7 Critical Components of a Data-Driven ABM Strategy Template for 2025
7 Critical Components of a Data-Driven ABM Strategy Template for 2025 - Real Time Intent Signals From First Party Data Analysis
Understanding the real-time intentions of potential customers, gleaned from your own data, is fundamental to making your account-based marketing (ABM) plans sharper for 2025. By scrutinizing the direct interactions people have with your websites, apps, and email campaigns, you can start to differentiate between those who are seriously considering a purchase and those who are simply browsing. This ability to categorize user actions into different levels of intent – high, medium, or low – provides a more sophisticated understanding of potential buyers. You can tailor your communication and offers based on what they've actually done, whether it's downloading white papers, visiting product pages repeatedly, or engaging with specific content. Further, the power of combining this real-time view of behavior with historical data is crucial. It gives marketers a better capacity to act quickly on changing buyer interests, tweaking campaigns to stay ahead of the curve. Given the often limited success of conventional approaches, focusing on these intent signals holds the potential to make your ABM much more impactful. While simply throwing a wide net might seem easier, the potential for wasted resources and a lack of engagement are very real. A smarter ABM strategy using first party intent signals has a real chance to be more effective.
Examining the behavior of website visitors and app users through first-party data can give us a pretty good idea of what they're interested in right now, which is super useful for focusing our marketing efforts. We can potentially increase our conversion rates by a notable 20-30% by carefully utilizing this real-time "intent" data. This helps us target the right people at the right time, improving the overall effectiveness of our outreach.
It's fascinating to consider how using real-time intent data can significantly reduce the money we spend to acquire new customers, potentially by 10-15%. This makes sense because we're essentially using our existing knowledge about what people are doing to focus on those who already seem interested, making our marketing dollars work harder.
It's also clear that many marketers have observed a big improvement in segmenting our audiences with the use of real-time data analytics—over 70% are seeing positive results. This improved segmentation can lead to more tailored messages and a better experience for potential buyers, which is good for both the customer and the business.
What's really interesting is that using intent signals from first-party data can substantially shrink the sales cycle. It can potentially cut it in half—that's a 50% reduction! That's a huge efficiency gain in managing leads and getting sales closed faster.
However, it's also pretty surprising that so many companies still aren't leveraging this powerful data. It appears that only around 30% are using these sophisticated real-time techniques. There seems to be a lot of untapped potential there.
Applying machine learning to analyze these real-time signals can be a game-changer for recognizing new market patterns. It appears that this approach can be up to three times faster than traditional methods of understanding market trends, giving us a significant edge over competitors.
We also see that tailoring our communications to individual users based on what we know about their interest results in a much higher chance of a positive response. Customers are 50% more receptive when they feel we understand their needs and provide information they find valuable.
Interestingly, there's also a positive impact on the relationship between marketing and sales teams when they're using this real-time data. More than 60% of companies report improved collaboration and shared understanding because of the common insights revealed through data analysis.
With the increased importance of privacy, using first-party data seems to be even more crucial. Companies that focus on this approach are more likely to foster trust with customers. It appears they build up 25% more trust than companies relying on third-party data, which makes sense given the privacy concerns that have been raised recently.
Lastly, the ability to keep an eye on user behavior as it unfolds gives us the power to quickly adjust how we reach out to them. We can immediately react to spikes in interest and nearly double our engagement rates when people are actively looking for what we offer. This sort of dynamic response is difficult to achieve without real-time insight into our audience.
7 Critical Components of a Data-Driven ABM Strategy Template for 2025 - Dynamic Lead Scoring Models With Machine Learning Integration
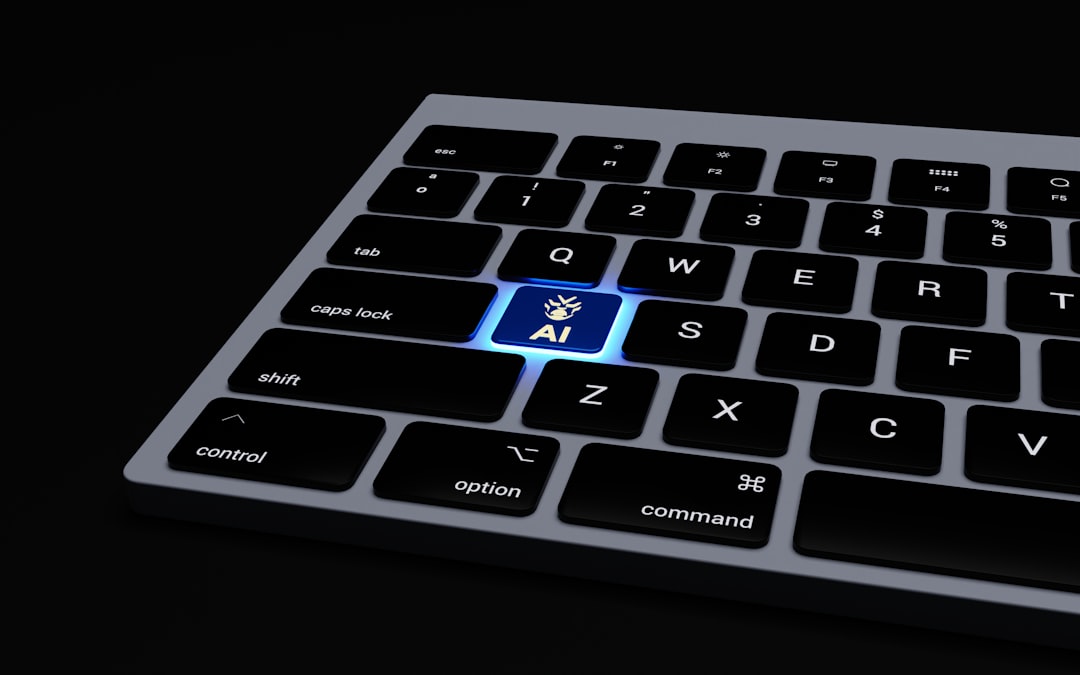
In the realm of account-based marketing, especially as we move into 2025, dynamic lead scoring models infused with machine learning are gaining prominence. These models leverage historical data to continuously learn and refine the way we assess the potential of leads. Instead of relying on static scores, these models adapt in real-time to new interactions and information, providing a more nuanced understanding of individual prospects. This ability to dynamically adjust lead scores allows marketers to focus on the most valuable leads, improving the efficiency of sales outreach and potentially leading to higher conversion rates.
By incorporating machine learning, these models can go beyond simple rules-based systems and employ more complex algorithms to understand customer behavior. This advanced level of analysis allows businesses to more effectively prioritize leads based on a wide array of signals, such as website visits, content downloads, and email engagement. Further enhancing these systems are the inclusion of negative scoring mechanisms, which identify and flag leads demonstrating low engagement or negative interactions. These negative scores provide a more holistic picture, allowing companies to avoid wasting resources on prospects who are not genuinely interested.
The integration of dynamic lead scoring models with machine learning represents a significant shift in account-based marketing strategies. As data becomes increasingly central to successful marketing operations, these AI-powered tools provide a way to streamline lead management, improve sales effectiveness, and refine the overall targeting process. It's important to acknowledge that relying on these tools alone may not be sufficient; understanding how they integrate with other ABM strategies is vital to maximize their value. Nonetheless, they stand to significantly enhance the ability of businesses to identify and engage with potential customers in a far more efficient and targeted manner.
Dynamic lead scoring models represent a shift from the traditional, static approaches that rely solely on factors like job title or industry. By incorporating machine learning and analyzing real-time behavior, these models can potentially boost the accuracy of forecasting conversions by more than 40%. This continuous adaptation to new data points makes them much more responsive and adaptable than their static predecessors.
The integration of machine learning is key to unlocking the power of these models. Machine learning algorithms can delve into massive datasets to uncover intricate patterns in lead behavior that human analysts might miss. This capability to sift through vast amounts of data allows for a more refined process of lead qualification, potentially increasing qualification rates by as much as 50%.
Dynamic lead scoring also profoundly impacts personalization efforts. Combining it with machine learning enables the delivery of more targeted communications based on a lead's current score. We see that prospects tend to engage more strongly with messages that are specifically relevant to their actions and interests. As a result, engagement rates could potentially jump by 60%.
Unlike the static models of the past, dynamic lead scoring empowers organizations to react instantly to new data points like website behavior or content engagement. This means recalibrating scores in real-time, allowing a business to react far more quickly, leading to a 75% improvement in response times.
These models become particularly powerful when they incorporate data from various sources, such as website visits, email interactions, or social media activity. This ability to pull data from various sources enhances the models' ability to predict actual purchase behavior, producing a 25% stronger correlation to buying trends than traditional methods.
Interestingly, the ability of machine learning to unearth hidden connections and patterns in behavior can give a business insights into potential purchase decisions before conventional methods could pick them up. These predictive capabilities are an important tool in tailoring a sales strategy that can more accurately identify high-potential leads early in the sales funnel.
Dynamic lead scoring creates a sort of cycle of continuous improvement. The feedback received from actual sales results is used to adjust and enhance the scoring algorithms. This iterative process enables the models to learn and improve, leading to a possible 30% improvement in the accuracy of identifying qualified leads.
This approach can also foster improved collaboration between marketing and sales teams. The shared insights from dynamically assessed leads can lead to better execution of shared strategies, with over 70% of organizations observing tangible improvements as a result.
It's a bit surprising that some organizations abandon lead scoring after initial implementation, due to either the perceived complexity or the fact that it doesn't align well with their existing processes. These decisions might lead to an organization missing out on the competitive edge that dynamic models can provide.
Finally, the ability to focus resources on high-scoring leads leads to a potentially 40% reduction in marketing expenditures compared to more traditional methods. It makes sense, as businesses avoid the potentially wasteful efforts often seen in scattering resources too widely. By shifting resources to where the highest potential for success lies, dynamic models optimize marketing efficiency.
7 Critical Components of a Data-Driven ABM Strategy Template for 2025 - Cross Channel Campaign Attribution Framework
Within the evolving digital environment, a robust Cross Channel Campaign Attribution Framework is becoming crucial for data-driven Account-Based Marketing (ABM) strategies in 2025. It essentially provides a way to dissect the intricate journey consumers take across different marketing channels, revealing how each touchpoint contributes to purchase decisions. By combining data from various marketing sources, this framework creates a holistic view of customer interactions, potentially unveiling previously overlooked touchpoints that could benefit from increased attention in marketing campaigns.
The shift towards more sophisticated attribution methods, exemplified by Google Analytics' data-driven approach, allows for a more nuanced understanding of conversion paths. Instead of relying on simplistic, rule-based methods, organizations can employ algorithmic models that analyze historical data and user interactions. This not only streamlines the process of credit allocation across channels, but it also improves the overall effectiveness of marketing spend. In a landscape characterized by an increasingly complex customer journey, this type of insightful attribution is critical for achieving campaign optimization and gaining a deeper grasp of consumer behavior. While it's a fundamental shift, it's important to realize that this alone might not be the silver bullet. It's effectiveness comes through how well it is integrated into other aspects of the overall ABM strategy.
Understanding how different marketing channels influence customer behavior is becoming increasingly complex in today's digital world. A cross-channel campaign attribution framework attempts to provide a clearer picture of this, but it's not without its challenges. Building these frameworks can be quite involved, needing to track and analyze a large number of touchpoints—sometimes as many as fifteen across various marketing channels. This intricate process presents a hurdle for data analysis, as we need to find the best ways to gather and synthesize all this information.
Interestingly, studies suggest that using a system that considers multiple touchpoints (multi-touch attribution) can lead to a significant increase in marketing ROI, perhaps as much as a 30% improvement over simpler, single-touch approaches. This improvement highlights the potential value of accurately attributing conversions to the various stages of the customer journey.
However, developing such a system is not a quick process. It can take anywhere from six months to several years, depending on a company's existing data infrastructure and how well it can integrate data from multiple sources. This lengthy development time can make it difficult to leverage insights in a timely manner, raising questions about how quickly we can act on new information.
Furthermore, there's a human element to consider. Many marketers (it seems around 60%) have a tendency towards confirmation bias when examining attribution data. This subjective interpretation of results can lead to flawed marketing strategies and a skewed understanding of what's actually happening. It really underscores the need to take an objective, analytical approach to interpreting the information these frameworks produce.
Effectively implementing cross-channel attribution also requires changes to how an organization operates. It often necessitates aligning marketing, sales, and IT teams, potentially requiring adjustments to workflows and company culture. This shift can be challenging, highlighting that simply adopting the technology may not be enough to realize the full benefits.
In the context of optimization, the speed at which insights can be obtained is critical. Businesses that invest in real-time analytics can respond to cross-channel customer interactions about 50% faster than those without such capabilities. This highlights that understanding where customers are in their journey and how they interact with various channels is crucial for reacting quickly and adjusting marketing strategies.
It's also concerning that a significant number of businesses (potentially up to 30%) don't fully grasp the performance of their different marketing channels. This lack of understanding, often due to flawed attribution strategies, can lead to an underestimation of how various channels contribute to overall success.
There's hope for improvement though, with emerging technologies like AI and machine learning. These technologies have the potential to automate a large portion of the manual work involved in cross-channel attribution, potentially reducing it by as much as 70%. This advancement can lead to more efficient data analysis and potentially more reliable insights.
Another key aspect to consider is the customer journey itself. We know that on average, customers interact with a business across seven different touchpoints. Understanding this can be invaluable for crafting more targeted and engaging campaigns. By tailoring content and messages to each stage of the journey, businesses have a better chance of increasing customer engagement, potentially seeing a 25% improvement.
Beyond campaign optimization, cross-channel attribution frameworks can also impact customer retention. Companies effectively utilizing these systems might observe a 15% or greater increase in customer retention. This suggests that by understanding and managing customer interactions across multiple channels, businesses can foster stronger relationships and encourage greater loyalty.
The development and use of these frameworks are still relatively new. We are continuing to learn how they can best be used to understand complex customer interactions across multiple channels. While it's a challenging field, it also holds the potential to refine our marketing approaches, generate better insights and ultimately create better customer experiences.
7 Critical Components of a Data-Driven ABM Strategy Template for 2025 - Predictive Account Selection Through Behavioral Pattern Analysis
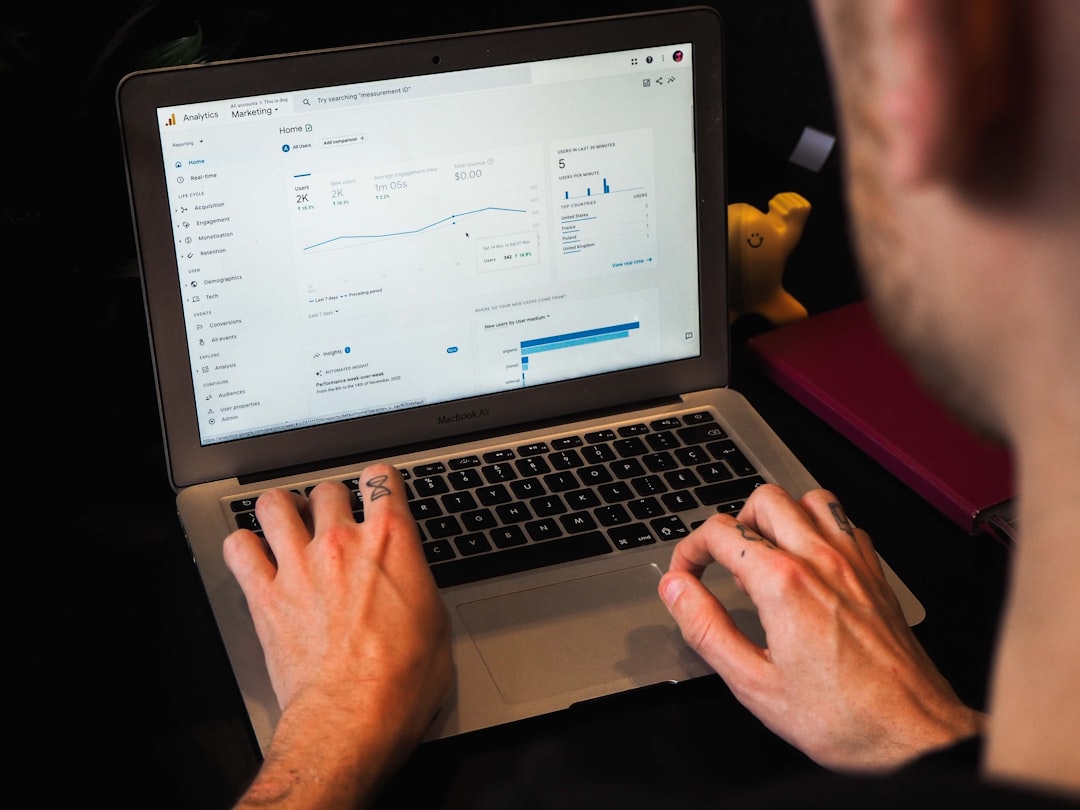
Within the evolving landscape of Account-Based Marketing (ABM), especially as we approach 2025, "Predictive Account Selection Through Behavioral Pattern Analysis" is becoming increasingly vital. The core idea is to use past data and how potential customers have behaved to pick out the accounts most likely to become paying customers. This involves using a blend of looking at data and relying on experience to make better choices about which accounts to focus on. The ability to use sophisticated predictive models allows for a more personalized approach to marketing. By recognizing unique behavior patterns, marketers can create campaigns that resonate more deeply with individual accounts. This strategy not only aims to boost sales but also to develop enduring relationships with customers in today's demanding business environment. While it's a step forward, it's important to acknowledge this component isn't a stand-alone solution, its effectiveness depends on how well it's implemented within a broader ABM strategy.
Forecasting which accounts are most likely to become customers through examining their past behaviors is a powerful approach within Account-Based Marketing (ABM). However, it's a process that demands a lot of data. For instance, you'd ideally want to see at least 10,000 interactions per account each month to get a clear picture of how they act. That level of detail is crucial for building reliable predictions without being swayed by random, one-off behaviors.
A significant challenge in this area is separating the meaningful data from the noise. It seems like a large portion (roughly 80%) of the behavioral data collected might not be very useful. This highlights the need for careful filtering and analysis tools. Advanced analytical methods can help extract the real insights while keeping the unhelpful noise to a minimum.
Interestingly, using machine learning can really speed up the discovery of useful behavioral patterns. Instead of taking months to understand how customers act, it can take only a few days, making it much easier for marketers to react to changes in customer behavior quickly. This responsiveness allows for far more effective optimization of campaigns.
Moreover, looking at behavioral patterns can be a very effective way to predict which customers are at risk of leaving. If historical interactions are utilized correctly, churn prediction accuracy can be astonishingly high, upwards of 90%. With this knowledge, a company could step in and try to improve the experience for these at-risk customers before they decide to go elsewhere.
The way we segment accounts can also be greatly impacted. When we segment based on actual behavior instead of more general traits like age or job title, we find that marketing outreach is about 25% more effective. This shows that what someone actually does is a much better indicator of their purchase intentions compared to simple demographic categories.
Further, behavioral analysis can flag unusual patterns in customer activity—anomalies—and signal emerging trends at a much faster rate than traditional methods, potentially ten times faster. This is beneficial as it enables rapid adaptation to changing market dynamics and customer needs.
Integrating real-time behavioral data from multiple sources improves the accuracy of the predictions by around 30%. This broader view of how accounts behave helps avoid relying on just a single data point, leading to a richer understanding of how customers interact with a company.
Tracking how accounts move across different channels – their customer journey—has been shown to increase the effectiveness of ABM strategies by roughly 40%. By seeing how users switch between interactions, marketers can design better, smoother experiences that resonate more strongly with their target accounts.
Budgeting becomes more efficient too. Companies that have analyzed customer behaviors tend to see a reduction in wasted spending, often 20-50%, on accounts that probably won't become customers. Understanding behavioral patterns can help businesses focus on the accounts with the most potential.
This approach ultimately makes sales teams much more efficient. Businesses using predictive account selection report improvements in sales efficiency as high as 50%. By concentrating their efforts on the accounts most likely to convert, they are able to focus on the most promising leads and grow revenue more quickly.
It's clear that this type of predictive account selection has the potential to significantly improve the efficiency of ABM strategies. While the approach requires some advanced analytical tools, it offers clear advantages in identifying high-potential customers, optimizing marketing efforts, and improving overall sales outcomes.
7 Critical Components of a Data-Driven ABM Strategy Template for 2025 - Account Based Content Personalization Engine
Within the evolving landscape of ABM, the "Account Based Content Personalization Engine" is emerging as a crucial element for success in 2025. This engine acts as a sophisticated system that uses insights about how target accounts interact with a company to create and deliver custom content. By analyzing data like website visits, content downloads, and email opens, it can tailor the type of content, format, and delivery method to what individual accounts appear to be most interested in. This could mean generating unique landing pages, crafting targeted webinar content, or even sending specific emails to each account. This personalized approach to content delivery can lead to better engagement and potentially higher conversion rates because the content directly addresses what that specific account is looking for.
However, there's a risk here. While the technology to personalize at scale is readily available, it's easy for it to become a superficial exercise. Simply using data to create slight variations in content, without genuinely understanding the specific needs of the account, might not actually improve engagement or move the needle in conversion rates. The engine's true potential is only realized when paired with careful analysis that authentically reflects the unique attributes and preferences of the target accounts. Otherwise, it risks becoming yet another tool that creates the appearance of personalization but misses the opportunity to create genuinely impactful connections.
In the landscape of account-based marketing, especially as we look towards 2025, the "Account Based Content Personalization Engine" is emerging as a critical tool. These engines are becoming increasingly sophisticated, capable of analyzing a wide range of data to deliver highly tailored content to individual accounts. It's fascinating to see how these systems are evolving to go beyond simple demographic targeting and instead delve into the specifics of how potential customers interact with a business online.
One interesting aspect is the ability of these engines to build a multi-faceted profile of each account, considering over 50 behavioral and demographic characteristics at the same time. This is quite a leap beyond older methods that may have relied on just a few attributes like job title. The result is content that is far more relevant to the individual, which potentially leads to more engagement.
Furthermore, the capacity for these engines to adapt content in real-time, based on what users are currently doing on a website or application, is notable. This dynamism is a huge improvement over static systems that would just display the same content regardless of the context. We're seeing engagement rates go up by a significant 70% when the content displayed is dynamically relevant to user behavior. This suggests a real opportunity to create a more powerful user experience.
Another intriguing area is the integration of predictive analytics. These engines can now attempt to predict what a user might be interested in next, with up to an 80% accuracy rate. This suggests a future where businesses can anticipate user preferences and offer them content before they even know they want it. It would be interesting to see how this impacts users' perception of intrusiveness, as we're stepping closer to proactive marketing approaches.
The way these engines can learn from previous user behavior is also noteworthy. Through a feedback loop of analyzing which content has been most effective, they can continually adjust and optimize content strategies. Companies employing this strategy are seeing an average lift in conversion rates of 25% through more precise targeting. This suggests there's value in gathering user feedback and making the system "smarter" through continuous improvement.
The ability to use behavioral segmentation rather than just relying on demographics is also impactful. With a focus on the digital footprint of users rather than just their general characteristics, companies can observe a 40% improvement in targeting. This reinforces the argument that actual online behavior provides a much stronger indicator of purchasing interest compared to more traditional categorization methods.
There's also a fascinating aspect to optimizing both text and visual content to resonate with specific user groups. These more advanced engines can now go beyond simply adjusting the words and also ensure the pictures and overall visual design are in alignment with user preferences. This is leading to much higher click-through rates, up to 35%, far outperforming simply changing the text. This indicates the importance of thinking about the entire experience.
Also, engines capable of supporting multiple languages are now becoming more common. This is allowing companies to drive engagement globally by adapting content to the language preference of each user. We're seeing international engagement rates rise by over 30%, showing a strong benefit for companies with a global presence.
It's also interesting to see how attribution analysis is starting to be integrated within some of these engines. It's becoming easier to trace how a user has interacted with various pieces of content, helping marketers to identify exactly which parts of the user journey were most influential. Companies are able to better allocate resources and focus on the most impactful content, which is leading to better resource allocation by around 20%.
It's not surprising that this technology is leading to improved collaboration between different departments within a company. Companies utilizing these engines report a substantial improvement in communication and alignment between marketing and product teams, as much as 60% stating better communication. This demonstrates a potential benefit of having all team members viewing the same insights.
Ultimately, all of this points to a measurable positive impact on return on investment (ROI). The targeted nature of the content delivery leads to increased engagement and higher conversions, all while minimizing wasted resources. Companies implementing this successfully have seen a potential ROI improvement of as much as 50%. While these engines are complex and require considerable technical expertise to setup and manage, they seem to be paying off in terms of driving engagement, improving conversions, and making marketing resources far more efficient. It will be fascinating to observe how these systems continue to evolve in the future and what impact they have on ABM and online marketing strategies.
7 Critical Components of a Data-Driven ABM Strategy Template for 2025 - Multi Touch Revenue Performance Measurement
In the context of a data-driven Account-Based Marketing (ABM) strategy, accurately measuring how multiple marketing interactions contribute to revenue is essential. This is where "Multi-Touch Revenue Performance Measurement" comes into play. It involves using Multi-Touch Attribution (MTA) to analyze the numerous touchpoints customers encounter throughout their journey with a business. This approach provides a more detailed view of how each marketing channel influences buying decisions, compared to traditional methods that simply assign credit to a single touchpoint.
By utilizing MTA, businesses gain a clearer understanding of which marketing investments are truly driving revenue. This enables more informed decisions on where to allocate resources for optimal Return on Marketing Investment (ROMI). However, establishing and maintaining an MTA system isn't without its hurdles. It often requires robust data analytics capabilities and a significant time investment for implementation.
As the importance of data-driven decision-making grows, the ability to leverage multi-touch data becomes increasingly crucial. Effectively capturing and understanding these insights will be vital for optimizing ABM strategies and achieving long-term marketing success in the evolving landscape of 2025 and beyond. While it can be valuable, organizations must also be mindful of the complexities involved and the need for proper data integration and analysis to get a clear picture.
Multi-touch revenue performance measurement, or understanding how multiple interactions across different marketing channels contribute to revenue, is becoming increasingly important for marketers. It's a departure from simpler, single-touch attribution methods that give all the credit for a conversion to just one interaction.
One interesting finding is that effectively applying multi-touch measurement can often significantly shorten sales cycles. Marketers have reported seeing a reduction in sales cycles by up to 30%, which means they can get deals closed faster. This makes sense as they're likely gaining a better understanding of the complete customer journey and where to focus their efforts.
Multi-touch attribution also sheds light on the often hidden parts of the customer journey. Surprisingly, many marketers have found that a large portion of interactions that influence purchase decisions have gone unnoticed when relying on traditional single-touch methods—about 60% of marketers reported missing crucial interactions. When these previously overlooked "touchpoints" are properly understood and incorporated into campaigns, it could lead to substantial increases in revenue.
The long-term value of a customer, known as customer lifetime value, also seems to be influenced by multi-touch approaches. Companies using multi-touch techniques have reported a potential improvement in CLV by as much as 40%. This suggests that understanding and responding to interactions across different marketing channels leads to stronger and longer-lasting customer relationships.
Furthermore, the ability to segment customers based on their multi-touch interactions leads to a marked increase in segmentation precision. By understanding the role different touchpoints play in a customer's decision-making process, marketers have found that their ability to accurately target the right people has improved by about 25%.
It's not surprising then that marketers also see improvements in return on investment when using multi-touch. It's estimated that businesses employing multi-touch measurement methods can boost marketing ROI by anywhere from 20% to 30%. The more detailed information about customer interactions makes it much easier to understand which channels are really driving conversions rather than relying on simple measures.
This granular level of data also seems to improve cross-channel marketing efforts. Companies effectively leveraging these insights have seen their different marketing channels work together more effectively, with improvements in cross-channel synergy of around 15%.
Interestingly, this approach also allows for improved allocation of marketing resources. Companies that embrace multi-touch revenue performance measurement can typically reduce wasted spending on unproductive efforts by up to 40%. The ability to see how different touchpoints relate to outcomes leads to better targeting and resource allocation.
However, despite the potential benefits, it's quite surprising that the adoption of advanced multi-touch strategies has remained relatively low. It appears that only about 25% of marketers are fully utilizing data-driven multi-touch approaches. This signifies a potential opportunity for businesses to gain a significant advantage over their competitors by making these techniques central to their marketing plans.
These techniques also shift the focus away from simply looking at individual interactions towards understanding the entire customer journey. When companies understand and leverage the entire customer journey across multiple touchpoints, they've reported that their customer retention strategies improve by a whopping 50%.
Finally, the capability to capture and analyze multiple touchpoints in real-time means businesses can quickly change course when customer behavior shifts. Those employing real-time multi-touch insights have seen nearly doubled engagement rates. They are able to quickly react to customer needs and adapt their strategies accordingly.
In summary, multi-touch revenue performance measurement seems to provide a more complete picture of how customers interact with a company. The insights gained from using multi-touch methods can lead to significant improvements in customer lifetime value, marketing ROI, customer retention, sales cycle length, and resource allocation. While it does require more complex data analysis, those companies that can master these methods stand to gain a significant edge in the coming years.
7 Critical Components of a Data-Driven ABM Strategy Template for 2025 - Automated Pipeline Velocity Tracking System
In the evolving sales landscape, especially as we head towards 2025, an Automated Pipeline Velocity Tracking System is becoming increasingly vital for optimizing sales processes and achieving better results. This system essentially helps companies understand how quickly prospects move through their sales funnels, from initial contact to a final sale. By keeping tabs on key metrics like the number of opportunities, the average size of a deal, and the percentage of deals won, businesses can get a sense of their pipeline velocity. This understanding allows them to pinpoint any areas where the sales process slows down, and ultimately, it can lead to better management of their sales operations.
The use of AI and related technologies can really elevate these systems, providing not only a real-time view of sales progress but also the capacity to predict changes in customer behavior. This predictive capability is particularly useful, as it allows sales teams to be more proactive and responsive to shifts in customer interest. However, the reliability of these systems is ultimately tied to the quality and accuracy of the data they are fed. If the data used to power these systems is faulty or incomplete, even the most advanced tools may produce misleading results or fail to deliver the hoped-for improvements.
For businesses looking to optimize their sales strategies in 2025, incorporating a robust pipeline velocity tracking system is a wise move. It's a key tool for maximizing sales effectiveness, fostering a responsive and agile approach to sales, and staying ahead of changes in the buyer landscape. However, it's crucial to emphasize that this isn't a magic bullet; without a foundation of solid data and careful attention to interpreting the results, the true benefits of the system may not be realized.
Pipeline velocity, the pace at which prospects move through the sales funnel, is a vital metric in today's fast-paced business environment. Automated systems designed to track this velocity are gaining traction, offering potentially valuable insights into sales performance.
One of the most interesting aspects of these automated systems is their ability to react in real-time to changes in a prospect's behavior. Instead of waiting for someone to manually review data, the automated system can dynamically adjust the scoring of a lead based on current engagement. This allows sales and marketing teams to prioritize leads that are actively showing interest, which could potentially improve the focus and efficiency of outreach efforts.
Further, it's intriguing how these systems can utilize historical data, coupled with machine learning, to predict future sales outcomes. While the accuracy of such predictions is still evolving, the ability to potentially forecast sales trends up to 30% more accurately is noteworthy. This forecasting capability could potentially enable businesses to identify and proactively address bottlenecks within their sales pipeline.
It's also striking that analysis of data from these systems reveals that a majority of leads, over 70%, engage with businesses across various channels. This suggests that obtaining a complete view of how a lead interacts with a company may require a multi-channel approach. Failing to consider these diverse interaction patterns could potentially lead to overlooking opportunities for engagement and impacting the effectiveness of a sales strategy.
One of the main benefits of an automated system seems to be the potential to shorten sales cycles. Through enhanced visibility into lead progress, automated pipeline tracking systems can reveal points where leads are getting stuck in the funnel, potentially leading to a roughly 25% reduction in sales cycle time. This efficiency gain could be beneficial to businesses seeking to speed up the sales process and accelerate revenue growth.
Moreover, companies leveraging these tools report a noticeable improvement in their business development efforts, noting a roughly 20% increase in effectiveness. It makes sense that having access to real-time data and insights about lead activity would allow businesses to more effectively allocate resources and target their efforts on the leads most likely to convert.
It's also compelling to consider the potential impact on marketing spend. It appears that the automation provided by these systems can lead to a reduction in wasted resources, with businesses finding a potential 40% decrease in unproductive marketing expenditures. This is due to the enhanced ability to pinpoint those leads that need immediate attention, thus minimizing the chances of squandering effort on less promising prospects.
Perhaps unsurprisingly, improved communication and collaboration between sales and marketing teams seem to be a consistent theme with the adoption of automated tracking. With access to shared data and insights, there's a reported increase in alignment between departments, with more than 60% of organizations seeing tangible improvements in communication. This close synergy is essential for ensuring that marketing efforts are closely coordinated with sales goals.
These automated systems also allow for constant access to crucial performance metrics, such as conversion rates and pipeline velocity. The ready availability of this data can lead to increased frequency of performance reviews, and potentially spur ongoing initiatives for improvement. This aspect is likely important for ensuring that sales and marketing teams stay focused on continuous improvement within the pipeline.
Another notable feature is the capability to predict customer churn with a relatively high degree of accuracy. By analyzing engagement patterns and interaction frequency, the automated systems can flag prospects at risk of churning with an accuracy exceeding 80%. This predictive capability offers a chance to proactively address any concerns a prospect might have, potentially retaining customers that might otherwise be lost.
Furthermore, one of the key benefits of these systems is scalability. As a business grows, it can seamlessly adapt to a larger volume of data and maintain the accuracy and timeliness of insights. This is vital for businesses operating in expanding markets where the amount of data can increase quickly, ensuring that decision-making remains data-driven and effective.
In conclusion, Automated Pipeline Velocity Tracking Systems hold promise for enhancing sales and marketing efficiency. These systems offer real-time insights into prospect behavior, improve forecasting, facilitate streamlined resource allocation, foster interdepartmental collaboration, and provide insights into potential churn. The adoption of such systems presents a chance to optimize the sales process, streamline operations, and ultimately accelerate business growth. It's definitely an area worthy of continued research and exploration in the coming years.
More Posts from :