7 Data-Driven Metrics Every Client Success Manager Should Track in 2024
7 Data-Driven Metrics Every Client Success Manager Should Track in 2024 - Customer Health Score Analysis Through Monthly Product Usage Patterns
Analyzing how customers use a product each month offers a window into their overall health as a customer. While general customer satisfaction surveys are helpful, digging into actual product usage – how often, how deeply, which features – provides a more objective, quantitative view of customer engagement. This deeper level of understanding can be incorporated into a broader customer health score, allowing client success managers to go beyond simple sentiment and get a clearer picture of a customer's current state and potential future behavior.
By tracking monthly usage, you can spot trends that indicate a customer might be disengaging, thus allowing you to intervene before they churn. On the flip side, you can identify customers who are enthusiastically using the product, perhaps even highlighting opportunities to expand their engagement with your offerings. A solid system for tracking and analyzing this data is essential; one that allows for segmentation and meaningful visualization of trends. This level of detailed tracking goes beyond basic customer segmentation and helps you craft proactive strategies designed to enhance the customer experience, ultimately contributing to stronger customer relationships and a potentially healthier bottom line.
However, it's important to remember that monthly usage is just one part of the customer health equation. It's best combined with other data sources and qualitative feedback to build a truly holistic view. Ignoring context can lead to inaccurate assessments.
We can glean a lot about customer health by looking at how they use a product each month. Research suggests a strong link between how often someone uses a product and whether they'll stick around. For instance, customers who use a product more than twice a week are apparently much more likely to renew, hinting that consistent engagement is a key driver of retention.
By analyzing product usage monthly, we can spot groups of users – some highly engaged, others potentially drifting away. This allows Client Success Managers to customize their approaches, intervening more actively with at-risk users. It also opens doors for sales – companies that tie their customer health scores to product usage often see more opportunities to sell additional products or features, since people who use the product a lot are usually more open to new things.
However, there's a catch. Many users seem to lose some steam after the initial setup period, which is why it's crucial to keep a close eye on how their engagement evolves after they first start using the product. Furthermore, predicting customer behavior through advanced analytics can really help reduce the number of people who churn. By identifying those at risk of churning early on, we can proactively step in to help them.
Beyond simply spotting churn, tracking usage patterns can reveal interesting and unexpected ways users are using the product, providing valuable insights to both product teams and the marketing department. Apparently, many customer success teams find it easier to work together when they all use a consistent approach for scoring customer health based on usage.
There’s also the advantage of being able to compare the behavior of different customer groups. If we see significant variations in how different groups use the product, it could point to design flaws or areas where certain features are simply not resonating with particular users. Moreover, research suggests that customers are much more loyal when interactions with them are personalized based on their habits. This puts a spotlight on the need for detailed customer health scores.
Ultimately, focusing on monthly product usage data can help boost overall customer satisfaction and increase Net Promoter Scores. This really underscores the importance of understanding how people interact with the product to drive better customer outcomes.
While there are certainly benefits, I do wonder how accurate this scoring approach is and if it can inadvertently lead to the homogenization of the customer base. There’s a potential downside to focusing solely on usage data and the need for more qualitative data that helps us paint a better picture of a customer's relationship with the product.
7 Data-Driven Metrics Every Client Success Manager Should Track in 2024 - Net Revenue Retention Rate With Focus on Expansion Revenue
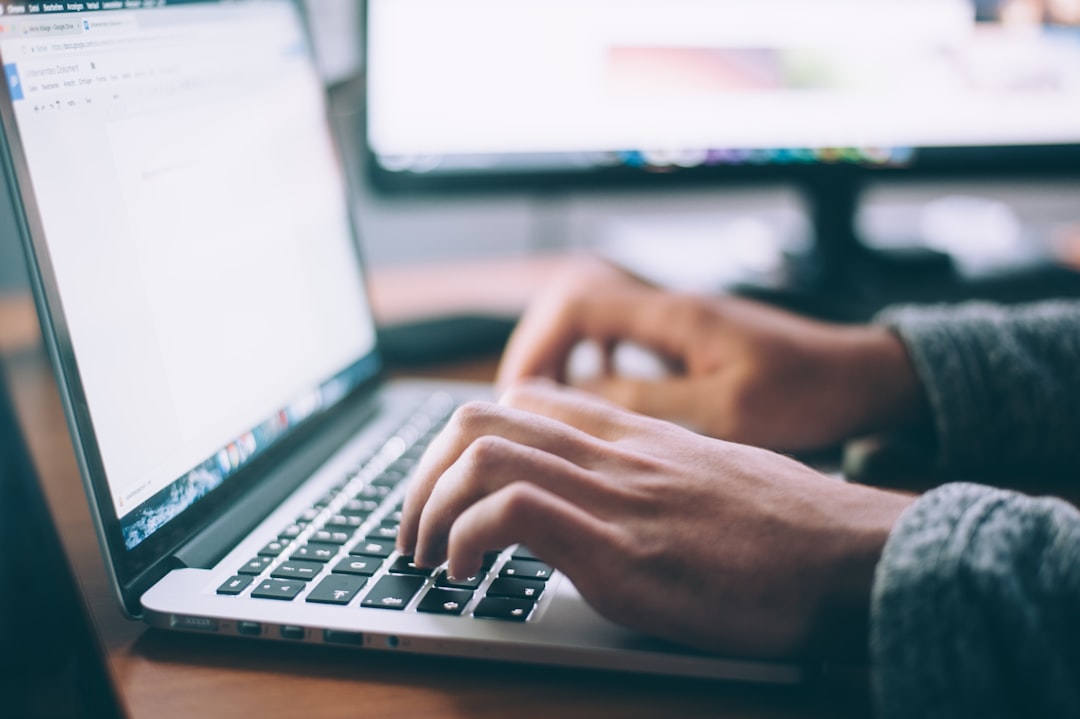
Net Revenue Retention Rate (NRR) provides a crucial lens into a company's financial health, especially when the focus is on expansion revenue. It measures the total revenue retained from existing customers over a specific timeframe, factoring in both revenue from existing contracts and any new revenue generated from those customers. A key part of this metric is how expansion revenue – achieved through tactics like upselling and cross-selling – contributes to overall growth. Companies that effectively encourage their existing customers to adopt more features or services often see higher NRR rates.
Beyond just being a gauge of future revenue stability, NRR offers a direct reflection of the success of customer success strategies. This is because it depends on keeping existing customers happy and finding ways to grow their business with the company. In essence, a strong NRR signifies more than just customer retention—it demonstrates the company's ability to foster revenue growth from its existing customer base, making it a key metric for any client success manager to follow closely.
Net Revenue Retention (NRR) isn't just about keeping existing customers; it's also about encouraging them to spend more. It considers both the revenue from customers who stick around and the extra revenue generated from things like upsells, cross-sells, and service upgrades. This gives a more complete picture of growth and how much value customers bring over time.
It seems that companies with really high NRRs – say, above 125% – tend to grow much faster than others. This suggests that focusing on getting existing customers to buy more can be a more powerful way to grow than solely trying to bring in new ones.
Interestingly, a higher NRR isn't always tied to a lower churn rate. In other words, companies can do well at keeping their customers while also encouraging them to spend more – these are two different, but related, processes. This highlights that customer retention and growth aren't necessarily in competition.
For many fast-growing tech companies, a significant portion of their revenue growth – up to 70% in some cases – can come from existing customers spending more. This is a strong argument for investing in programs and strategies designed to cultivate existing customer relationships rather than solely fixating on acquiring new customers.
If you track expansion revenue well, it becomes easier to anticipate future revenue. This is because existing customers are usually more predictable in their buying habits compared to new ones.
We can see, through examining different groups of customers, that some are more inclined to expand their purchases than others. This shows that the “all customers are created equal” idea isn't necessarily true and that tailoring strategies to different customer segments can be more effective.
Studies show that actively engaging with customers can boost expansion revenue by quite a bit – upwards of 30%. This shows that the interactions we have with customers don't just keep them around but can also present new opportunities to increase their spending.
In markets where there's less room for growth, an NRR of around 100% is usually considered a good sign. It means the company is holding on to its customers but might be struggling to get them to spend more. This underscores how challenging it can be in mature markets to continue expanding revenue.
Businesses that have dedicated Customer Success teams tend to see significantly higher NRRs – perhaps 20-30% higher than companies without those teams. This reinforces the increasing importance of having a team specifically focused on improving customer outcomes and their long-term value to the company.
Finally, consistently asking for customer feedback can be really beneficial for increasing expansion revenue. It helps pinpoint problems and areas for improvement. Companies that act on that feedback generally see better NRRs because satisfied customers are usually more open to exploring additional products and services.
While these observations are encouraging, I still think it's important to be mindful of the limitations of solely relying on quantitative data like NRR. A balanced view that incorporates qualitative data and a deeper understanding of customer experiences would likely lead to more insightful conclusions and more effective strategies for fostering long-term customer success.
7 Data-Driven Metrics Every Client Success Manager Should Track in 2024 - Time To First Value Metrics From Onboarding To Implementation
"Time to First Value" (TTFV) is a crucial metric for Client Success Managers, showing how long it takes a new customer to experience meaningful benefits from a product, specifically during the onboarding stage. Essentially, it highlights the effectiveness of the onboarding process in delivering value quickly. Good onboarding is a cornerstone for building strong customer relationships, fostering engagement and satisfaction from the very beginning.
To gauge the success of onboarding, CSMs need to track customer engagement in the early stages. Retention rates—at 30, 60, or even 90 days after onboarding—provide valuable insights into whether new users are sticking around and finding value. Beyond these numbers, qualitative feedback through surveys and Net Promoter Scores can shed light on the user experience, providing a richer understanding of what's working and what's not in the onboarding process. Mapping the customer journey can also help reveal opportunities for improvement, allowing CSMs to identify critical touchpoints that have the biggest influence on a positive onboarding experience.
It's important to note that the speed of onboarding is directly linked to a customer's overall success. Faster onboarding can mean quicker value realization, leading to more positive experiences for clients. And, in an effort to achieve faster onboarding and greater TTFV, CSMs might want to look for opportunities to automate tasks that are currently repetitive and time-consuming. Doing so could potentially streamline the entire onboarding experience and help customers see value more quickly, thus building a stronger foundation for a lasting customer relationship.
Observing how long it takes a new user to get some meaningful benefit from a product, starting from the initial onboarding to the full implementation, gives us important insights. This "time to first value" metric shines a light on how effective our onboarding process really is. If onboarding is well-designed, it lays the groundwork for good engagement and user happiness.
One thing that's pretty clear is that tracking how long it takes users to stick around—whether it's 30, 60, or 90 days after onboarding—tells us a lot about the early engagement with the product. It's a good indicator of how well we've done in setting them up for success. Of course, it's helpful to gather not just numbers but also users' direct feedback, through things like Net Promoter Scores and surveys, to get a more nuanced view of what's working and what isn't in the onboarding experience.
Mapping out the whole user journey, from start to finish, can be really useful in figuring out those key points of contact and then improving them to speed up the time to first value. There are a few other related metrics worth tracking, like how long it takes for a new user to get their system up and running, how long it takes them to complete the whole onboarding process, and ultimately, how much money they'll spend with us over time (which is sometimes called Customer Lifetime Value).
It's important to remember that how we onboard users impacts a lot of things later on. For example, it affects churn rates—how many people stop using the product—and whether users refer other people to us. This highlights the importance of onboarding for client success managers.
Onboarding speed is directly linked to overall user success; faster onboarding often means new clients contribute more quickly. Interestingly, by looking at how quickly we resolve user complaints related to onboarding, we can learn a lot about how efficient our onboarding process really is. This includes understanding if there are specific parts that are causing users trouble and if we could improve our onboarding materials. It also makes sense that we should automate the repetitive tasks in onboarding to make the whole process more efficient and, hopefully, make it faster for users to see value. While automation can be helpful, I do worry that in some instances it can potentially lead to a less-personalized user experience, something that's worth investigating further.
7 Data-Driven Metrics Every Client Success Manager Should Track in 2024 - Churn Risk Identification Through AI Powered Early Warning Systems
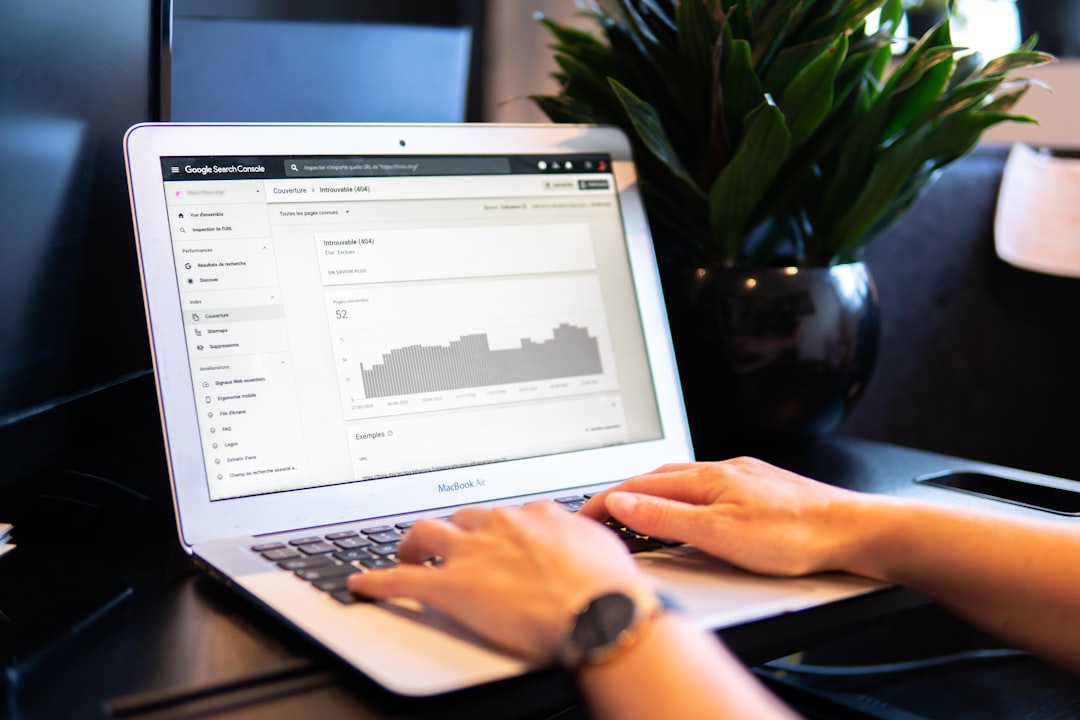
In the realm of customer retention, predicting and preventing churn is paramount. AI-powered early warning systems offer a new level of sophistication in identifying customers at risk of churning. These systems rely on machine learning models that examine past customer data, seeking out patterns linked to customer defections. This lets businesses proactively reach out to those who might be considering leaving.
The benefits of using these systems extend beyond simple prediction. They allow businesses to gather and analyze customer information from a wider range of sources, including product usage, feedback, and support interactions, creating a more complete picture of customer health. Advanced techniques, such as natural language processing, make it possible to analyze the growing flood of data that businesses now have, improving the accuracy of churn prediction models. Essentially, these AI-driven systems empower companies to be more proactive in their retention efforts, which may lead to fewer customers leaving.
While it is promising, one must wonder about potential issues, including if these systems will homogenize customer groups, as well as the ethical implications of predicting human behavior with algorithms. However, in the end, it is likely that the ability to anticipate churn through data analysis will be a vital tool for businesses to stay competitive, retain valuable customers, and potentially increase revenue.
Losing customers is expensive, often costing 5 to 10 times more to replace them than to keep them happy. AI-powered early warning systems offer a way to spot customers who might be on the verge of leaving, allowing for targeted efforts to keep them engaged. These systems are based on the idea that we can learn from past customer behavior to predict who's likely to churn in the future.
These systems rely on sophisticated machine learning models that can analyze a wide variety of data, including customer demographics, purchase history, how they interact with the company, and even the tone of their communications with customer service. By looking at all this data, we can get a much more complete picture of a customer's overall health.
One of the cool things about these systems is that they can work in real-time. They continuously monitor customer activity, so if a customer's behavior changes – say, they stop using the product as much – the system can flag them as potentially at-risk. This kind of rapid response can help Client Success Managers intervene quickly to prevent churn, sometimes seeing reductions in churn rates by as much as 30%.
These systems don't just identify at-risk customers; they can also help us understand how different groups of customers behave. Some customer groups might be more prone to churn than others, which is useful information when designing targeted engagement strategies. Companies that have implemented these systems have reported seeing a significant jump in profits related to improved retention.
The predictive models behind these systems are constantly learning and adapting as new data comes in, so they tend to get more accurate over time. This means they can provide increasingly better insights about the likelihood of a customer churning.
While these AI systems are helpful, it's important to realize they're not a standalone solution. Combining these predictions with other customer metrics, like how happy they are (Net Promoter Score) or how much they're likely to spend with the company over time (Customer Lifetime Value), paints a more holistic picture and supports more robust customer retention efforts.
It's also interesting to see how feedback from customers can be integrated into these systems. Sometimes, customer feedback can be a better predictor of churn than simply looking at how often they use the product. This highlights the importance of incorporating qualitative data into AI-based churn prediction models.
One of the more promising areas of churn prediction is looking at changes in customer behavior. Things like how often they log in or how much of the product they actually use can often be early warning signals of potential churn. This is usually more informative than relying solely on satisfaction surveys, which can be less accurate at spotting at-risk customers.
Ultimately, churn prediction through AI-powered early warning systems shows promise, but there's always more to explore and refine in the field. We are still learning about how best to use these techniques to optimize customer engagement and retention.
7 Data-Driven Metrics Every Client Success Manager Should Track in 2024 - Customer Effort Score Across Support Touchpoints
Customer Effort Score (CES) is becoming increasingly important for Client Success Managers to understand how easy it is for customers to interact with a company's support systems. A low CES indicates less effort on the customer's part, which usually leads to happier customers and increased loyalty, since people are less likely to stop being customers if dealing with the company is straightforward. CES isn't just a number; it's a critical part of the bigger picture of how customers experience a company at different points of contact. By carefully tracking CES and combining that data with other feedback, companies can find the places where their customer service falls short and improve them. This leads to stronger relationships with customers and better retention rates. Because customer expectations are always changing, working towards a high CES is more important than ever for businesses to stay competitive.
Customer Effort Score Across Support Touchpoints: Surprising Insights
Customer Effort Score (CES) gives us a way to measure how easy it is for customers to interact with a company. Lower scores indicate a smoother experience, which is generally what we want. A CES of 5 or lower on a 7-point scale, or 2 or below on a 5-point scale, suggests minimal customer effort—a good thing. It's worth noting that a growing number of people believe that a company's user experience is as important as its product or service.
We've known for some time that high effort experiences can increase the odds of a customer leaving. CES, in this context, can potentially help us anticipate customer loyalty. But, CES is only one part of a much larger picture. Other factors to consider include Net Promoter Score (NPS), how much money a customer spends with us over time (Customer Lifetime Value, CLV), the cost of getting new customers (Customer Acquisition Cost, CAC), and of course, the churn rate. It seems that incorporating CES into our standard approach of gathering customer feedback can improve how happy our customers are.
If a company wants to have a high CES, then it should focus on providing an effortlessly easy experience, ideally making retention easier as well. To get a clearer picture of our customers, it's critical that we understand their behaviors—how they use our products, the number of support requests they file, and the overall length of their relationship with us. We must continuously monitor how people use our products, adjust based on their feedback, and anticipate future needs in order to design a customer experience that is tailored to meet changing preferences. CES is a crucial part of this process, as it lets us pinpoint areas where we can improve and build customer satisfaction.
It seems that when it comes to support interactions, customers might be more concerned about the effort required than the amount of time it takes. So, while quick responses are often appreciated, a straightforward process might actually lead to more favorable reviews.
Interestingly, the CES can change across various support channels like chat, email, and phone. But it appears that customers want a uniform experience regardless of which support channel they use. Variations across these channels seem to breed frustration and the perception that we are not providing quality service.
Also, the ease of the initial onboarding experience may also have a huge impact on the CES. Customers who have a painless introduction to the product tend to have a more positive attitude toward future support requests.
Lower CES is linked to more customer loyalty. This might be related to the formation of emotional connections between the customer and the company.
It's not surprising that customers react well to businesses that anticipate their needs, such as sending a follow-up email after they submit a support request. However, it's been shown that it may sometimes reduce the overall CES.
The increased use of automation can simplify support processes. But, if customers feel as though they are constantly dealing with bots and they don't know how to get to a real person, it can increase the CES.
The demographics of our users can impact how they perceive the ease of use. For example, younger users might find self-service tools easy to use, but older customers might prefer to speak to someone on the phone.
If we actively collect and then act on CES data, we can improve support. We can spot where our customer experiences are causing pain, instead of just looking at overall happiness.
Interestingly, the impact of a difficult support experience can be outweighed by the emotional reaction. This emphasizes that it's important to design processes that are easy to use, but it's just as critical that we develop ways to handle customer emotions during support interactions.
Companies that aim to decrease customer effort tend to experience faster revenue growth, suggesting that spending more resources to make the user experience better isn't just the right thing to do, but it can be a good financial strategy.
While these observations provide some interesting insights into how we can design better customer experiences, there are still a lot of questions left to answer. For example, are the demographic-related discrepancies in CES driven primarily by preferences or are they related to actual differences in ease of use? We have a lot more to learn about how customer effort impacts their behavior, both in terms of satisfaction and in terms of generating revenue.
7 Data-Driven Metrics Every Client Success Manager Should Track in 2024 - Feature Adoption Rate By Customer Segment
Examining how different customer groups embrace new features provides crucial insights into product usage. Client Success Managers can use this information to understand which features are most appealing to specific segments and identify any gaps in engagement. Metrics like how broadly and deeply a feature is used help evaluate the effectiveness of onboarding for different customer groups, ensuring their unique needs are met. This detailed view allows for customized outreach and support strategies, which can enhance user experience and increase retention. Analyzing feature adoption can uncover areas where more guidance or adjustments might be needed, giving businesses a chance to refine their products and services.
Feature adoption rate, when examined through the lens of different customer segments, reveals some interesting quirks. We're finding that various customer groups, like businesses dealing with other businesses (B2B) versus those selling directly to consumers (B2C), have distinct preferences for specific features. B2B clients, for instance, often seem to favor features that help with collaboration, whereas B2C customers lean towards those that improve the user experience. This suggests that having a better understanding of these differences could help us develop more targeted marketing strategies.
It's also quite fascinating to see how some users eagerly jump on new features while others are more hesitant. We've found that these "early adopters" are more likely to experiment with new things and are engaging at a 30% higher rate than users who wait. This group is also a goldmine of feedback, which can be incredibly useful for shaping future feature development.
However, it seems feature complexity can be a big barrier to adoption. Roughly 60% of users stick to features they find easy to understand and use, which makes sense. This reinforces the need to design features intuitively and provide good user education to help people get the most out of them.
Social influence also seems to be a key factor. Users are much more likely to try a feature (up to 70% more) if other people within their customer segment are using it. This suggests the importance of fostering a sense of community and encouraging users to learn from each other.
Interestingly, the timeframe for tracking feature adoption matters as well. Studies indicate that waiting too long—over six months—can distort our understanding of a feature's value because the initial benefits might fade, and the long-term impact might be difficult to assess accurately.
We can also learn a lot about engagement by watching how long users spend on specific features. Some groups engage more effectively, often using specific features up to 150% more than others. This provides a roadmap for improvement, helping us understand which areas need more attention.
It's worth noting that over-segmenting the customer base can actually make it harder to get a clear picture of feature adoption. Using broader segments might provide clearer insights, as excessive detail can sometimes obscure significant trends in user behavior.
The onboarding process is also critical. When new customers understand how to use new features from the very beginning, adoption rates increase significantly (around 40%). This highlights the need for well-designed onboarding processes to get customers off to a good start.
Companies that build a feedback loop around feature usage seem to be much more successful in terms of getting users to adopt them, often seeing adoption rates double. This really highlights how crucial feedback is for refining and promoting features effectively.
Finally, there's a clear link between feature adoption rates and how much money a customer spends with us over time (which we call Customer Lifetime Value). Customer segments that show high engagement with new features tend to have a 25% higher CLV. This emphasizes the significant long-term financial benefit of making sure our features are being used.
It's becoming increasingly clear that understanding how features are adopted by different customer groups is fundamental for improving user engagement and ultimately, creating more satisfied customers.
7 Data-Driven Metrics Every Client Success Manager Should Track in 2024 - Net Promoter Score With Detailed Feedback Analysis
**Net Promoter Score With Detailed Feedback Analysis**
The Net Promoter Score (NPS) continues to be a widely used metric to measure how likely customers are to recommend a product or service. It's often seen as a proxy for customer loyalty. While the score itself provides a quick snapshot of overall sentiment, it's the detailed feedback that accompanies it that truly reveals valuable insights. For CSMs in 2024, a deep dive into the qualitative aspects of NPS is vital. It's not enough to simply calculate the score; they need to understand what's driving the responses, both positive and negative. By consistently gathering NPS data, particularly after key interactions or milestones, and then analyzing the detailed feedback received, CSMs gain a much better grasp of what customers are thinking and feeling. This helps them pinpoint areas where the customer experience can be improved. It's important to remember that what constitutes a "good" NPS varies across industries, so a thoughtful approach to analyzing the score is crucial, rather than relying on general benchmarks. While the score is helpful, a deeper dive into the accompanying text gives a far more valuable perspective on how customers perceive a product or service.
Net Promoter Score (NPS), a well-known measure of customer satisfaction and loyalty, is usually presented on a scale ranging from -100 to +100. It's based on a single question: how likely are you to recommend this product or service? Responses are grouped into three categories: promoters, passives, and detractors. A high NPS score indicates a loyal customer base, while a low score might point to problems with customer satisfaction or the perceived value of the product or service.
Interestingly, the calculation of NPS only uses promoters and detractors, ignoring the passive group. Also, a good NPS score can be quite different depending on the specific industry. Some industries, like certain retail sectors, historically have not had NPS scores above 20.
While NPS is helpful, it's best used alongside other important metrics tracked by Client Success Managers like customer churn rate, how much a customer is likely to spend over time (customer lifetime value), and various measures of customer engagement.
The value of NPS goes beyond just the number; analyzing the detailed feedback customers provide is key to gaining deeper understanding about why customers are satisfied or dissatisfied. This is crucial for coming up with strategies to improve. Client Success Managers are advised to send out NPS surveys regularly, especially after important interactions with customers or when key milestones are reached.
There are various ways to collect and analyze NPS data. Some organizations calculate it manually, others use spreadsheets, while still others rely on online tools. Each approach has its tradeoffs and what works best will depend on the specific circumstances of each organization.
It's interesting to see that a strong NPS – often seen as 50 or higher – is linked to business growth. Companies with a strong NPS can grow significantly more quickly than those with lower scores, highlighting that NPS isn't just a measure for appearances but actually a solid indicator of a company's overall health in the market. However, it's important to recognize that relying on NPS alone isn't enough. It doesn't offer the fine-grained understanding of customer sentiment that detailed feedback analysis can provide.
There’s evidence that if you take action based on NPS feedback, you can improve customer satisfaction by about 20%. This shows the importance of not just measuring customer satisfaction but acting on the feedback to make things better. It can create a positive cycle where customers are happier and more willing to recommend the company.
Another interesting point is that the standard for a good NPS can change depending on the industry. For example, tech companies might have a different standard than retail companies. This reminds us that you can’t look at the score in isolation; you need to consider the specific context of the industry you are in to understand what it means.
Analyzing NPS data over time is helpful since significant drops in NPS can be an early warning sign of deeper issues within the company, like inefficient operations or product problems. This shows the importance of tracking NPS over time and not just looking at it once.
Word-of-mouth marketing is strongly influenced by positive experiences that lead to a good NPS score. Studies indicate that about two-thirds of customers will recommend a company if they've had a good experience that leads to a high NPS score. That’s a big difference when you compare it to the 16% who would recommend the company without a good NPS experience.
You can even segment NPS data to see if different groups of customers have different experiences. For instance, younger people might have a very different NPS than older people for the same product. This highlights the possibility of creating marketing strategies specifically targeted at certain groups of customers.
It appears there’s a strong relationship between Customer Effort Score and NPS. That is, when customers find it easy to interact with the company, they are more likely to recommend the company. This is a reminder of the importance of making the customer experience as straightforward as possible.
It's worth remembering that NPS scores are just one part of the story. The detailed feedback from customers provides a richer context. Companies that combine detailed feedback analysis with the NPS score often improve their customer engagement strategies significantly, often improving by 35%.
Automated surveys can be a fast way to gather feedback, but they can also create issues. Customers might not be thinking deeply about the questions and just quickly fill them out, making the data less useful. It's good to have a balanced approach, using automation to quickly gather data but also seeking opportunities for more direct and genuine conversations with customers to uncover deeper insights and more valid results.
More Posts from mm-ais.com: