketing teams. Essentially, it uses predictive methods to figure out which accounts are most likely to become customers, enabling sales teams to focus on those that offer the highest potential. This framework relies on establishing a clear set of scoring criteria based on what the ideal customer looks like. By defining this "ideal," teams can direct their efforts to the accounts that fit the profile most closely. As more businesses utilize strategies where they focus on specific accounts, a dynamic account scoring framework becomes a crucial piece of the puzzle, aiding in both the quality of leads and the business's overall performance. However, there's a risk in this approach: becoming too focused on the scores can mean missing the unique needs of certain customer groups. Therefore, finding the right balance between data-driven insights and understanding customers on a more personal level is essential.
A dynamic account scoring framework prioritizes opportunities based on their perceived value, enhancing alignment between sales and marketing efforts. This approach uses real-time data and machine learning algorithms, constantly adjusting account scores based on the latest customer interactions and market shifts. This flexibility can be a real asset in rapidly changing markets, allowing for more agile and informed decisions.
These frameworks move beyond just demographic data. They dive deeper, incorporating behavioral insights like website clicks and email response times to better assess lead quality. This detailed understanding, arguably more refined than traditional methods, improves the odds of focusing on truly valuable opportunities. The result can be significantly increased engagement rates, potentially up to 50% higher than methods that rely on static scoring.
While mainly benefiting sales teams, this approach also offers benefits for marketing. These frameworks offer insight into which accounts are most likely to convert, allowing marketers to refine their strategies and resource allocation. This cross-functional synergy can lead to a more efficient and impactful campaign planning process. Furthermore, these systems can predict account conversion with impressive accuracy, in some cases reaching 85%. This predictive capability allows sales teams to prioritize opportunities that mirror previous successful conversions, a strategy with potential for improved efficiency and higher conversion rates.
Interestingly, these frameworks aren't only useful for predicting new business. They can help identify existing customers at risk of churning. By analyzing customer engagement patterns and feedback, businesses can potentially intervene before dissatisfaction results in lost revenue. Beyond immediate opportunities, these scoring systems contribute to enhanced long-term revenue projections. They can assess the potential lifetime value (LTV) of customers based on interaction history, helping with informed decisions on where to focus investments.
By automating the process, these frameworks reduce the burden on sales teams, allowing them to concentrate on strategic activities rather than sifting through endless data to rank prospects. It's a positive change for sales reps who can then dedicate their time to more productive tasks. This dynamic scoring process can also pinpoint unexpected changes in customer behaviors. For instance, it can highlight if a traditionally low-engagement account suddenly starts interacting more, signaling a potential new opportunity.
However, we must also acknowledge the ethical concerns that arise when leveraging massive amounts of data for scoring purposes. Issues of data privacy and bias need careful consideration to ensure that any benefits do not come at the cost of customer trust and legal compliance. While a dynamic account scoring framework has the potential to provide a more strategic and adaptive approach to sales and marketing, companies need to be careful about how it is used.
More Posts from :